Machine learning for flow-informed aerodynamic control in turbulent wind conditions
Communications Engineering(2022)
摘要
Control of aerodynamic forces in gusty, turbulent conditions is critical for the safety and performance of technologies such as unmanned aerial vehicles and wind turbines. The presence and severity of extreme flow conditions are difficult to predict, and explicit modeling of fluid dynamics for control is not feasible in real time. Model-free reinforcement learning methods present an end-to-end control solution for nonlinear systems as they require no prior knowledge, can easily integrate different types of measurements, and can adapt to varying conditions through interaction. Here, we show that reinforcement learning methods can achieve effective aerodynamic control in a highly turbulent environment. Algorithms are trained with different neural network structures, and we find that reinforcement learning agents with recurrent neural networks can effectively learn the nonlinear dynamics involved in turbulent flows and strongly outperform conventional linear control techniques. We also find that augmenting state observations with measurements from a set of bioinspired flow sensors can improve learning stability and control performance in aerodynamic systems. These results can serve to inform future gust mitigation systems for unmanned aerial vehicles and wind turbines, enabling operation in previously prohibitively dangerous conditions.
更多查看译文
关键词
Aerospace engineering,Computer science,Engineering,general
AI 理解论文
溯源树
样例
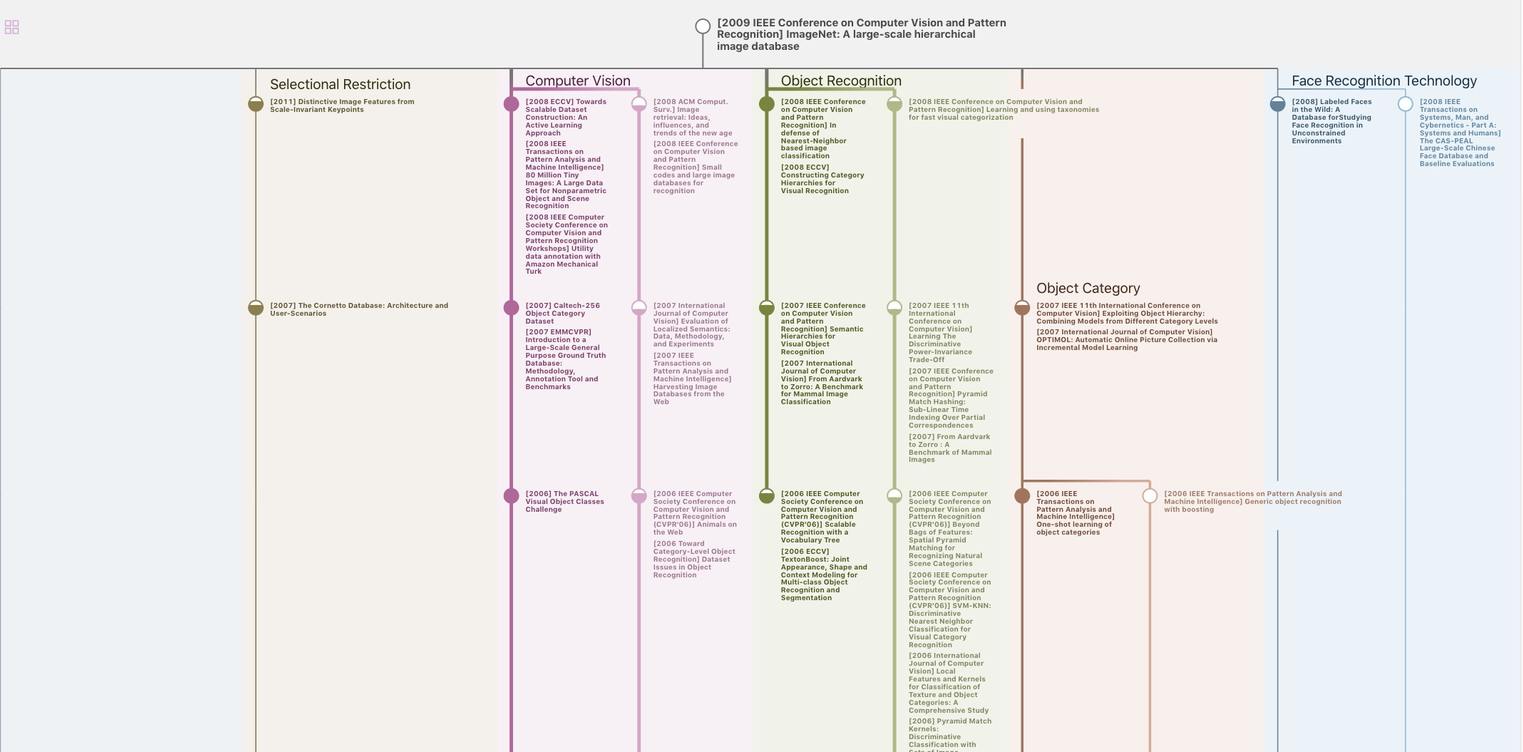
生成溯源树,研究论文发展脉络
Chat Paper
正在生成论文摘要