Individuals with anxiety and depression use atypical decision strategies in an uncertain world
crossref(2023)
摘要
Previous studies have identified that enhanced learning rate is a key mechanism for adapting to fast-changing reward environments with high volatility, and that impairments in flexible learning rate may be associated with several psychiatric conditions. However, these studies have mostly assumed a single strategy manifested in the human probabilistic learning process. In contrast, we propose an alternative perspective and develop a hybrid mixture-of-strategy (MOS) model that incorporates three strategies: the expected utility strategy, which aims to maximize rewards; the magnitude-oriented strategy, which only considers reward magnitude; and the habitual strategy, which tends to repeat previous decisions. While the expected utility strategy is statistically optimal, the magnitude-oriented and habitual strategies are suboptimal but computationally simpler. In an open dataset where healthy controls and patients with anxiety and depression performed a probabilistic reversal learning task with distinct volatility conditions, our MOS model outperforms previous best-fitting models. Parameter analyses suggest that individuals with anxiety and depression exhibit weaker preferences for the optimal expected utility strategy but stronger preferences for the magnitude-oriented heuristic. The relative strength of these two strategies also predicts individual variation in symptom severity. Importantly, our MOS model explains the slower learning in patients by the different weighting of the different strategies rather than learning rate per se. These findings underscore the complexity of human learning and decision-making and suggest the possibility of mixed strategies in learning and decision-making. Further research is necessary to distinguish the flexible-learning-rate account from the mixture-of-strategy account.
更多查看译文
AI 理解论文
溯源树
样例
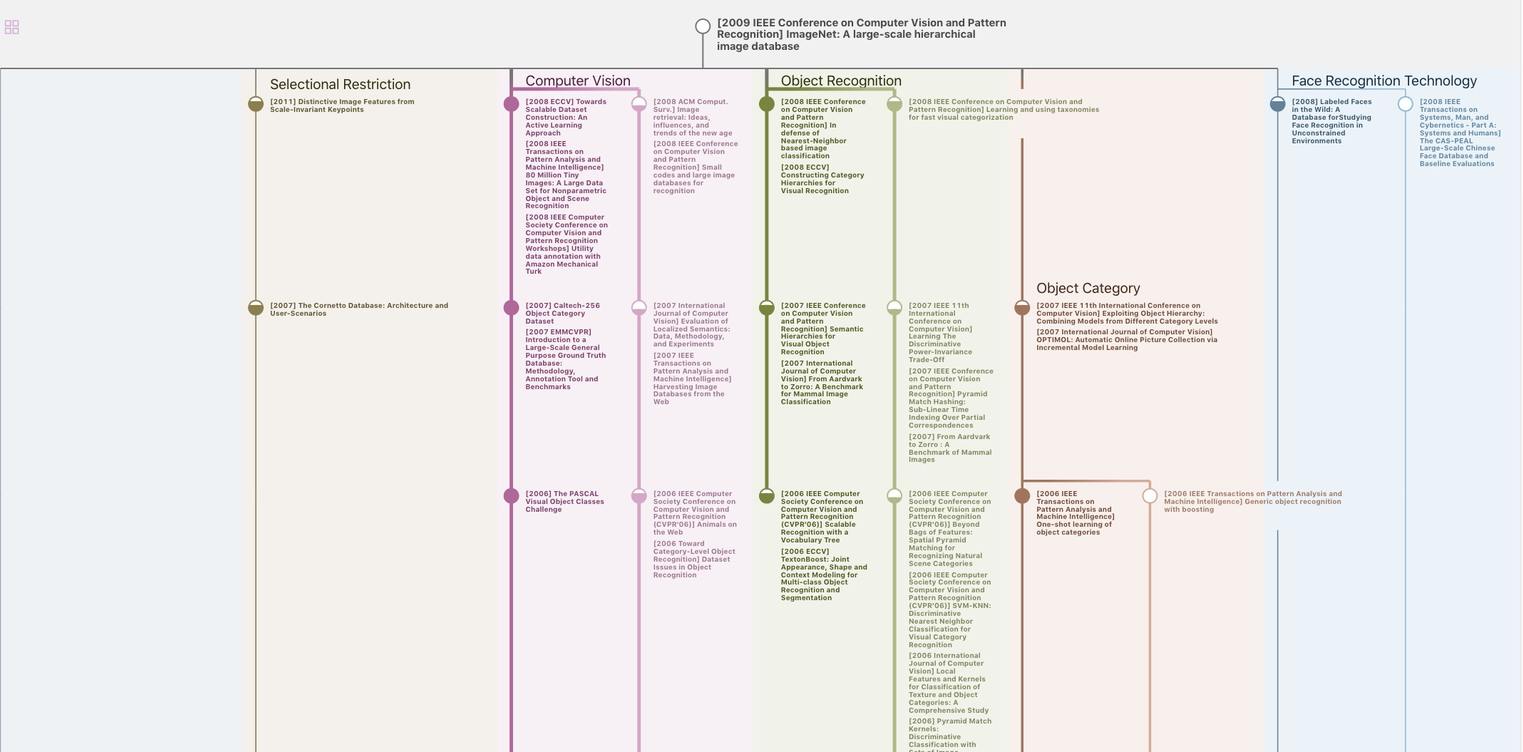
生成溯源树,研究论文发展脉络
Chat Paper
正在生成论文摘要