Deep learning model for the quantification of lung abnormalities in ILD patients
EUROPEAN RESPIRATORY JOURNAL(2022)
摘要
Lung fibrosis quantification from CT scans is prone to large inter and intra observer variability and its correlation with PFT is essential in the definition of disease progression. There is the need for a reliable and reproducible tool for abnormalities quantification. For this reason, a deep learning abnormalities quantification model was used to explore the correlation with PFT in ILD patients. The abnormalities segmentation model is based on 2D U-Net combined with Res Next as encoder and deep supervision and was trained on axial unenhanced chest CT scans of 199 COVID-19 patients and externally validated on 50 COVID-19 patients. Whole lungs were segmented using RadiomiX toolbox. Validation of the quantification performance was explored in a cohort of 20 ILD patients. The model performed the automatic segmentation of all abnormalities and calculate the ratio on the total lung volume ((abnormalities volume/whole lungs volume) * 100). This value is then correlated with the Forced Vital Capacity (FVC) and Diffusion Lung Capacity for carbon monoxide (DLCO) for each patient with Pearson correlation coefficient (ρ). The deep learning segmentation algorithm achieved good performances (mean DSC 0.6 ± 0.1) on the external test set. The percentage volume of disease region correlated with FVC and DLCO were the ρ = −0.70402, −0.58133, respectively (P <. 001 for all). The developed algorithm performed similarly to radiologists for disease-extent contouring, which correlated with pulmonary function to assess CT images from patients with ILD. This automatic quantification tool could help in the prognosis and diagnosis of ILDs, based on the lung abnormalities extent.
更多查看译文
AI 理解论文
溯源树
样例
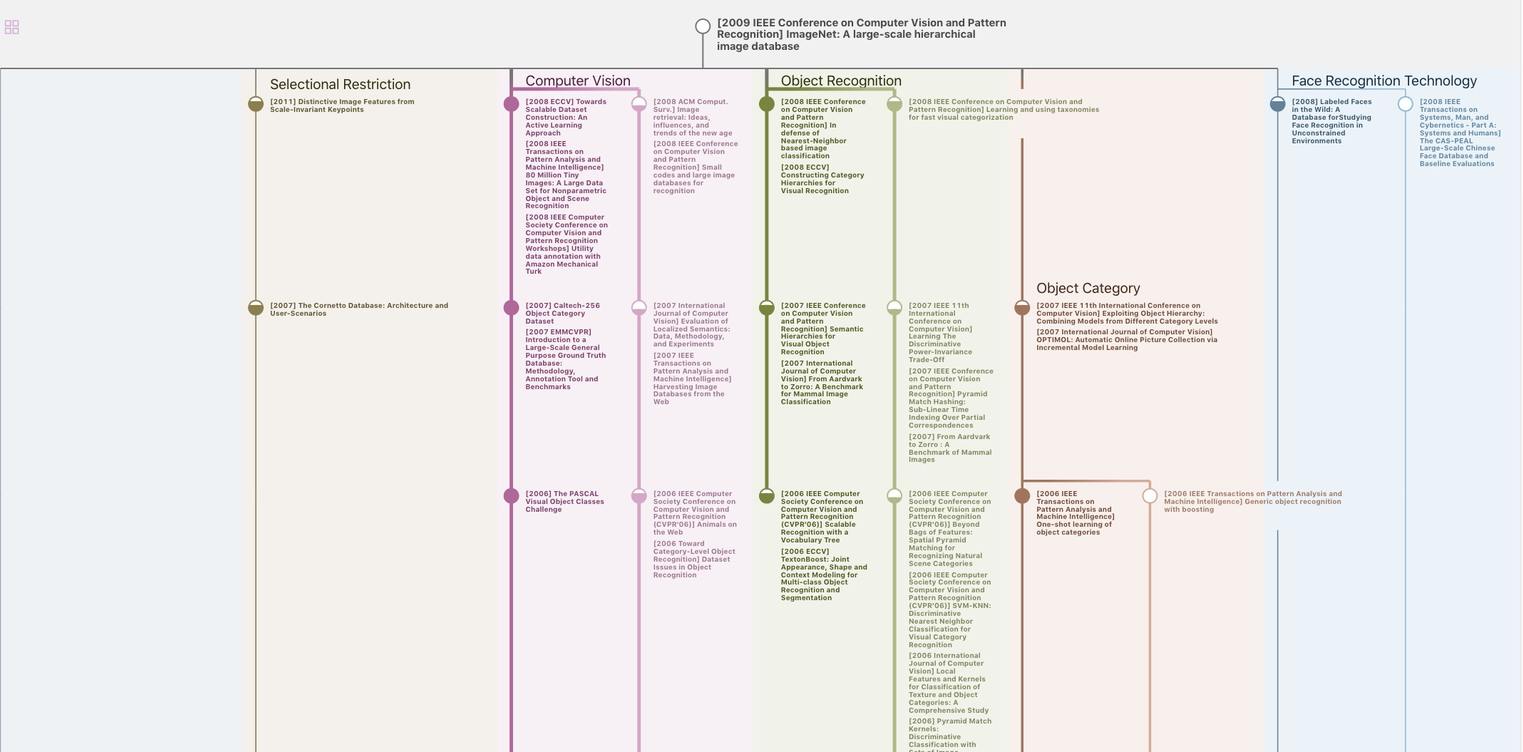
生成溯源树,研究论文发展脉络
Chat Paper
正在生成论文摘要