GRRIEn analysis: a data science cheat sheet for earth scientists learning from global earth observations
Artificial Intelligence for the Earth Systems(2023)
摘要
Abstract Globally available environmental observations (EOs), specifically from satellites and coupled earth systems models, represent some of the largest datasets of the digital age. As the volume of global EOs continues to grow, so does the potential of this data to help earth scientists discover trends and patterns in earth systems at large spatial scales. To leverage global EOs for scientific insight, earth scientists need targeted and accessible exposure to skills in reproducible scientific computing and spatiotemporal data science, and to be empowered to apply their domain understanding to interpret data-driven models for knowledge discovery. The GRRIEn (Generalizable, Reproducible, Robust, and Interpreted Environmental) analysis framework was developed to prepare earth scientists with an introductory statistics background and limited/no understanding of programming and computational methods to use global EOs to successfully generalize insights from local/regional field measurements across unsampled times and locations. GRRIEn analysis is generalizable, meaning results from a sample are translated to landscape scales by combining direct environmental measurements with global EOs using supervised machine learning; robust, meaning that model shows good performance on data with scale-dependent feature and observation dependence; reproducible, based on a standard repository structure so that other scientists can quickly and easily replicate the analysis with a few computational tools; and interpreted, meaning that earth scientists apply domain expertise to ensure that model parameters reflect a physically plausible diagnosis of the environmental system. This tutorial presents standard steps for achieving GRRIEn analysis by combining conventions of rigor in traditional experimental design with the open-science movement.
更多查看译文
关键词
earth scientists learning,data science cheat sheet,observations
AI 理解论文
溯源树
样例
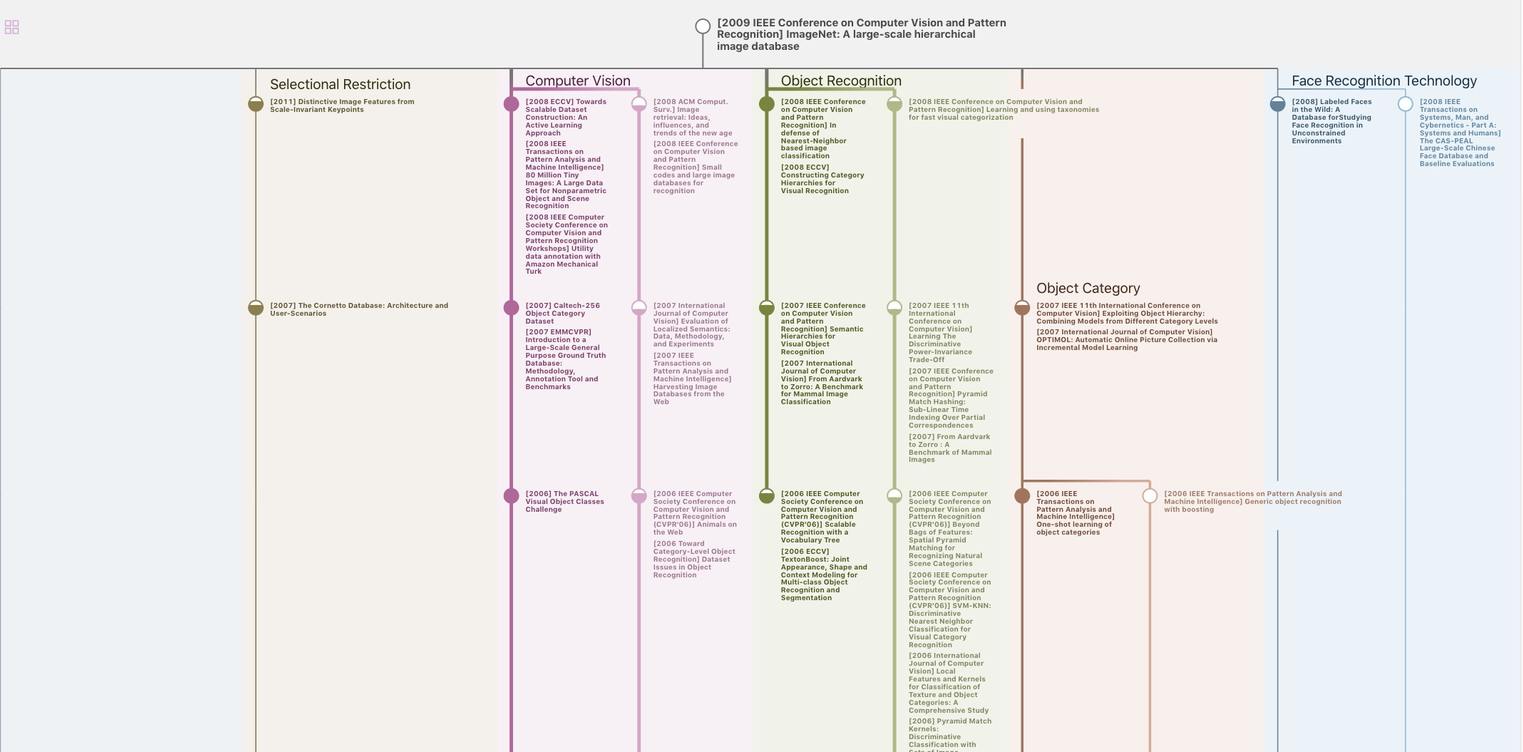
生成溯源树,研究论文发展脉络
Chat Paper
正在生成论文摘要