Graph Convolution Neural Network Based End-to-End Channel Selection and Classification for Motor Imagery Brain–Computer Interfaces
IEEE Transactions on Industrial Informatics(2023)
摘要
Classification of electroencephalogram-based motor imagery (MI-EEG) tasks is crucial in brain–computer interface (BCI). EEG signals require a large number of channels in the acquisition process, which hinders its application in practice. How to select the optimal channel subset without a serious impact on the classification performance is an urgent problem to be solved in the field of BCIs. This article proposes an end-to-end deep learning framework, called EEG channel active inference neural network (EEG-ARNN), which is based on graph convolutional neural networks (GCN) to fully exploit the correlation of signals in the temporal and spatial domains. Two channel selection methods, i.e., edge-selection (ES) and aggregation-selection (AS), are proposed to select a specified number of optimal channels automatically. Two publicly available BCI Competition IV 2a (BCICIV 2a) dataset and PhysioNet dataset and a self-collected dataset (TJU dataset) are used to evaluate the performance of the proposed method. Experimental results reveal that the proposed method outperforms state-of-the-art methods in terms of both classification accuracy and robustness. Using only a small number of channels, we obtain a classification performance similar to that of using all channels. Finally, the association between selected channels and activated brain areas is analyzed, which is important to reveal the working state of brain during MI.
更多查看译文
关键词
Brain computer interface (BCI), channel selection, graph convolutional network (GCN), motor imagery (MI)
AI 理解论文
溯源树
样例
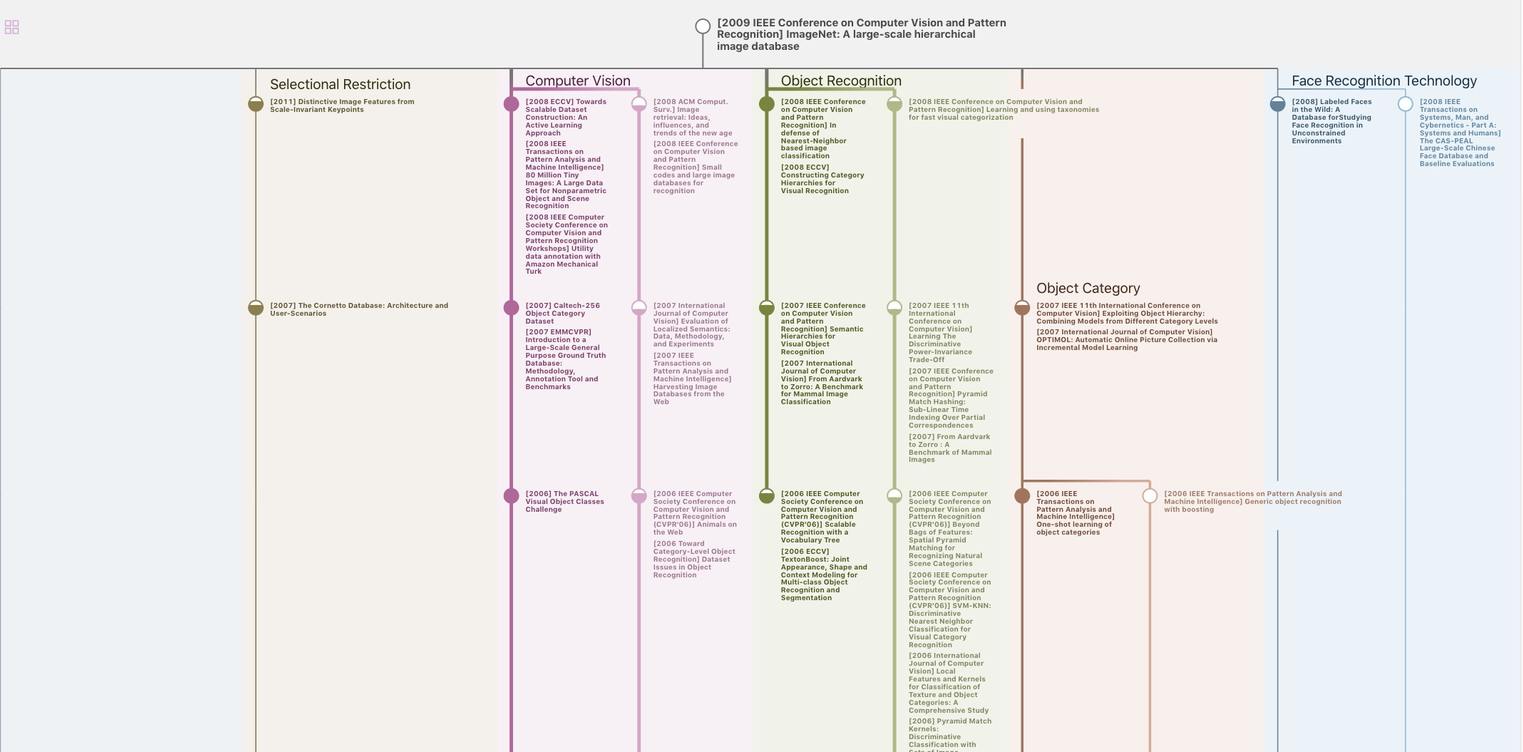
生成溯源树,研究论文发展脉络
Chat Paper
正在生成论文摘要