Forecasting the Pharmacokinetics With Limited Early Frames in Dynamic Brain PET Imaging Using Neural Ordinary Differential Equation
IEEE Transactions on Radiation and Plasma Medical Sciences(2023)
摘要
In dynamic brain positron emission tomography (PET) studies, acquiring a time series of images, typically lasting more than an hour, is necessary to derive pharmacokinetic parameters. Analytically, these parameters are estimated by establishing kinetic models such as compartment models that consist of sets of ordinary differential equations (ODEs), and by fitting the sparse time-activity curve (TAC) of the tracer. Yet, these models are simplified approximations of highly complex underlying processes, and sufficient samples of TAC are required throughout the entire acquisition, which is not only impractical but also hindered by patient involuntary motion and intrinsic noise. Therefore, recovering samples in missing timeframes are often required, which, in practice, is achieved by interpolation or extrapolation. Here, we introduce a novel deep-learning-based method that utilizes neural ODE (N-ODE) to predict TAC in the extended timeframes by mimicking the analytical method in a data-driven manner. By training N-ODE to solve and fit sets of ODE such that the solution replicates the observed TAC, the N-ODE converges to the functional shapes that best describe the underlying pharmacokinetic processes. We customized N-ODE to predict the full-dynamic images (12 frames, 60 min), hence pharmacokinetic parameters, given limited early-frame images (7–9 frames, 20–30 min). For proof of concept, the proposed N-ODE was applied to simulated and clinical
$^{\mathrm{ 18}}\text{F}$
-PI-2620 brain PET. We demonstrated that the proposed N-ODE delivered promising performance, indicated by bias, variance, and mean absolute error as well as pharmacokinetic parameters, such as rate constants, standardized uptake value ratio (SUVr), and binding potential (
$\mathrm {BP}_{\mathrm {ND}}$
).
更多查看译文
关键词
Artificial intelligence, dynamic positron emission tomography (PET), neuroimaging, positron emission tomography PET
AI 理解论文
溯源树
样例
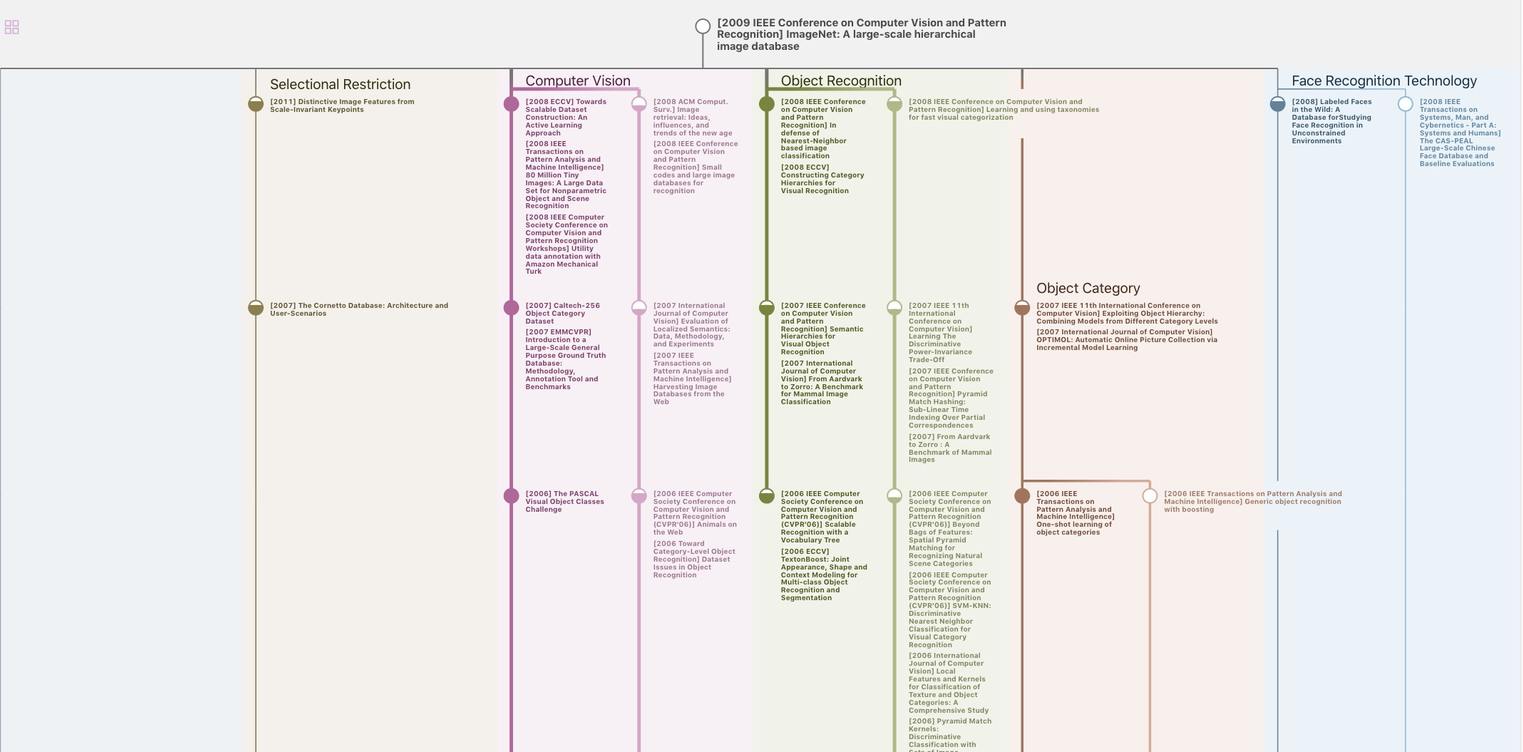
生成溯源树,研究论文发展脉络
Chat Paper
正在生成论文摘要