Personalization Through User Attributes for Transformer-Based Sequential Recommendation
Lecture Notes in Electrical EngineeringRecommender Systems in Fashion and Retail(2023)
摘要
Sequential recommendation models are able to learn user preferences based solely on the sequences of past user-item interactions. However, there usually exist additional features about the items as well as the user that can be exploited to improve themodel performance. Various models have already successfully exploited additional item information, but as fashion taste, for example, is really dependent on the individual person, characterizing the consumer becomes even more important for recommendations. The integration of user attributes into sequential item recommendation models has received far less attention, although user attributes can alleviate the cold-start problem to some degree through providing general preferences of the user at the start of a session. To address this, we propose a way to fuse user attributes directly at the item representation layer and compare this to a pre- and postfusion baseline. To test our approach, we adapt the well-known model BERT4Rec to use these fused user-item representations as input. We also experiment with a unidirectional transformer model inspired by SASRec. We evaluate all models on a real-world dataset from a fashion e-commerce shop as well as on the public Movielens-1m dataset. Our results show that the integration of user attributes improves the recommendation results for BERT4Rec for next item prediction and can also leverage the user information for recommendations in case of a cold-start setting.
更多查看译文
关键词
Sequential recommendation,User attributes,Item recommendation
AI 理解论文
溯源树
样例
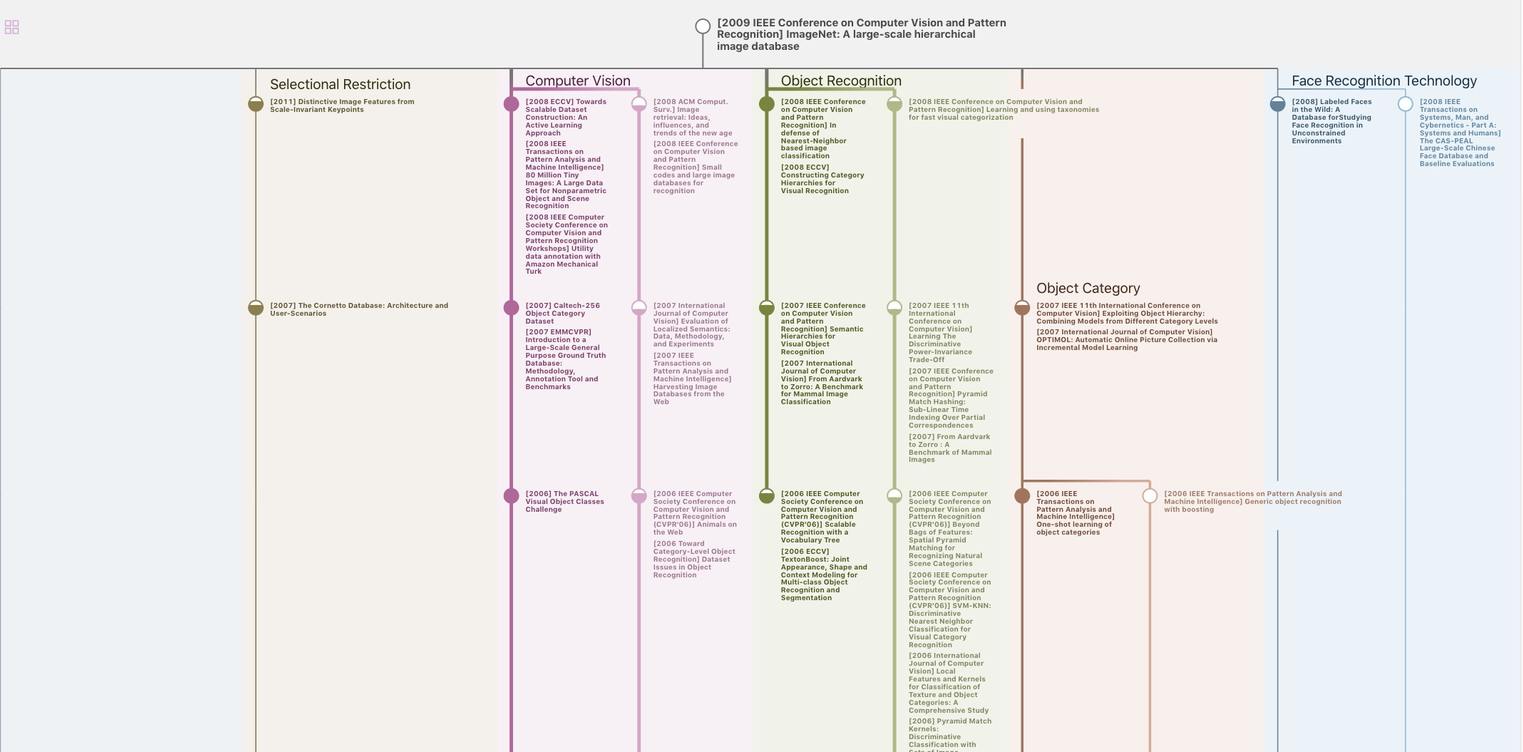
生成溯源树,研究论文发展脉络
Chat Paper
正在生成论文摘要