Automated Visual Evaluation (AVE) of cervical precancerous lesions with integrated patient meta-information: an initial study
Optics and Biophotonics in Low-Resource Settings IX(2023)
摘要
Cervical precancer - a major threat in low- and middle-income countries (LMICs) - is often screened through Visual Inspection with acetic Acid (VIA) but suffers from limited reproducibility and inaccuracy. Automatic Visual Evaluation (AVE) utilizes deep learning algorithms based on cervigram or digital images to detect cervical precancer accurately and efficiently. However, important patient data and test results that canprovide additional context to the images have not been integrated into the AVE workflow. The NCI ALTS dataset provides over 30,000 cervigram images with hundreds of histopathology- verified positive cases, paired with HPV, cytology, colposcopy, and histology results. To take advantage of both the images and other data, we designed a deep learning image classification algorithm, then modified its architecture to integrate age, HPV status, and cytology result with two methods - concatenation, and multiplication via an added "MetaNet". We then proposed a two-stage training process that maximized the predictive power of both the images and meta- information while allowing flexibility for missing data. This enabled us to run concurrent inference on a patient's images, test results, and age, and return a binary cervical precancer prediction. The experiments demonstrated that in certain scenarios, the MetaNet model produced a synergistic effect between the images and meta-information by outperforming models that only use each component individually, increasing specificity and maintaining high sensitivity. This work provides a direction for the integration of patient meta-information into an end-to-end AVE prediction model, and generally for other medical imaging data, to potentially increase prediction power and more precisely calibrate patient risk.
更多查看译文
关键词
AVE, HPV testing, cytology, deep learning, neural networks, multimodal
AI 理解论文
溯源树
样例
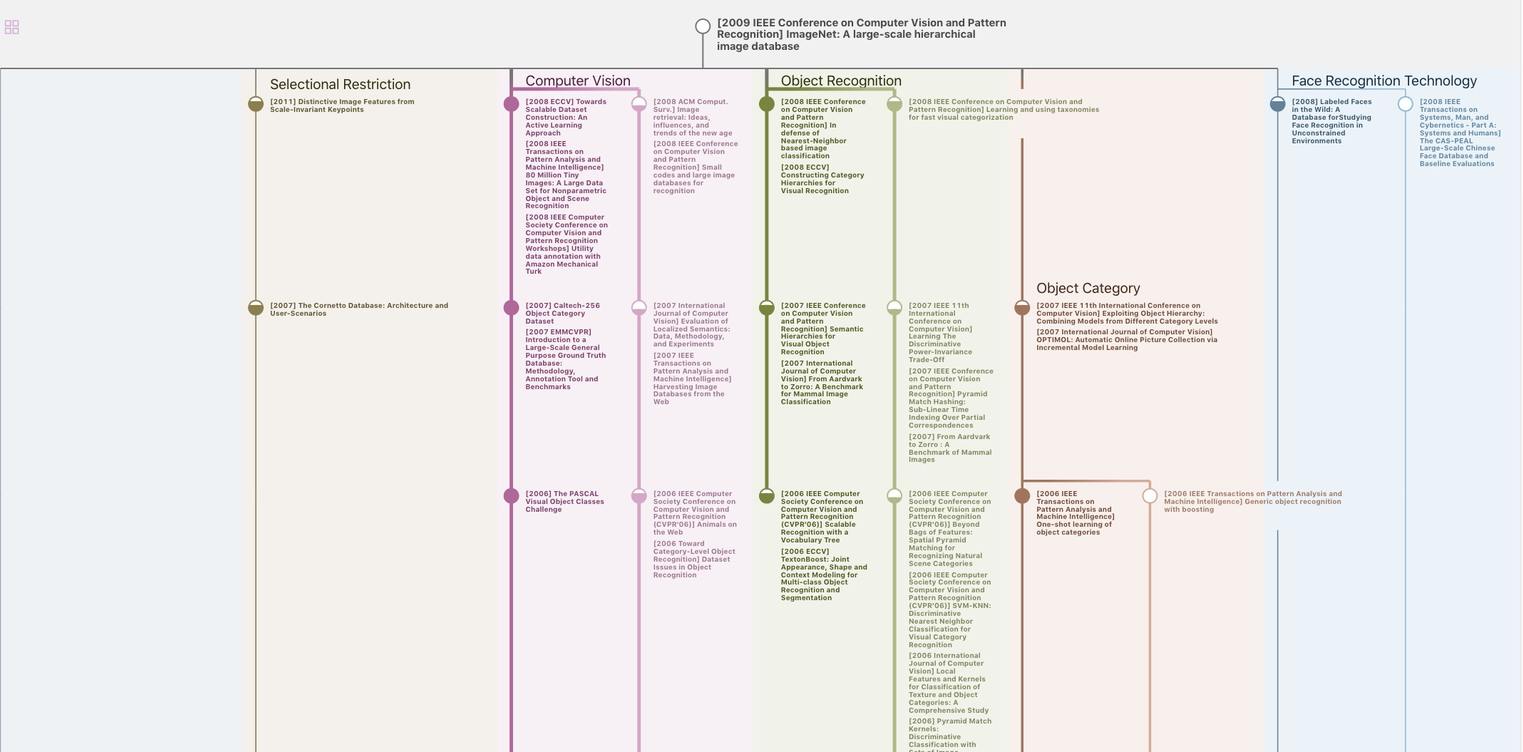
生成溯源树,研究论文发展脉络
Chat Paper
正在生成论文摘要