Deep Reinforcement Learning-Based Anti-Jamming Algorithm Using Dual Action Network
IEEE Transactions on Wireless Communications(2022)
摘要
Due to the open nature of wireless communication, malicious electromagnetic jamming has long been a severe threat to the establishment and stability of communication links. To address this anti-jamming problem, a Markov decision process (MDP) with a two-dimensional action space consisting of transmit frequency and power is proposed in this paper, modeling the interaction between a normal communication link and the presence of malicious jammers in a frequency hopping (FH) communication system. Furthermore, we also prove the existence of the deterministic optimal policy of the proposed model theoretically. To obtain a policy for the communication link to avoid being jammed, the Dual Action Network-Based Deep Reinforcement Learning Algorithm, and Action Feedback Mechanism are proposed. The energy consumption and frequency switching overhead are considered and evaluated in both the proposed model and the algorithm. Finally, the proposed model and algorithm are verified not only in a virtual simulation environment but also in the field testing environment. The result suggests that the proposed algorithm is of great practical value for solving anti-jamming problems.
更多查看译文
关键词
Anti-jamming,Markov decision process,deep reinforcement learning,dual action network,action feedback mechanism,power efficiency,frequency switching overhead,field testing
AI 理解论文
溯源树
样例
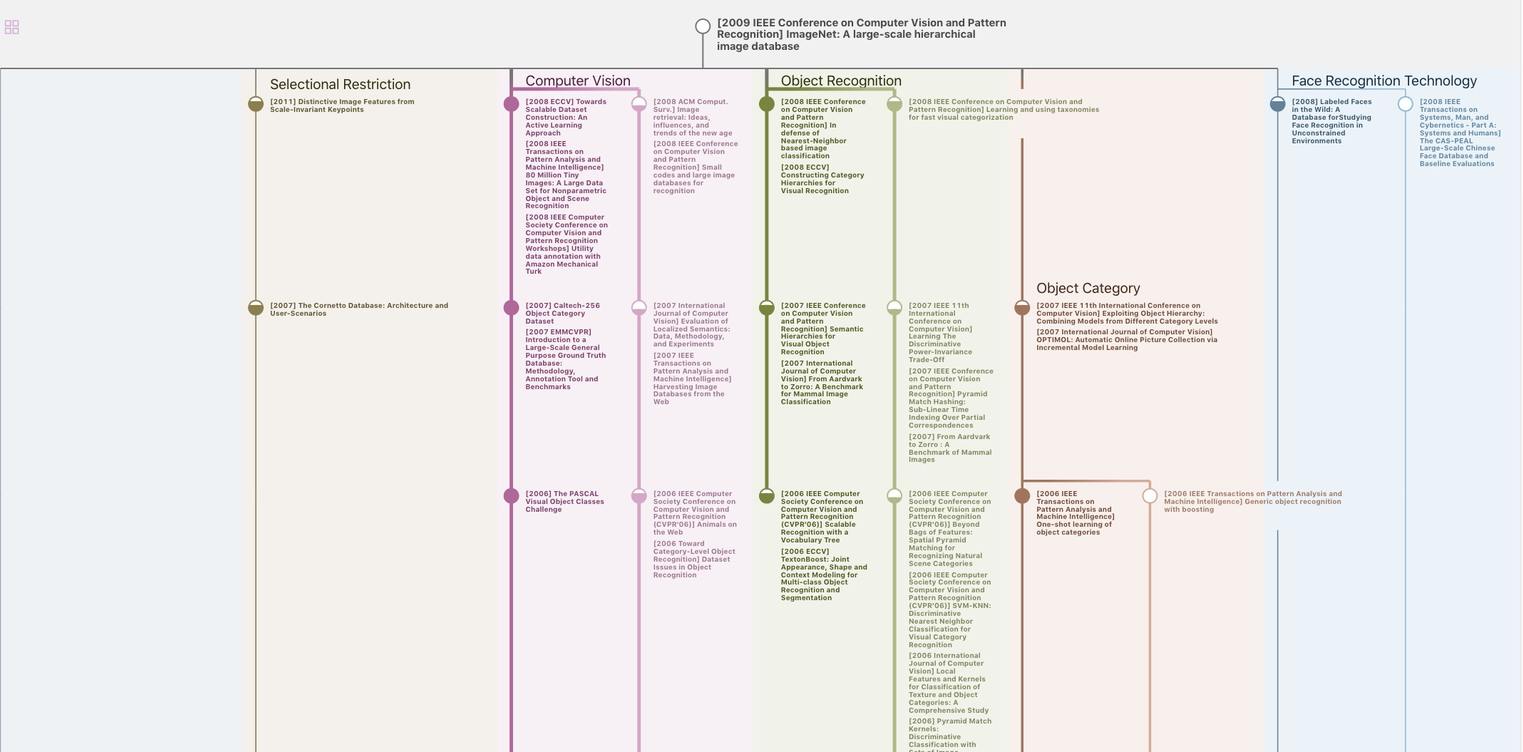
生成溯源树,研究论文发展脉络
Chat Paper
正在生成论文摘要