Go Closer to See Better: Camouflaged Object Detection via Object Area Amplification and Figure-Ground Conversion
IEEE Transactions on Circuits and Systems for Video Technology(2023)
摘要
Camouflaged Object Detection (COD) aims to detect objects well hidden in the environment. The main challenges of COD come from the high degree of texture and color overlapping between the objects and their surroundings. Inspired by that humans tend to go closer to the object and magnify it to recognize ambiguous objects more clearly, we propose a novel three-stage architecture called Search-Amplify-Recognize and design a network SARNet to address the challenges. Specifically, In the Search part, we utilize an attention-based backbone to locate the object. In the Amplify part, to obtain rich searched features and fine segmentation, we design Object Area Amplification modules (OAA) to perform cross-level and adjacent-level feature fusion and amplifying operations on feature maps. Besides, the OAA can be regarded as a simple and effective plug-in module to integrate and amplify the feature maps. The main components of the Recognize part are the Figure-Ground Conversion modules (FGC). The FGC modules alternately pay attention to the foreground and background to precisely separate the highly similar foreground and background. Extensive experiments on benchmark datasets show that our model outperforms other SOTA methods not only on COD tasks but also in COD downstream tasks, such as polyp segmentation and video camouflaged object detection. Source codes will be available at
https://github.com/Haozhe-Xing/SARNet
.
更多查看译文
关键词
object detection,object area amplification,figure-ground
AI 理解论文
溯源树
样例
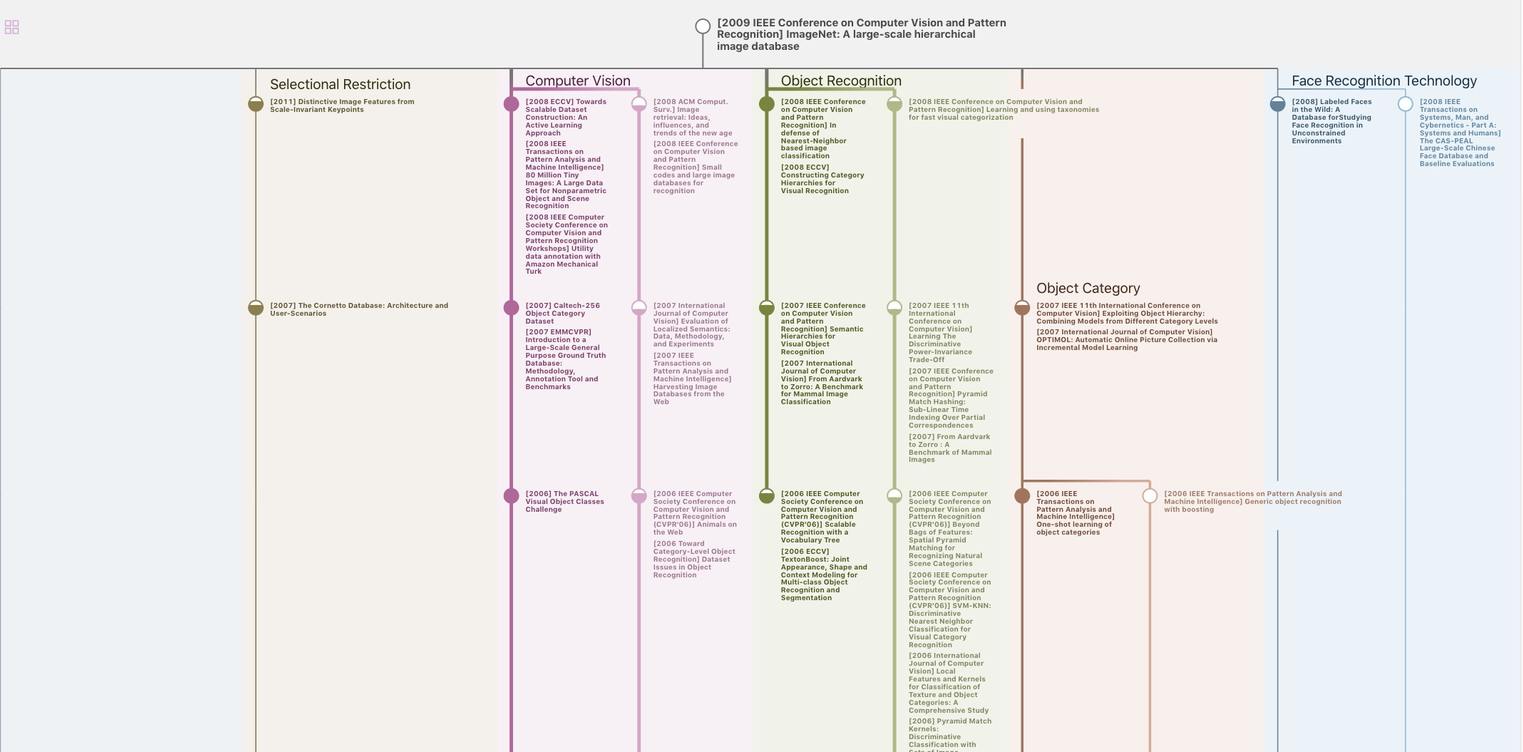
生成溯源树,研究论文发展脉络
Chat Paper
正在生成论文摘要