PoE: Poisoning Enhancement Through Label Smoothing in Federated Learning
IEEE Transactions on Circuits and Systems II: Express Briefs(2023)
摘要
In federated learning (FL), poisoning attack invades the whole system by manipulating the client data, tampering with the training target, and performing any desired behaviors. Until now, numerous poisoning attacks have been carefully studied, however they are still practically challenged in real-world scenarios from two aspects: (i)
multiple malicious client selections
- poisoning attacks are only successfully launched when the malicious client has been chosen in enough epochs (i.e., more than half of the epochs); (ii)
long-term poisoning training
- the poisoning training usually needs much more epochs than the normal training (i.e., 3 times longer than normal training), both are unavailable in real cases. To address these overlooked problems, we propose a
Po
isoning
${E}$
nhanced attack (PoE) against FLs, which is a general poisoning reinforcement framework. It is designed to transfer partially predicted probabilities of the source class to the target one. Thus, the inter-class distance between the source class and the target class is narrowed down in feature space for easier attacks. Towards this goal, the attack client uses label smoothing to change the model prediction distribution, dragging the global model in the direction that is favorable for poisoning. Extensive experiments show that PoE can significantly enhance the attack success rate (
$\sim \times8.4$
on average) in practical FLs with normal training epochs. It also achieves state-of-the-art adaptive attack performance against defensive FLs (i.e., robust aggregations). The code of PoE could be downloaded at
https://github.com/Leon022/poisoning_enhancement
.
更多查看译文
关键词
Training, Task analysis, Predictive models, Convergence, Data models, Toxicology, Smoothing methods, Federated learning, poisoning attack, secure aggregation, label smoothing
AI 理解论文
溯源树
样例
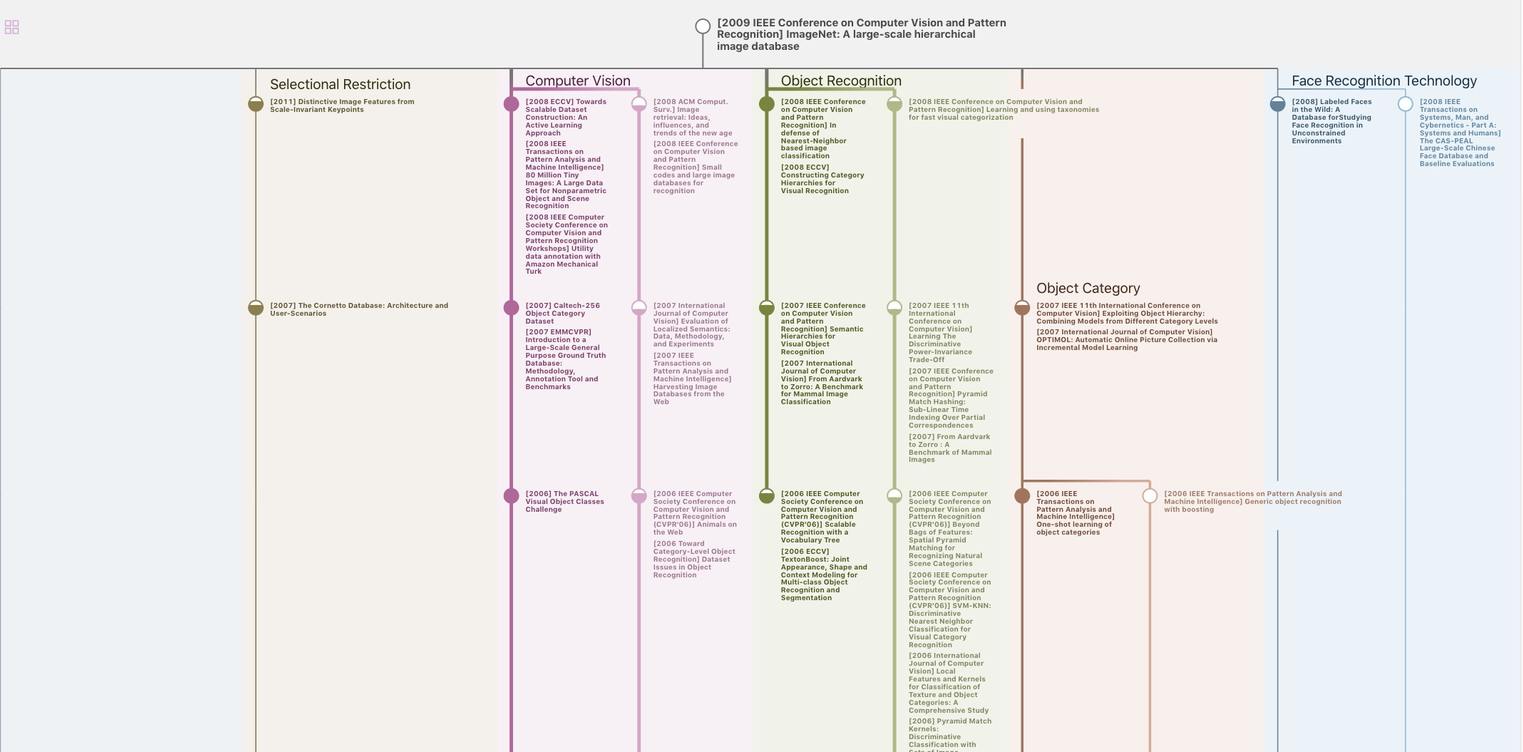
生成溯源树,研究论文发展脉络
Chat Paper
正在生成论文摘要