SOHO-FL: A Fast Reconvergent Intra-domain Routing Scheme Using Federated Learning
IEEE NETWORK(2024)
Beijing Univ Posts & Telecommun BUPT | Tsinghua Univ
Abstract
The development of machine learning provides a new paradigm for network optimizations, e.g., reinforcement learning (RL) has brought great improvements in many fields, such as adaptive video streaming, congestion control of TCP. The fundamental mechanism of such RL-based architectures is that the neural network decision model converges to a stable state by continuously interacting with network environment. However, for network routing problem, such RL-based strategies do not work well due to topology change. This is because topological changes would require the existing RL models to be retrained, while these models may stop making routing decisions or provide non-optimal decisions during the slow reconverging process of retraining, seriously affected transmission performance. To solve this problem, we proposed a fast convergent RL-model (SOHOFL), which can alleviate the performance degradation caused by the slow retraining process by federated learning. The experimental results based on real-world network topologies demonstrate that SOHO-FL outperforms the state-of-the-art algorithms in reconvergence time by 22.3% on average.
MoreTranslated text
Key words
Routing,Hidden Markov models,Data models,Computational modeling,Network topology,Optimization,Federated learning
求助PDF
上传PDF
View via Publisher
AI Read Science
AI Summary
AI Summary is the key point extracted automatically understanding the full text of the paper, including the background, methods, results, conclusions, icons and other key content, so that you can get the outline of the paper at a glance.
Example
Background
Key content
Introduction
Methods
Results
Related work
Fund
Key content
- Pretraining has recently greatly promoted the development of natural language processing (NLP)
- We show that M6 outperforms the baselines in multimodal downstream tasks, and the large M6 with 10 parameters can reach a better performance
- We propose a method called M6 that is able to process information of multiple modalities and perform both single-modal and cross-modal understanding and generation
- The model is scaled to large model with 10 billion parameters with sophisticated deployment, and the 10 -parameter M6-large is the largest pretrained model in Chinese
- Experimental results show that our proposed M6 outperforms the baseline in a number of downstream tasks concerning both single modality and multiple modalities We will continue the pretraining of extremely large models by increasing data to explore the limit of its performance
Upload PDF to Generate Summary
Must-Reading Tree
Example
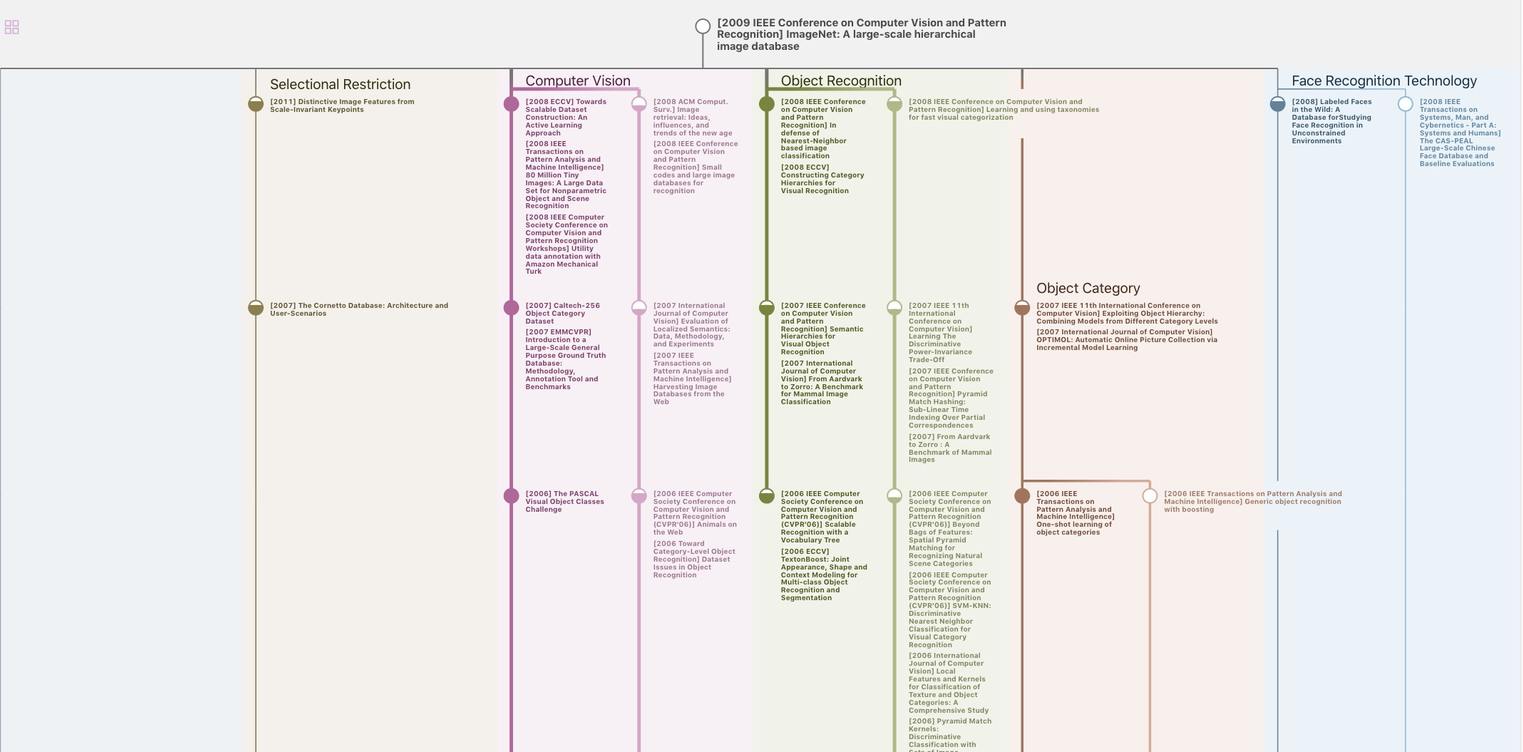
Generate MRT to find the research sequence of this paper
Related Papers
DIT and Beyond: Interdomain Routing with Intradomain Awareness for IIoT
IEEE INTERNET OF THINGS JOURNAL 2023
被引用3
FaCa: Fast Aware and Competition-Avoided Balancing for Data Center Network.
ALGORITHMS AND ARCHITECTURES FOR PARALLEL PROCESSING, ICA3PP 2023, PT VI 2024
被引用0
Data Disclaimer
The page data are from open Internet sources, cooperative publishers and automatic analysis results through AI technology. We do not make any commitments and guarantees for the validity, accuracy, correctness, reliability, completeness and timeliness of the page data. If you have any questions, please contact us by email: report@aminer.cn
Chat Paper