A Screening Model for the Prediction of Early Onset Parkinson’s Disease from Speech Features
Advances in Cognitive Science and CommunicationsCognitive Science and Technology(2023)
摘要
Parkinson's disease is a progressive neurodegenerative condition that is characterized by a variety of motor and non-motor disorders. Lack of movement, uncontrolled shaking of the hands, jaw, arms, legs, tongue, or hands are examples of motor symptoms. Non-motor symptoms include low blood pressure, sleep, pain, fatigue, skin and sweating, or restless legs. Clinical studies and assessment of associated symptoms, along with the characterization of a range of motor symptoms, are often used to diagnose Parkinson's disease. Traditional diagnostic procedures, on the other hand, may be vulnerable to diagnostic bias because they based on the assessment of motions that are usually delicate to human sight and hence hard to make early predictions. Patient therapy is complicated by the varied nature of Parkinson's disease symptoms and there is a variability in the progress. These symptoms are frequently missed, making early Parkinson’s disease diagnosis is difficult. There are inadequate comparative data to suggest a specific treatment course in either early or advanced PD with motor difficulties. As a result, machine learning technologies have been used to overcome these issues and there is a great deal of interest in building models that recommend the treatment for Parkinson's disease. A preliminary study is conducted to characterize the features of importance. We then employ machine learning models to the speech dataset in order to derive insights on the diagnosis. The main objectives of this work are to propose the stack ensemble algorithm as a promising screening model for the early onset PD diagnosis. The algorithm exhibits 97% accuracy when compared to other models under evaluation.
更多查看译文
关键词
early onset parkinsons,parkinsons disease,speech features,screening model
AI 理解论文
溯源树
样例
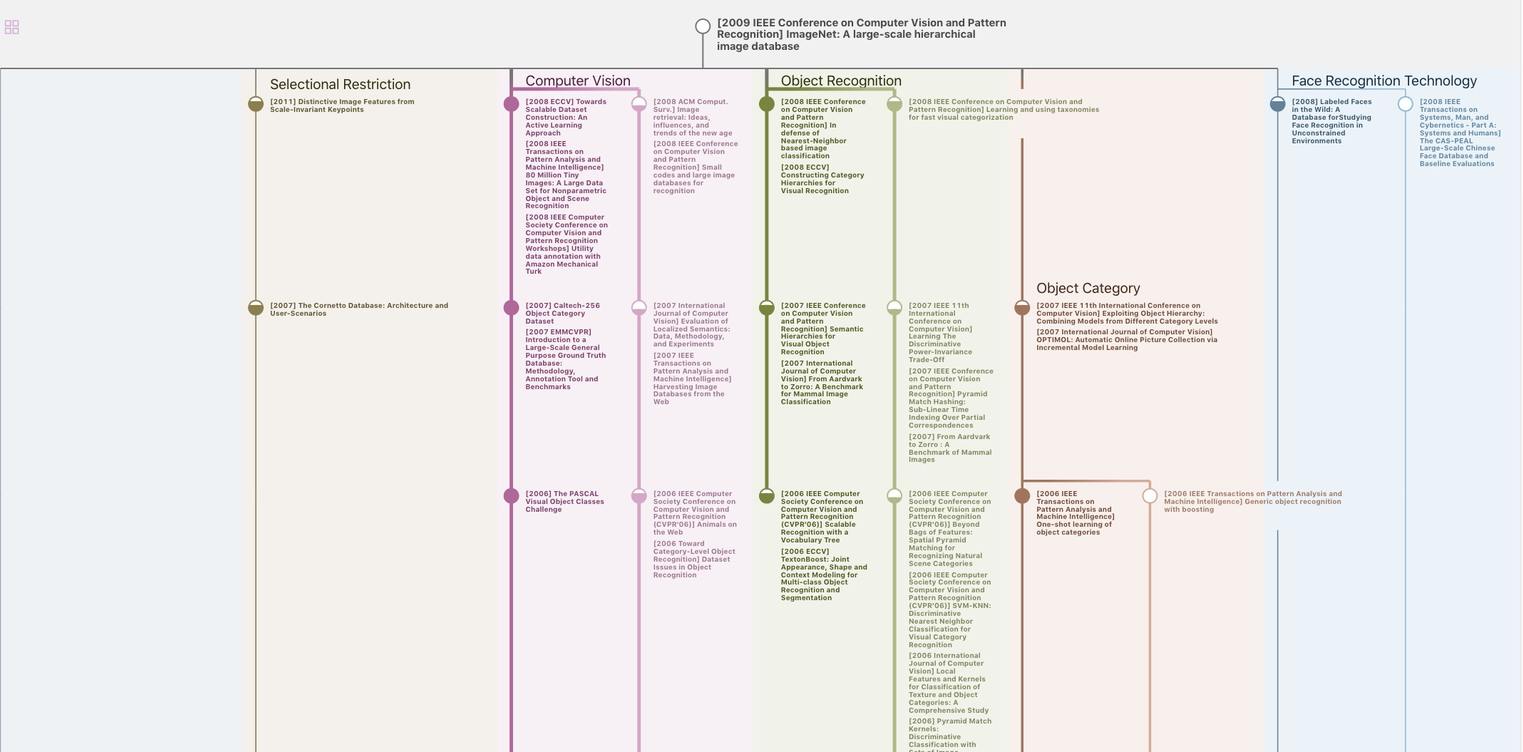
生成溯源树,研究论文发展脉络
Chat Paper
正在生成论文摘要