Transfer Subspace Learning via Label Release and Contribution Degree Distinction
Information Sciences(2023)
摘要
For sample face recognition issues, the transfer subspace learning approach works well. However, the majority of transfer subspace learning techniques now in use cannot decrease intra-class differences while increasing inter-class differences. In addition, when reconstructing samples, the contribution of each reconstructed sample is not considered. In this paper, we propose a Label Release and Contribution Degree Distinction (LRCDD)-based transfer subspace learning strategy to enhance recognition performance to solve these problems. Specifically, LRCDD reduces the intra-class differences and expands the inter-class differences by introducing the label release model into subspace learning. Assigning an unknown weight coefficient to the representation coefficient of each sample and performing weight learning makes the reconstructed sample in the subspace learning process more accurate, and thus a better transformation matrix or subspace is learned. We introduce a constraint of null diagonal to prevent the data sample from being represented by itself. This avoids multiple representations for each data point. Experimental result demonstrates that the average recognition rate of LRCDD on the EYB, AR, IJB-C, MegaFace, RFW, CPLFW, Flickr-Faces-HQ and Tufts-Face databases are 62.51%, 70.58%, 81.69%, 83.66%, 82.26%, 73.22%, 84.97% and 90.91%, respectively, which are higher than those of the state-of-the-art methods.
更多查看译文
关键词
label release,learning,transfer
AI 理解论文
溯源树
样例
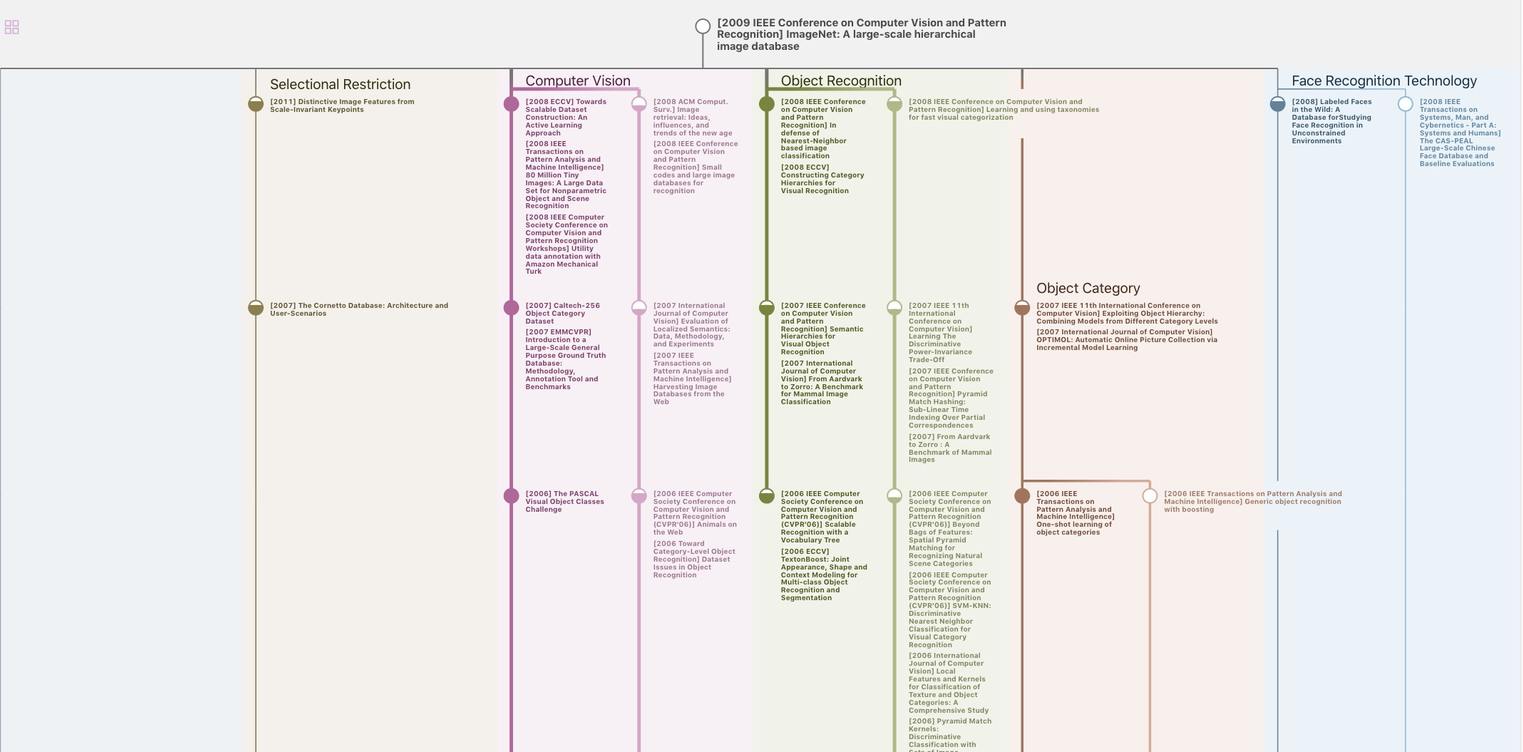
生成溯源树,研究论文发展脉络
Chat Paper
正在生成论文摘要