Amortized Bayesian Parameter Estimation Approach for WECC Composite Load Model
IEEE TRANSACTIONS ON POWER SYSTEMS(2024)
摘要
Calibrating the composite load model with distributed generation (CMPLDWG) is of a great challenge due to the presence of high-dimension parameters. This paper proposes an amortized Bayesian parameter estimation method for the CMPLDWG model. A prior distribution restrictor is developed via a classifier to filter out invalid simulations because of the unsuitable parameter combinations during the offline training stage. A sparse polynomial chaos expansion (SPCE) enabled global sensitivity analysis is also developed to identify the importance of each parameter's response to the captured system dynamics. This allows us to narrow down the problematic parameters and reduce the problem's complexity. Finally, the multi-fidelity trajectory statistics constructor is proposed to extract the representations from post-disturbance dynamic responses. The representations are further used by conditional masked autoregressive flow (CMAF) to learn the parameter posterior distribution. Comparative results with state-of-the-art approaches on the IEEE 39-bus power system demonstrate the high accuracy and robustness of the proposed method.
更多查看译文
关键词
Dynamic load modeling,global sensitivity analysis,Bayesian statistics,conditional masked autoregressive flow
AI 理解论文
溯源树
样例
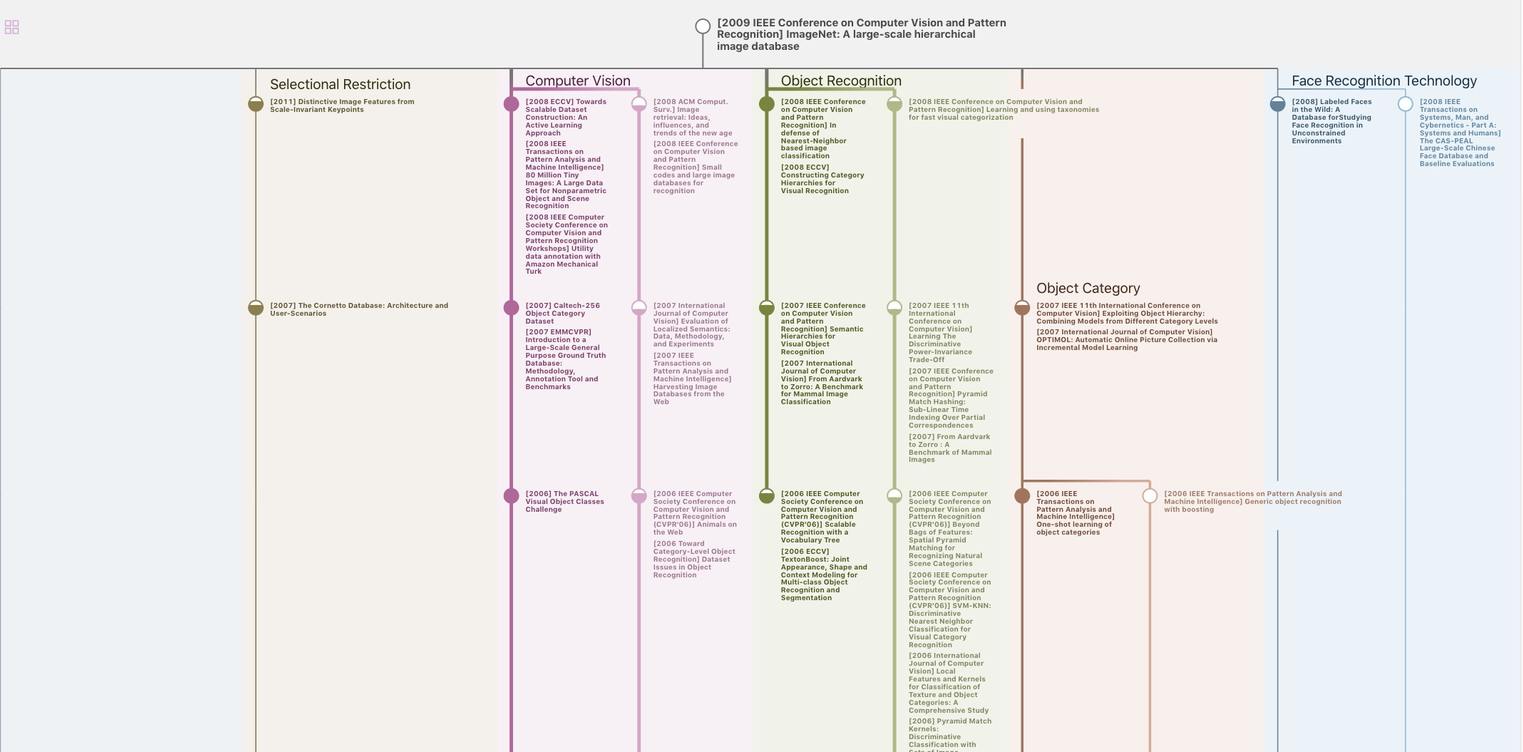
生成溯源树,研究论文发展脉络
Chat Paper
正在生成论文摘要