Imputing missing electroencephalography data using graph signal processing
18th International Symposium on Medical Information Processing and Analysis(2023)
摘要
Graph Signal Processing (GSP) is a framework for analyzing signals defined over a graph. Considering the electrodes used to record the electroencephalogram (EEG) as a sensor network makes it possible to use GSP to analyze EEG signals. Using the graph over which the signal is defined allows one to take advantage of a signal structure that is ignored by classic signal processing approaches. However, there are many details about how to use GSP to analyze the EEG that are not studied in the literature. Here we show an example of how to impute missing EEG data using GSP. We show that GSP allows reconstructing EEG missing data with a lower error than a classic approach based on radial basis functions, confirming that the underlying graph over a graph over which the signal is defined contains relevant information that can be exploited to improve a given signal processing task. By studying two approaches for building the graph (k-nearest neighbors and a thresholded Gaussian kernel) and the effect of its parameter, we highlight the importance of building the graph appropriately. These results show the potential of incorporating GPS techniques into the EEG processing pipeline.
更多查看译文
关键词
Electroencephalography, graph signal processing, missing data
AI 理解论文
溯源树
样例
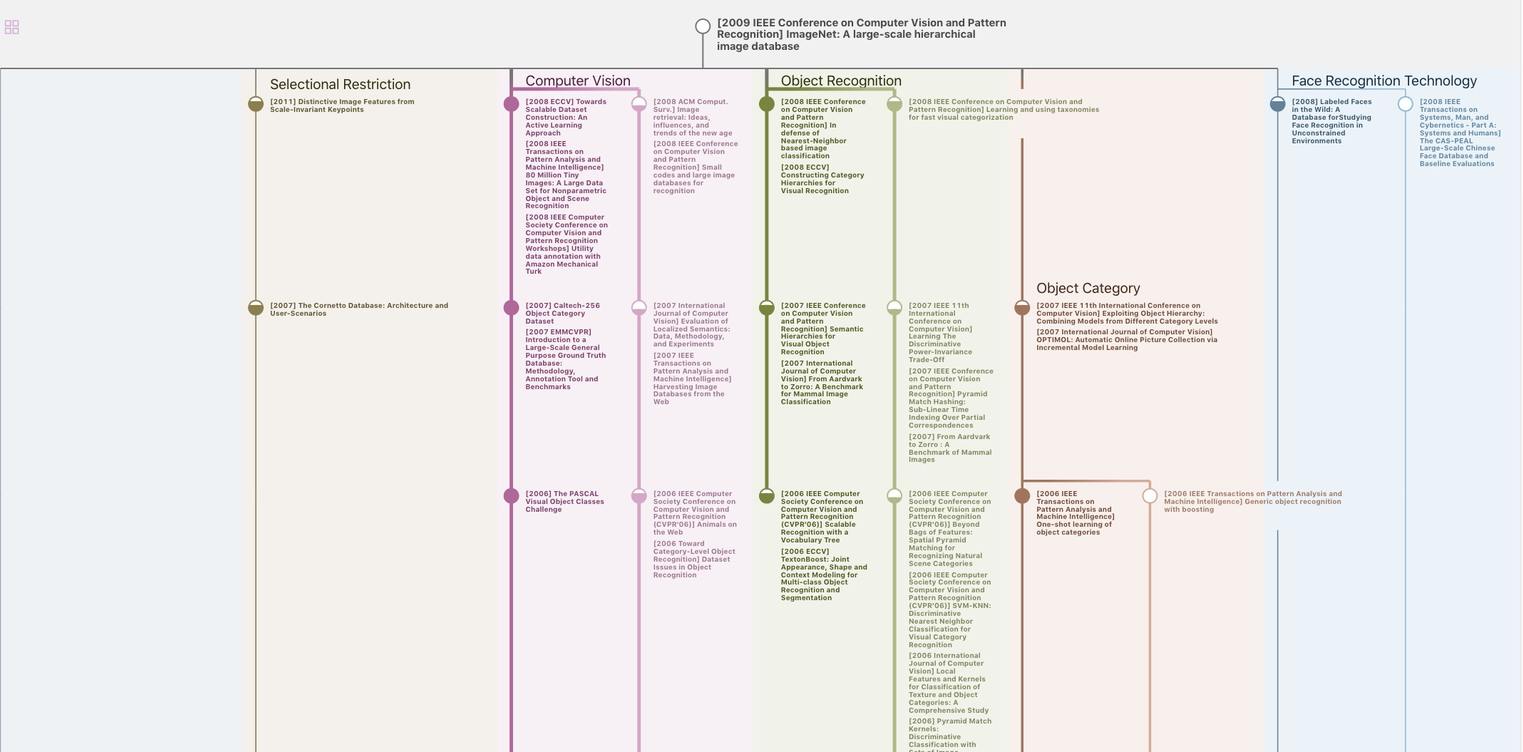
生成溯源树,研究论文发展脉络
Chat Paper
正在生成论文摘要