Smartphone App for patients with hemiplegia to support self-administered graded upper extremity exercise using deep learning model: Development and Usability Study (Preprint)
crossref(2022)
摘要
BACKGROUND Regular self-administered exercise after stroke can improve upper limb function. For proper self-administered exercise, an appropriate guide is required to match the exercise level to the patient’s upper limb disability. OBJECTIVE This study aimed to develop a mobile health application to support self-administered graded upper limb exercises for patients with hemiplegia using deep learning model. METHODS To develop a patient-centered, solution-based mobile application for upper extremity exercises for hemiplegia, we used a 5-step design thinking process. The exercise programs in the application were safe and easy to follow in the sitting position. A deep learning model was proposed to automatically evaluate the patients’ upper limb function for recommendation of exercise according to each patient’s disability. The model captured videos of patients reaching and grasping objects on a table as input and predicted the exercise level. Potential end-users, including patients, therapists, and physicians, evaluated the prototype and provided feedback for the usability and quality of the application using a mobile application rating scale. RESULTS A total of six features, my information, evaluation section, daily exercise, list of exercises, my current exercise status, and notification, were incorporated into the mobile application. The application consisted of 58 exercises categorized as postural and balance, range of motion, strengthening, and task-oriented training exercises with three levels of difficulty. A deep-learning-based model was proposed for automated upper limb function evaluation. The overall accuracy of classification of exercised level was 84.58% and inference time was 9~10 seconds. CONCLUSIONS We successfully developed a mobile application to support self-administered graded upper limb exercises for patients with hemiplegia based on individual ability, including automatic evaluation using a deep learning model.
更多查看译文
AI 理解论文
溯源树
样例
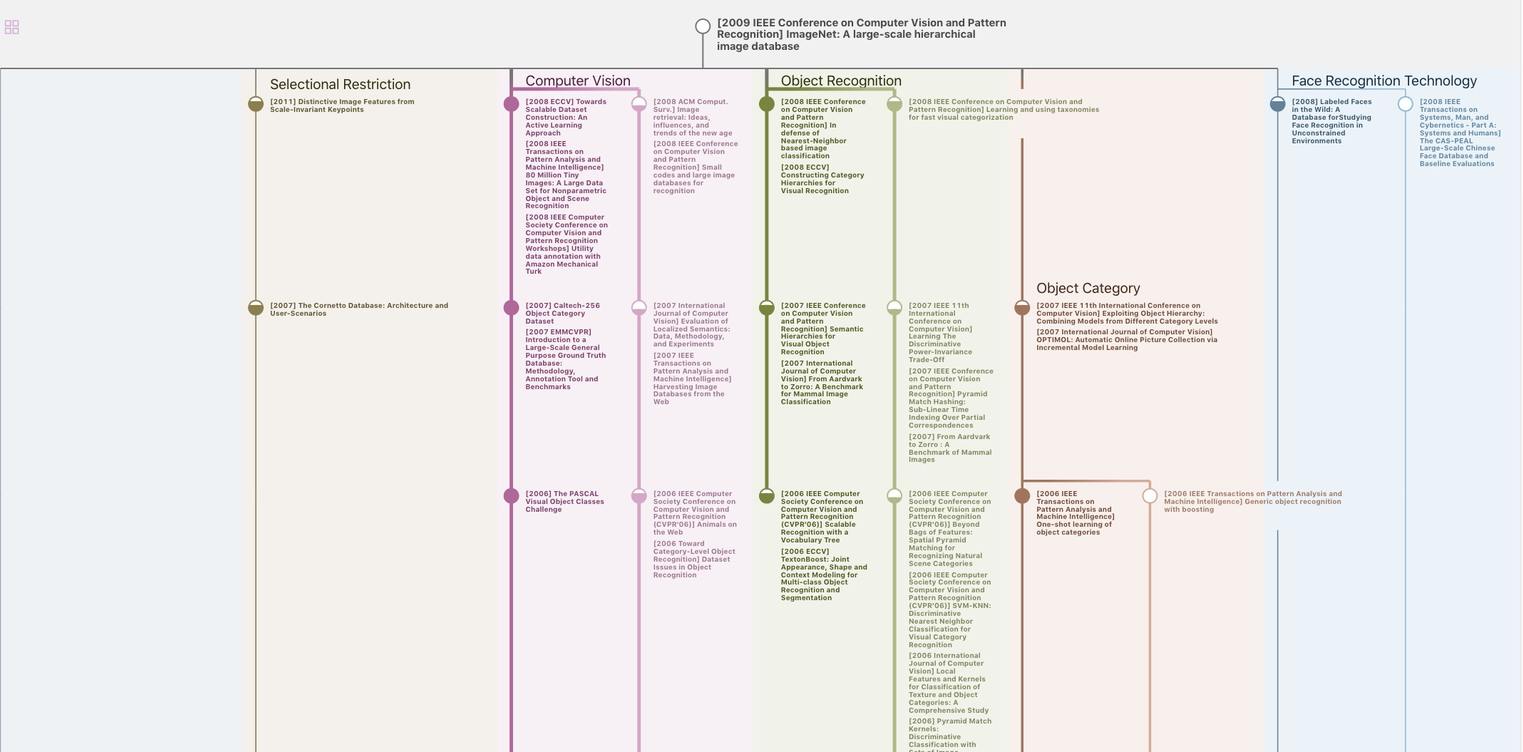
生成溯源树,研究论文发展脉络
Chat Paper
正在生成论文摘要