Site and Season Specific Calibrations Improve Low-cost Sensor Performance: Long-term Field Evaluation of PurpleAir Sensors in Urban and Rural India
crossref(2023)
摘要
Abstract. We report on the long-term performance of a popular low-cost PM2.5 sensor, the PurpleAir PA-II, at multiple sites in India, with the aim of identifying robust calibration protocols. We established 3 distinct sites in India (North India: Delhi, Hamirpur; South India: Bangalore), where we collocated PA-II with reference beta-attenuation monitors to characterize sensor performance and to model calibration relationships between PA-IIs and reference monitors for hourly data. Our sites remained in operation across all major seasons of India. Without calibration, the PA-IIs had high precision (Normalized Root Mean Square Error [NRMSE] among replicate sensors ≤ 15 %) and tracked the overall seasonal and diurnal signals from the reference instruments well (Pearson’s r ≥ 0.9) but were inaccurate (NRMSE ≥ 40 %). We used a comprehensive feature selection process to create optimized site-specific calibrations. Relative to the uncalibrated data, parsimonious least-squares long-term calibration models improved PA-II performance at all sites (cross-validated NRMSE: 20–30 %, R2: 0.82–0.95), particularly by reducing seasonal and diurnal biases. Because aerosol properties and meteorology vary regionally, the form of these long-term models differed by site. Likewise, using a moving-window calibration, we find a calibration scheme using seasonally specific information somewhat improves performance relative to a static long-term calibration model. In contrast, we demonstrate that a successful short-term calibration exercise for one season may not transfer reliably to other seasons. Overall, we demonstrate how the PA-II, when paired with a careful calibration scheme, can provide actionable information on PM2.5 in India with only modest irreducible uncertainty.
更多查看译文
AI 理解论文
溯源树
样例
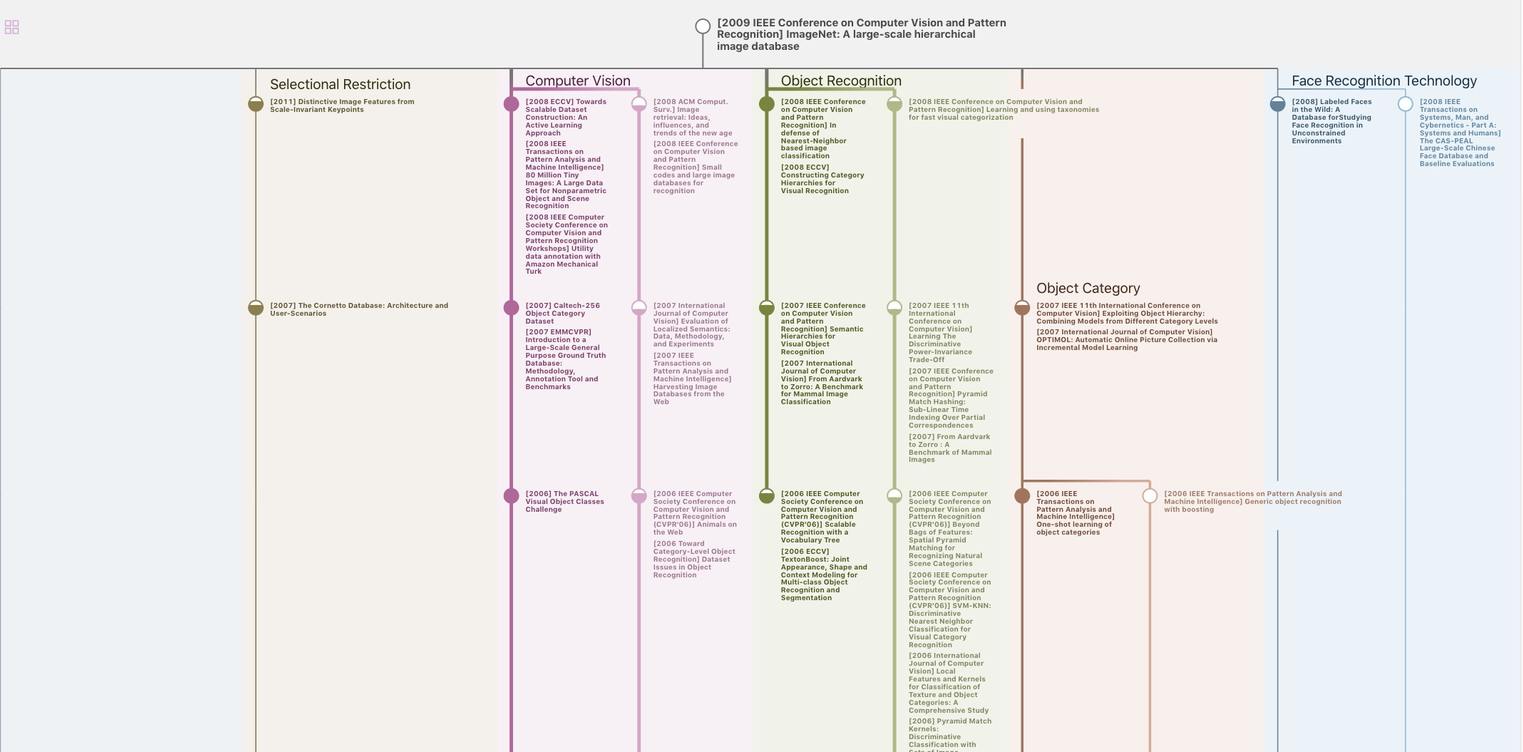
生成溯源树,研究论文发展脉络
Chat Paper
正在生成论文摘要