A deep-learning approach for scar detection in early contrast-enhanced cardiac computed tomography images
European Heart Journal Supplements(2023)
摘要
Abstract Diagnosis of myocardial fibrosis has a relevant prognostic and therapeutic role. Scar-tissue analysis is commonly performed with late gadolinium contrast-enhanced (CE) cardiac magnetic resonance (CMR). However, CMR might be contraindicated or unavailable. Coronary computed tomography (CCT), which is the technique of choice for many routine assessments, is emerging as an alternative to CMR. The objective of this study is to evaluate whether artificial intelligence (AI) could allow identification of myocardial fibrosis from routine early CE-CCT images. Fifty consecutive patients with left ventricular dysfunction (LVD), who underwent both CE-CMR and (early and late) CE-CCT were retrospectively selected. According to the late enhancement CMR patterns, patients were classified as with ischemic (n=15, 30%) or non-ischemic (n=25, 70%) LVD. Scar regions were manually traced on late CE-CCT using CE-CMR as ground-truth. On early CE-CCT images, the myocardial sectors were extracted according to AHA 16-segment model and labeled as with scar or not based on the late CE-CCT manual tracing. A deep-learning model was developed to classify each sector (Picture). Of the initial 44187 sectors computed out of the 8285 slices available from the early CE-CCT images, 4594 sectors (10%) presented scar. The CNN approach on the early CE-CCT images yielded a classification accuracy for all sectors of 71% (95% confidence interval (CI): 63%-79%) obtained through 5-fold cross validation. The mean sensitivity, positive predictive value (PPV) and negative predictive value (NPV) for the testing fold resulted in 73% (95% CI: 66%-79%), 56% (95% CI: 48%-65%) and 85% (95% CI: 82%- 88%), respectively. The mean AUC across the five folds was 76% (95% CI: 72%-81%). In a per-segment analysis of the 16-segment AHA model the bull's eye segmental comparison of CE-CMR and respective early CE-CCT findings an 91% agreement was achieved. Artificial intelligence can detect both ischemic and non-ischemic myocardial fibrosis from routine noninvasive coronary scans, without additional contrast-agent administration or radiational dose, thus assisting diagnosis and management of patients with LV dysfunction and coronary artery disease.
更多查看译文
关键词
scar detection,deep-learning deep-learning,tomography,contrast-enhanced
AI 理解论文
溯源树
样例
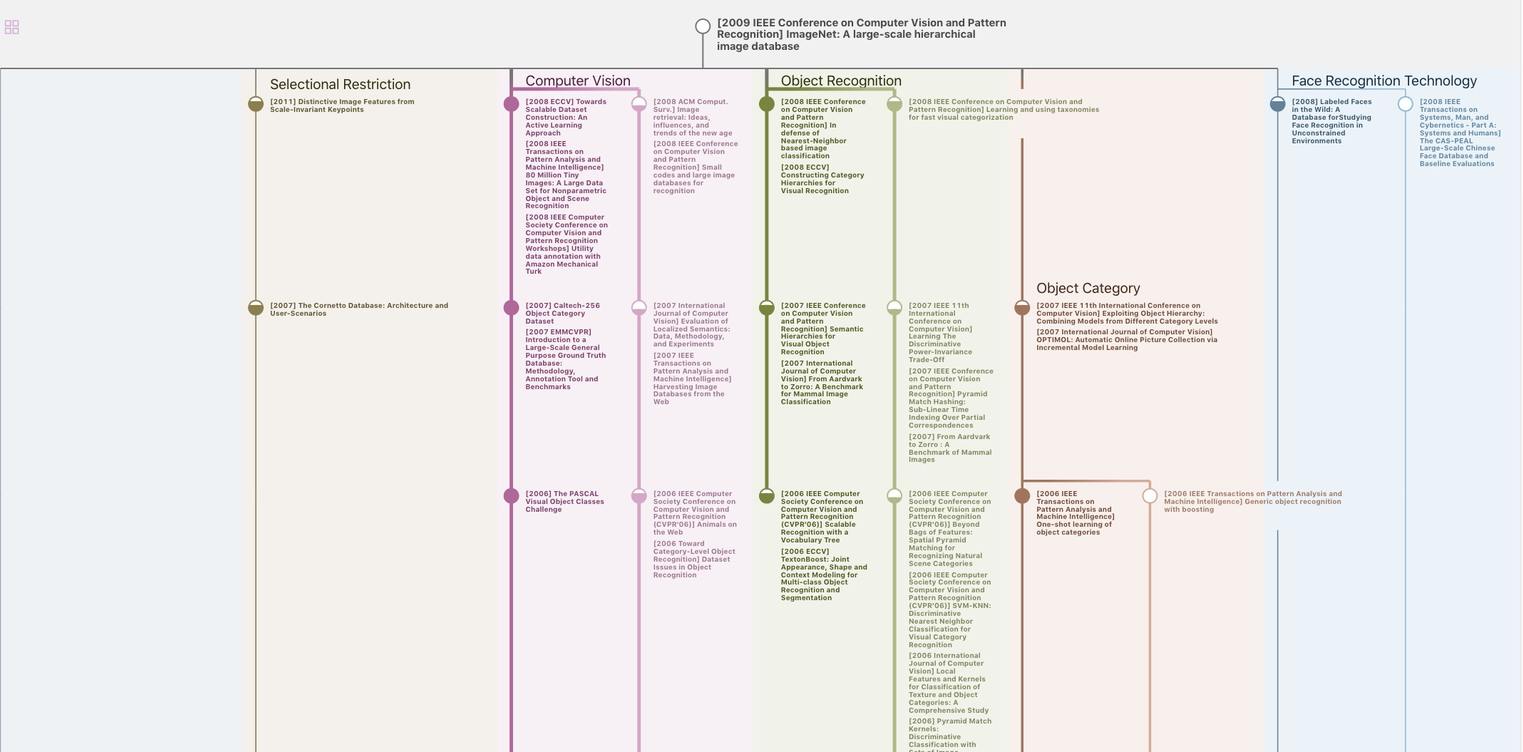
生成溯源树,研究论文发展脉络
Chat Paper
正在生成论文摘要