Developing a supplementary diagnostic tool for breast cancer risk estimation using ensemble transfer learning
crossref(2023)
摘要
This study utilised an ensemble of pre-trained networks and digital mammograms to develop a supplementary diagnostic tool for radiologists. Digital mammograms and their associated information were collected from the department of radiology and pathology, Hospital Universiti Sains Malaysia. Thirteen pre-trained networks were selected and explored in this study. ResNet101V2 and ResNet152 had the highest mean PR-AUC, MobileNetV3Small and ResNet152 had the highest mean precision, ResNet101 had the highest mean F1 score, and ResNet152 and ResNet152V2 had the highest mean Youden J index. Subsequently, three ensemble models were developed using the top three pre-trained networks based on PR-AUC, precision, and F1 score. The final ensemble model had a mean precision, F1 score, and Youden J index of 0.82, 0.68, and 0.12, respectively. Additionally, the final model demonstrated a balanced performance across mammographic density. In conclusion, this study exhibited the good performance of the ensemble transfer learning on digital mammograms for the purpose of breast cancer risk estimation. This model can be utilised as a supplementary diagnostic tool for radiologists, thus, reducing their workloads.
更多查看译文
AI 理解论文
溯源树
样例
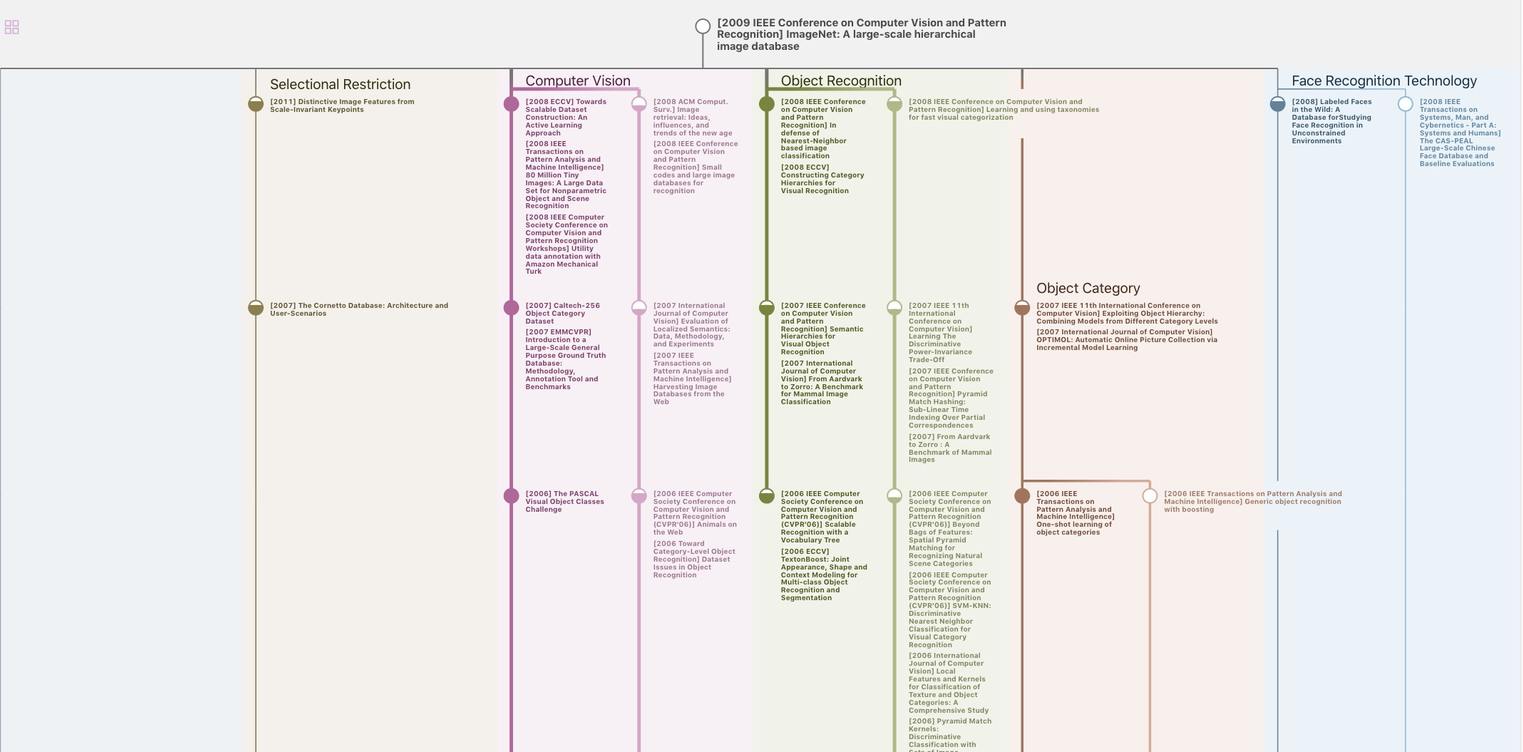
生成溯源树,研究论文发展脉络
Chat Paper
正在生成论文摘要