Fuzzy Representation Learning on Graph
IEEE Transactions on Fuzzy Systems(2023)
摘要
Recent years have witnessed a drastic surge in graph representation learning, which usually produces low-dimensional and crisp representations from graph topology and high-dimensional node attributes. Nevertheless, a crisp representation of a node or graph actually conceals the uncertainty and interpretability of features. In citation networks, for example, the reference between the two papers is always involved with fuzziness denoting the correlation degrees, that is, one connection may simultaneously belong to strong and weak references in different beliefs. The uncertainty in node connections and attributes inspires us to delve into fuzzy representations. This article, for the first time, proposes an unsupervised fuzzy representation learning model for graphs and networks to improve their expressiveness by making crisp representations fuzzy. Specifically, we develop a fuzzy graph convolution neural network (FGCNN), which could aggregate high-level fuzzy features, leveraging fuzzy logic to fully excavate feature-level uncertainties, and finally generate fuzzy representations. The corresponding hierarchical model composed of multiple FGCNNs, called deep fuzzy graph convolution neural network (DFGCNN), is able to generate fuzzy node representations which are more expressive than crisp ones. Experimental results of multiple network analysis tasks validate that the proposed fuzzy representation models have strong competitiveness against the state-of-the-art baselines over several real-world datasets.
更多查看译文
关键词
fuzzy representation learning,graph
AI 理解论文
溯源树
样例
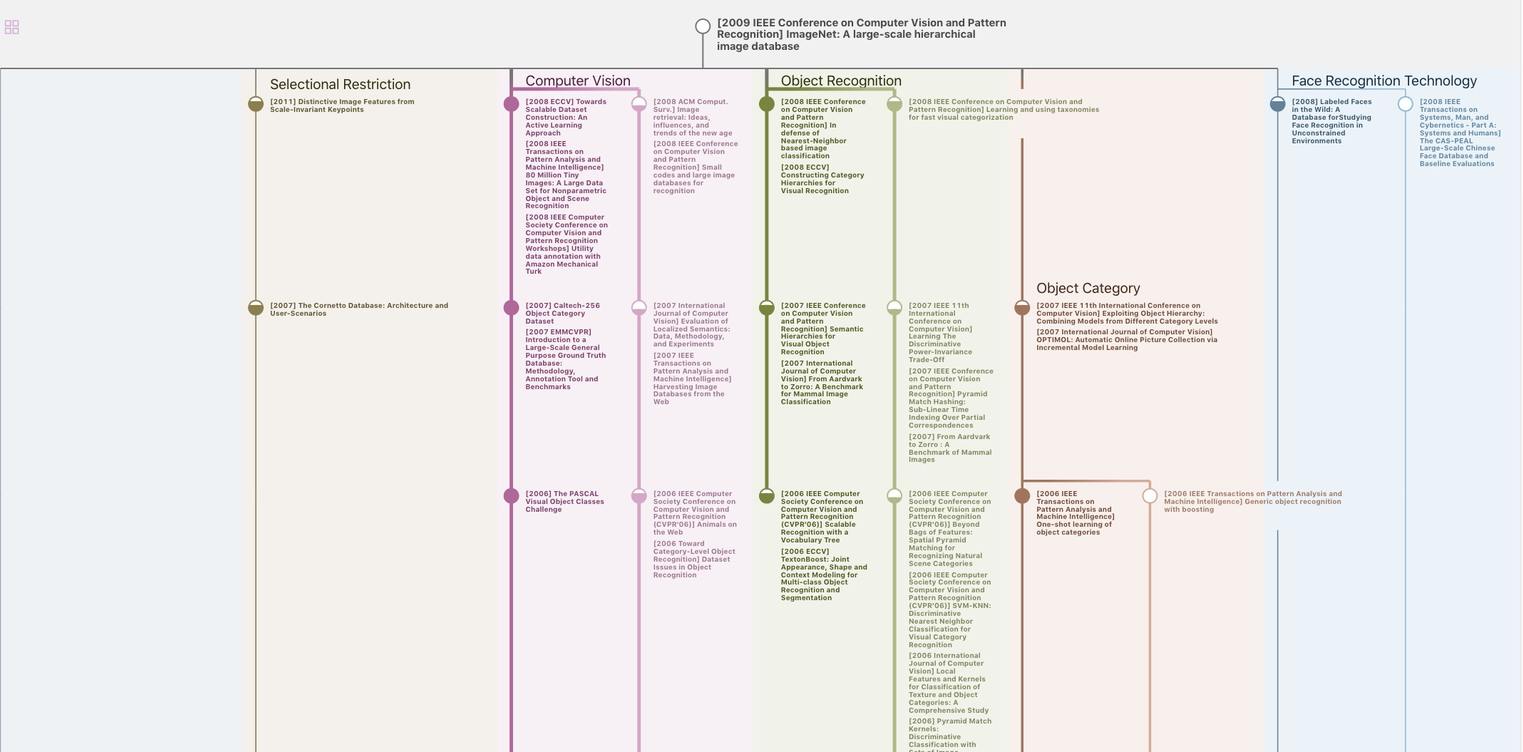
生成溯源树,研究论文发展脉络
Chat Paper
正在生成论文摘要