Complex Event Summarization Using Multi-Social Attribute Correlation
IEEE Transactions on Knowledge and Data Engineering(2023)
摘要
Complex social event summarization is a problem which has been shown having great utility for real-world applications, including crisis management, rumor control and government policy tracking. In recent years there has been significant research effort spent on effectively extracting meaningful textual descriptions of an event. However, in many critical situations, social events are complex and context-sensitive, which demands the online summarization of social events in an integrated manner. In this paper, we propose the first online complex social event summarization approach, namely SOMA, which summarizes the complex social events over multiple attributes including media content and contexts simultaneously. Specifically, we first propose a deep learning model that comprehensively summarizes events in regards to the text description and locations that they appear in, by utilizing their hidden connections in posts. We then propose a summary generator over time, text and location to achieve a maximal coverage of the summary over the original social event and minimal redundancy of the summary. Furthermore, we propose a location estimation method to address the location sparsity issue of complex events by mining the correlation between text and location. The evaluation over four real-event datasets and three benchmark datasets shows that our proposed approach outperforms the existing solutions for event summarizaiton in terms of effectiveness and efficiency.
更多查看译文
关键词
Event summarization,location sparsity,social media
AI 理解论文
溯源树
样例
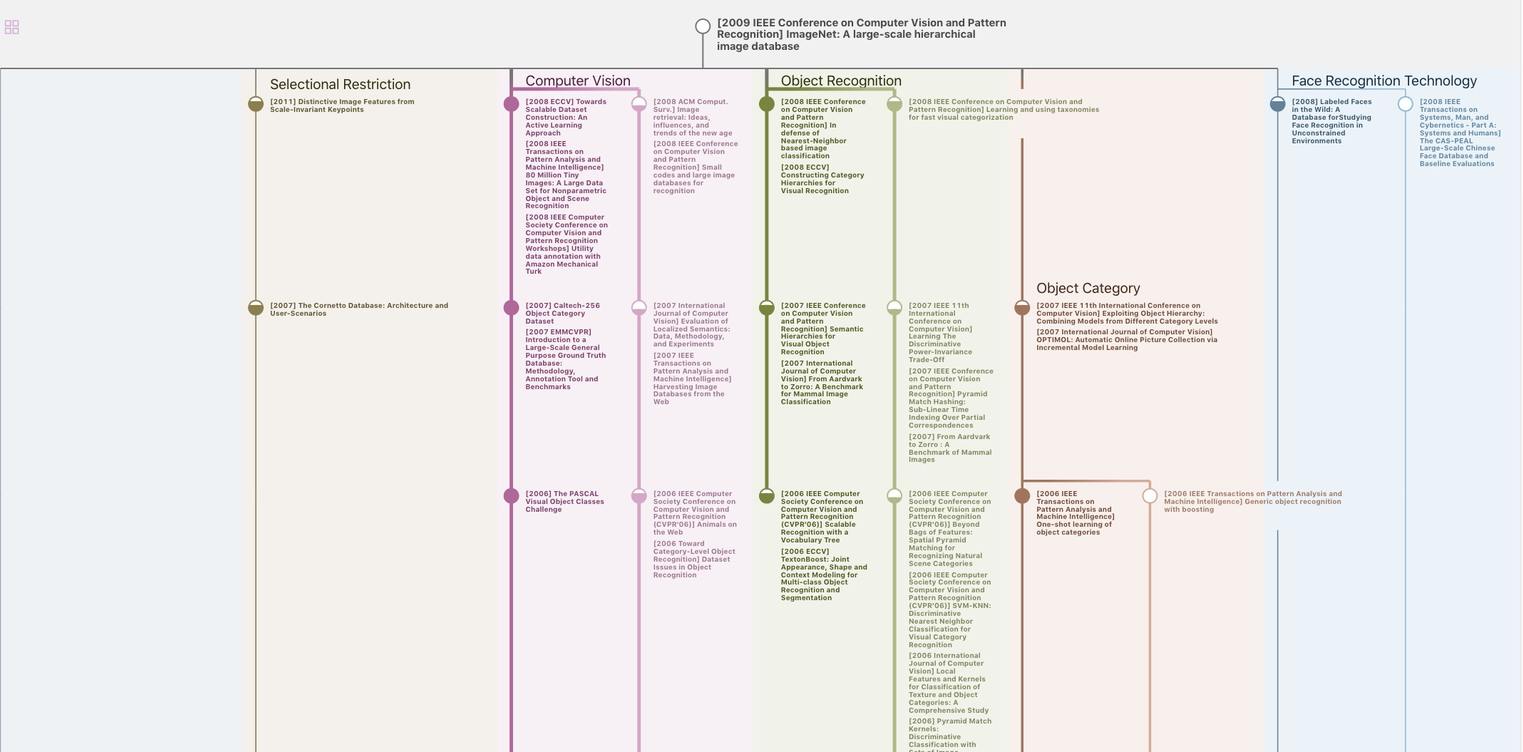
生成溯源树,研究论文发展脉络
Chat Paper
正在生成论文摘要