MSR89 Evaluating Prediction Model Calibration at the Moderate-Strong Level Using Patient Subgroups Identified With Clustering Analysis
Value in Health(2022)
摘要
Prediction models with poor calibration can be misleading and may result in incorrect and potentially harmful decisions. Calibration was deemed as the Achilles heel of prediction models, and the importance of model calibration has received more attention in recent years. Van Calster et al. defined a hierarchy of four increasingly strict levels of calibration: mean, weak, moderate, and strong calibration. They argued that although strong calibration is desirable for individualized decision making, it is unrealistic in practice. Thus moderate calibration is a better attainable goal and can still guarantee that decisions made based on the model are clinically nonharmful. In a recent concept paper by the GRADE working group, the authors suggested to evaluate calibration for subgroups defined either with different risk categories (e.g., lower or higher risk) or based on characteristics not included in the model. In this conceptual paper, we propose a new calibration level, namely moderate-strong level, as an extension to the hierarchy proposed by Van Calster et al. The moderate-strong calibration is evaluated for subgroups identified with clustering analysis of all predictors included in the model. It can provide better assurance than moderate calibration and is still realistic for evaluation, and it is more meaningful than the subgroups proposed by GRADE. The proposed methods are presented with an illustrative case study using T1D Exchange data to validate a prognostic model for type 1 diabetes patients, to demonstrate how subgroups can be identified with clustering analysis and how calibration at moderate-strong level can be evaluated with different numbers of subgroups. This illustrative example shows the potential application of clustering analysis in creating credible and meaningful subgroups, without collecting extra data than model validation, to approximate the strong calibration.
更多查看译文
关键词
patient subgroups,clustering analysis,prediction,moderate-strong
AI 理解论文
溯源树
样例
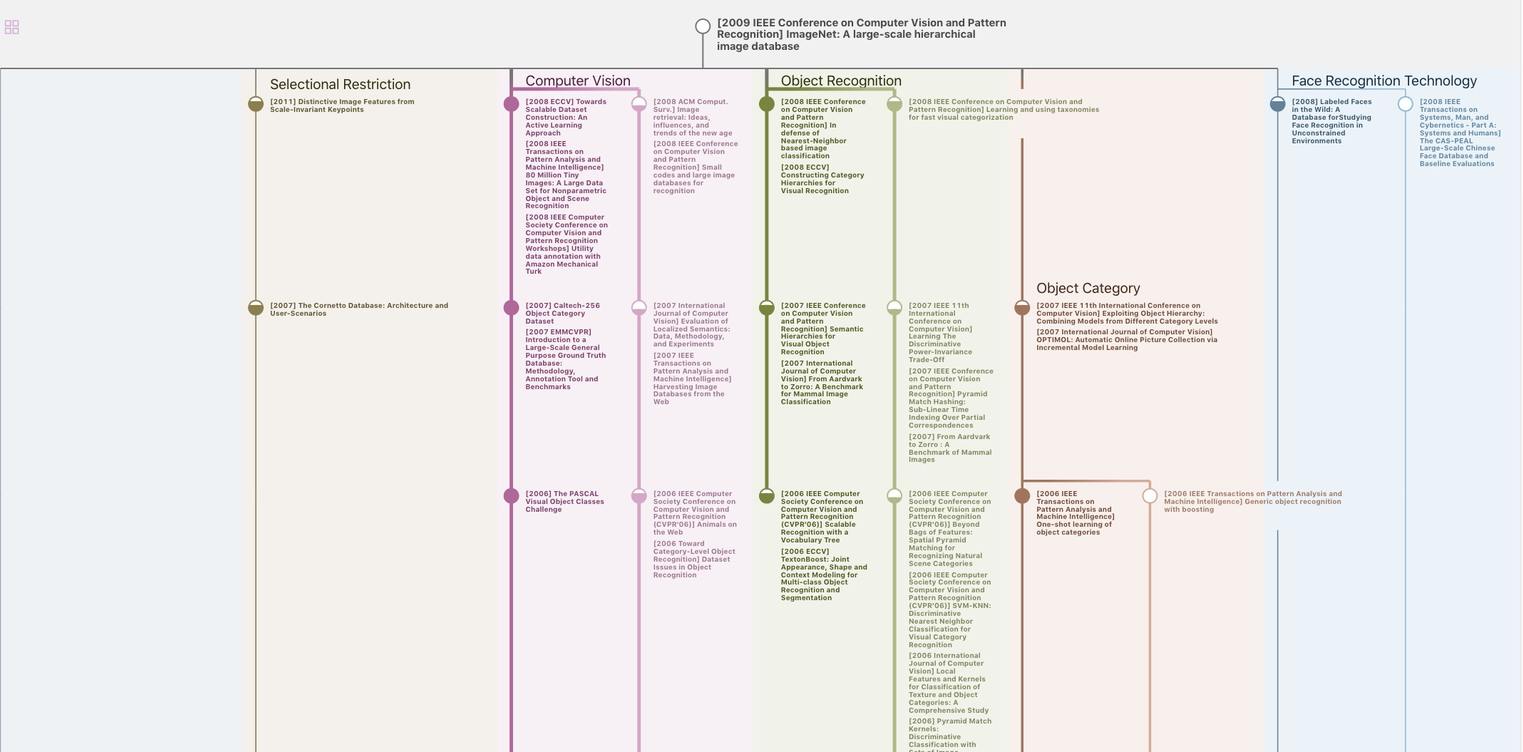
生成溯源树,研究论文发展脉络
Chat Paper
正在生成论文摘要