Multiscale Bayesian PCA for robust process modeling of a Fischer-Tropsch bench scale process
CHEMOMETRICS AND INTELLIGENT LABORATORY SYSTEMS(2023)
摘要
Building a good process model is critical for process monitoring technologies which ensure process safety, reliability, and profitability. In this work, we develop a Multiscale Bayesian PCA (MS-BPCA) algorithm and use it to model a real Fischer-Tropsch (FT) pilot plant. We demonstrate the algorithm's superior and robust performance in dealing with autocorrelation and noise contamination compared to BPCA and Multiscale PCA. The modeling performance of BPCA depends on the accuracy of the likelihood and prior parameters. Therefore, we explore various techniques (multiscale filtering, polynomial approximation, and PCA) to empirically estimate these parameters. Furthermore, we study the limitations of the BPCA modeling algorithm when its assumptions of gaussian data and independent noise are violated. Finally, this work will compare the modeling performance of all techniques (PCA, Bayesian PCA, Multiscale PCA, and Multiscale Bayesian PCA) with real data collected from a state-of-the-art bench-scale Fischer-Tropsch (FT) process.
更多查看译文
关键词
Bayesian PCA,Data-driven modeling,Fischer-Tropsch process
AI 理解论文
溯源树
样例
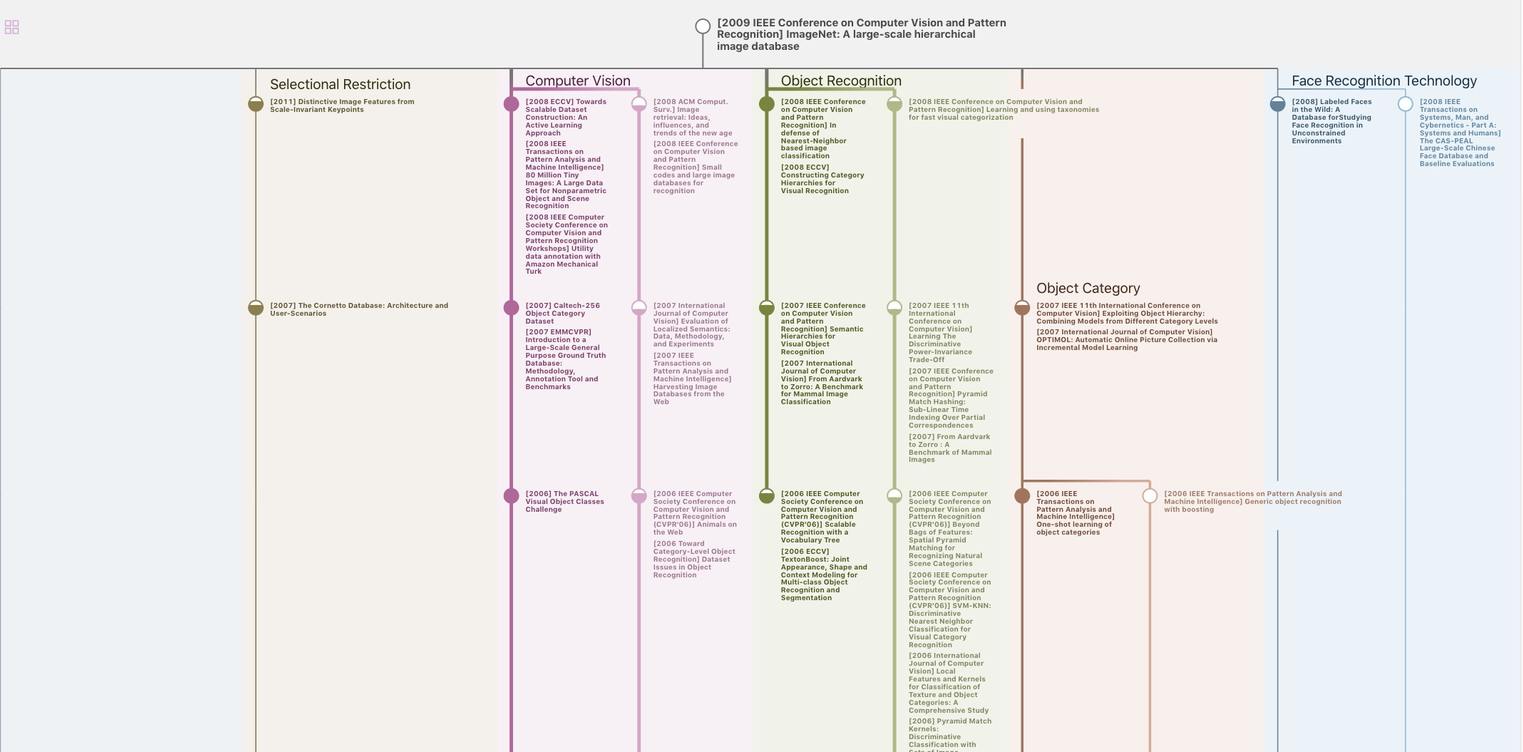
生成溯源树,研究论文发展脉络
Chat Paper
正在生成论文摘要