Abstract P6-01-19: Multiparametric MRI-based Longitudinal-radiomics Analysis for Early Prediction of Treatment Response of Breast Cancers to Neoadjuvant Chemotherapy: A Multicenter Study
Cancer Research(2023)
摘要
Abstract Purpose: We evaluated the performance of the longitudinal-radiomics of multi-parametric magnetic resonance imaging (MRI), developed and validated based on a multicenter dataset adopting a radiomic strategy, for prediction of treatment response to neoadjuvant chemotherapy (NAC) in breast cancer. Methods and materials: We analyzed clinical and pathological data of patients with breast cancer and compared machine-learning classification algorithms in predicting treatment response following NAC from four hospitals (primary cohort and external validation cohort 1-3) between July 2015 and December 2021. Patients were monitored with MRI before (pretreatment) and after (mid-treatment) three or four cycles of NAC. Longitudinal-radiomics analysis was performed at pre- and mid-treatment dynamic contrast-enhanced, T2-weighted imaging, and diffusion-weighted imaging mapping by using 3D Slicer software. Radiomics features were extracted from original and filtered images using PyRadiomics (V3.0.1) filtered images were generated using Laplacian of Gaussian and wavelet filters. The stacking strategy including LR, LDA and SVM (radial basis function) were used to combined the results of the base models, and gave a secondary prediction result. Results: In total, 1048 cases were enrolled in the study. In identifying the residual cancer burden (RCB) 0 v.s. RCB 1-3, longitudinal-radiomic models achieved the highest area under the receiver operating characteristic curve (AUC) of 0.909 and 0.893 in the training and test sets. The accuracies of the stacking-SVM classifier were 84.3% (86/102), 75.6% (205/271) and 77.9% (265/340) in the external validation set. In identifying the RCB 3 v.s. RCB 0-2, longitudinal-radiomic models achieved the highest AUC of 0.981 and 0.923 in the training and test sets. In the external validation set, the performance was with accuracy=91.2%-92.2%. The cascade model also stratified 78.9%, 69.1% and 76.1% of the RCB 1-2 patients into RCB 1-2 prediction group to receive suitable NAC and feasible breast-conserving surgery. Our findings showed high predictive ability and reproducibility across multicenter dataset. By two-step prediction results of cascade models, the patient could be assigned to one of the three groups corresponding to three RCB status: RCB 0, RCB 1-2, or RCB 3. In this way, using the cascade models, some of RCB 3 patients may allow treatment to be tailored for optimal outcomes. RCB 0 and RCB 1-2 groups could receive suitable NAC and feasible breast-conserving surgery. Conclusion: In conclusion, our study had good predictive ability for RCB assessment, which could help clinicians guide individualized treatment options for breast cancer patients in NAC. The application of stacking cascade model may have the potential to further reduce unnecessary NAC and economic costs associated with current breast cancer management. Figure 1. The flowchart of the study. This was a multicenter study with patients retrospectively enrolled from four medical centers in different cities of China. Pretreatment and midtreatment multi-parametric MRI (DCE, T2WI, and DWI) and clinical information were proposed to predict treatment response to NAC in patients with primary invasive breast cancer. The data from Foshan was used as the primary cohort (PC), and the other three data sets were used as independent validation cohorts (VC). This study included radiomic feature engineering, model building, model comparison and clinical application. Figure 1. The flowchart of the study. This was a multicenter study with patients retrospectively enrolled from four medical centers in different cities of China. Pretreatment and midtreatment multi-parametric MRI (DCE, T2WI, and DWI) and clinical information were proposed to predict treatment response to NAC in patients with primary invasive breast cancer. The data from Foshan was used as the primary cohort (PC), and the other three data sets were used as independent validation cohorts (VC). This study included radiomic feature engineering, model building, model comparison and clinical application. Figure 2. ROC curves among different radiomic signatures and among different models in identifying the RCB 0 vs RCB 1-3. RCB, residual cancer burden; ROC, receiver operating characteristic; SVM, support vector machine; RF, random forest; MLP, multilayer perceptron; LR, logistic regression. Figure 2. ROC curves among different radiomic signatures and among different models in identifying the RCB 0 vs RCB 1-3. RCB, residual cancer burden; ROC, receiver operating characteristic; SVM, support vector machine; RF, random forest; MLP, multilayer perceptron; LR, logistic regression. Figure 3. ROC curves among different radiomic signatures and among different models in identifying the RCB 3 vs RCB 0-2. RCB, residual cancer burden; ROC, receiver operating characteristic; SVM, support vector machine; RF, random forest; MLP, multilayer perceptron; LR, logistic regression. Figure 3. ROC curves among different radiomic signatures and among different models in identifying the RCB 3 vs RCB 0-2. RCB, residual cancer burden; ROC, receiver operating characteristic; SVM, support vector machine; RF, random forest; MLP, multilayer perceptron; LR, logistic regression. Citation Format: wei li, Yuhong huang, teng zhu, zhiyong wu, ying lin, guolin ye, Kun Wang. Multiparametric MRI-based Longitudinal-radiomics Analysis for Early Prediction of Treatment Response of Breast Cancers to Neoadjuvant Chemotherapy: A Multicenter Study [abstract]. In: Proceedings of the 2022 San Antonio Breast Cancer Symposium; 2022 Dec 6-10; San Antonio, TX. Philadelphia (PA): AACR; Cancer Res 2023;83(5 Suppl):Abstract nr P6-01-19.
更多查看译文
关键词
neoadjuvant chemotherapy,breast cancers,mri-based,longitudinal-radiomics
AI 理解论文
溯源树
样例
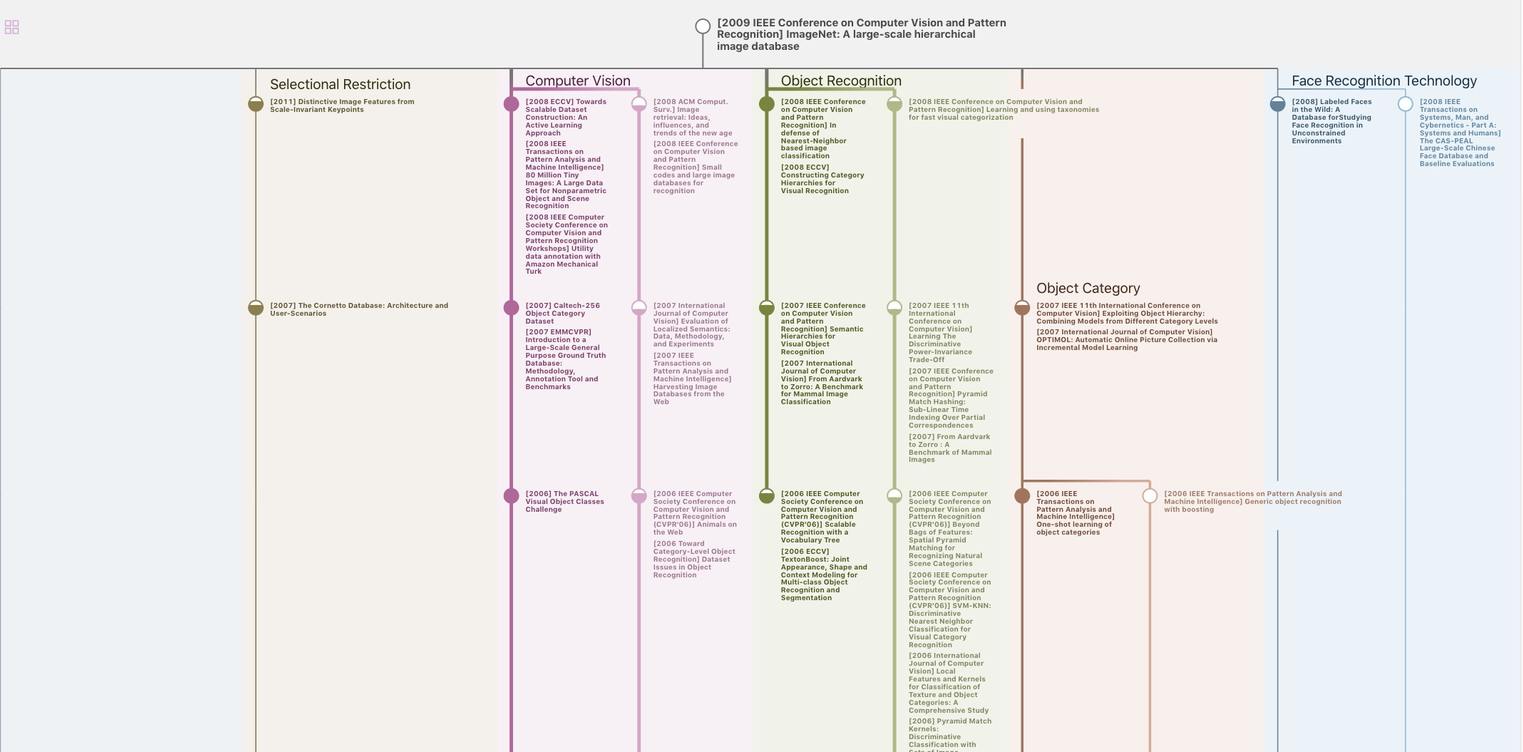
生成溯源树,研究论文发展脉络
Chat Paper
正在生成论文摘要