Amplifier Bias for Minimum Noise Figure in Thermally Constrained Systems
2021 51st European Microwave Conference (EuMC)(2022)
Abstract
The noise figure of low noise amplifiers (LNAs) critically depends, amongst others, on the biasing conditions and the transistor temperature. The latter may be constrained by the performance of the cooling system, in particular its thermal resistance, and the ambient temperature. This paper reports as a case study how the noise figure can be minimized in such constrained systems by properly adjusting the biasing conditions. For this, the two-dimensional noise figure dependence on the temperature and the drain current is measured for a commercial LNA. For a systematic mathematical treatment, the measured data are interpolated by means of two black-box models. In contrast to the first one, which requires the noise figure to be measured in a climate cabinet, the second one only necessitates room temperature data in addition to commonly available data sheet values. It is shown, that, depending on the thermal resistance of the cooling system and the ambient temperature, i.e., two constraining system parameters, an optimal drain current setting may reduce the dissipated power by up to about 50 % compared to the nominal data sheet value. As this lowers the heat load and, in turn, the device temperature, the noise figure can be improved by up to 0.4 dB.
MoreTranslated text
Key words
Adaptive biasing,low noise amplifier (LNA),noise figure,self heating,thermal management
求助PDF
上传PDF
View via Publisher
AI Read Science
AI Summary
AI Summary is the key point extracted automatically understanding the full text of the paper, including the background, methods, results, conclusions, icons and other key content, so that you can get the outline of the paper at a glance.
Example
Background
Key content
Introduction
Methods
Results
Related work
Fund
Key content
- Pretraining has recently greatly promoted the development of natural language processing (NLP)
- We show that M6 outperforms the baselines in multimodal downstream tasks, and the large M6 with 10 parameters can reach a better performance
- We propose a method called M6 that is able to process information of multiple modalities and perform both single-modal and cross-modal understanding and generation
- The model is scaled to large model with 10 billion parameters with sophisticated deployment, and the 10 -parameter M6-large is the largest pretrained model in Chinese
- Experimental results show that our proposed M6 outperforms the baseline in a number of downstream tasks concerning both single modality and multiple modalities We will continue the pretraining of extremely large models by increasing data to explore the limit of its performance
Upload PDF to Generate Summary
Must-Reading Tree
Example
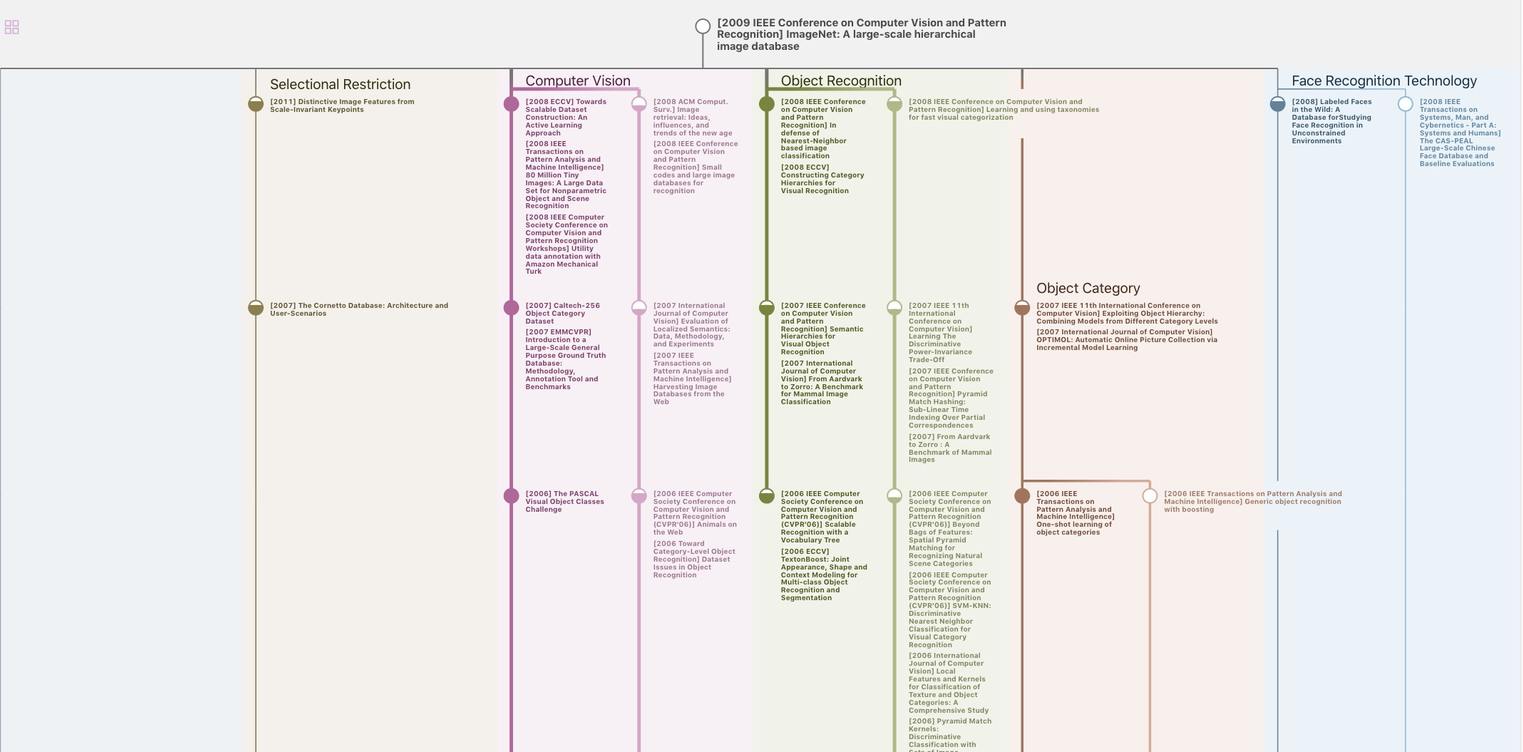
Generate MRT to find the research sequence of this paper
Related Papers
被引用707 | 浏览
1992
被引用84 | 浏览
2009
被引用20 | 浏览
2018
被引用11 | 浏览
2020
被引用38 | 浏览
Data Disclaimer
The page data are from open Internet sources, cooperative publishers and automatic analysis results through AI technology. We do not make any commitments and guarantees for the validity, accuracy, correctness, reliability, completeness and timeliness of the page data. If you have any questions, please contact us by email: report@aminer.cn
Chat Paper
GPU is busy, summary generation fails
Rerequest