Faster R-CNN based parathyroid detection by using an automated annotation algorithm with a paired color/near-infrared imager
Advanced Biomedical and Clinical Diagnostic and Surgical Guidance Systems XXI(2022)
摘要
During thyroid surgery, parathyroid glands may be accidentally extracted due to their similar shapes and colors to the surrounding tissues (lymph nodes, fat, and thyroid tissue). In order to avoid damaging or resecting vulnerable glands, we aim to assist surgeons to better identify the parathyroids with real-time bounding boxes on a screen available in operating rooms. Parathyroids are auto fluorescent when excited with near infrared (NIR) light; therefore, videos recorded simultaneously in NIR, and RGB color formats can be used to train a deep learning model for robust object detection and localization without the need for expert annotation. The use of NIR images facilitates the generation of the ground truth dataset. We collected 16 patients' videos during total thyroidectomy. The videos were initially decomposed into a series of images taken at every 10 frames. From this, an intensity threshold was applied on the NIR images creating newer images where the parathyroid can be easily selected. Using these images, ground truth bounding boxes were generated. Our ground truth database size was over 600 images, of which 540 images contained parathyroid glands and 66 did not. We ran Faster R-CNN twice, initially to perform localization using the images with parathyroids only. The second method was to perform classification using the entire dataset. For the first method, we achieved an average intersection over union of 85% and for second, we obtained a precision of 98% and a recall of 100%. Given the limited dataset we are very excited with these results.
更多查看译文
关键词
parathyroids, NIR, Faster R-CNN, image processing, localization, classification
AI 理解论文
溯源树
样例
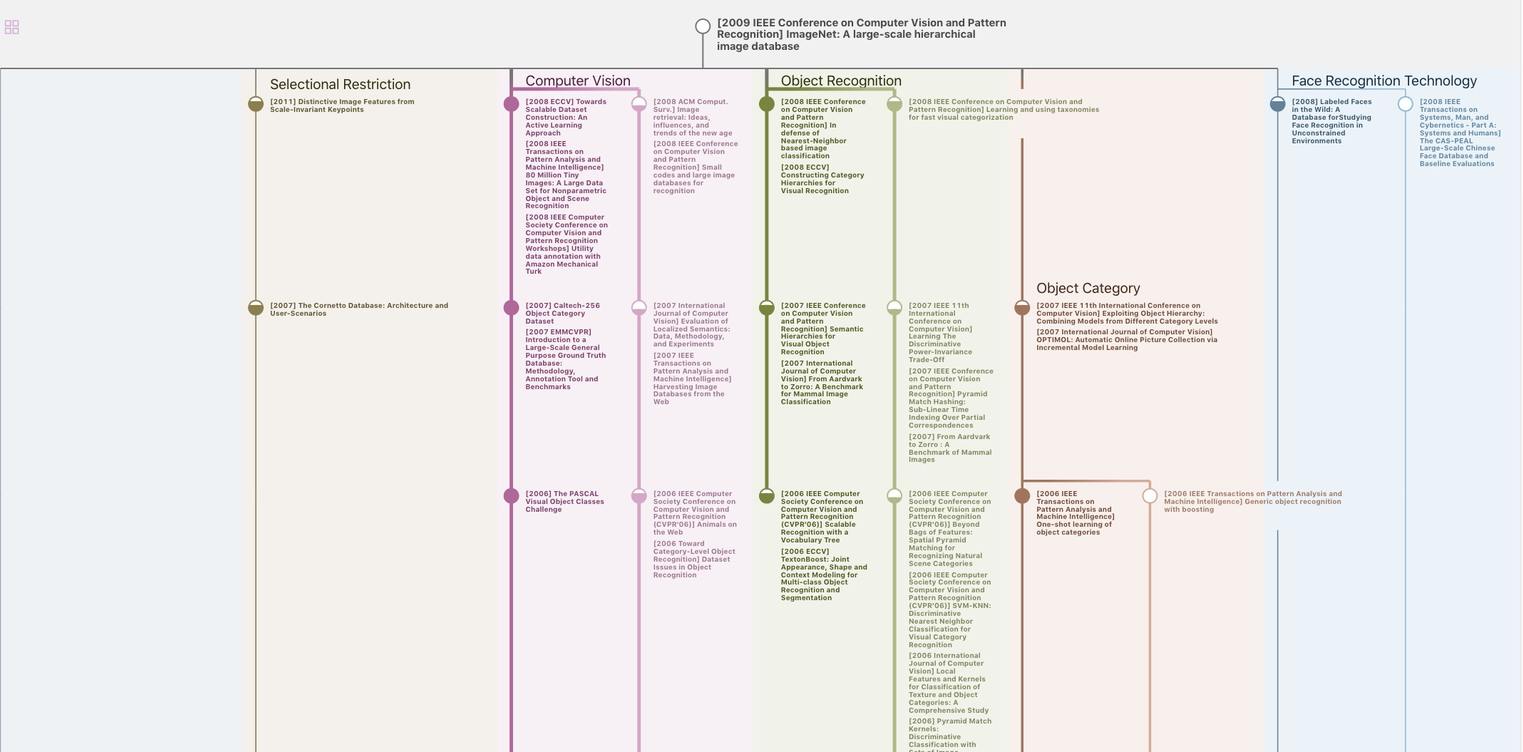
生成溯源树,研究论文发展脉络
Chat Paper
正在生成论文摘要