A physics-based neural network reconstruction of the dense matter equation of state from neutron star observables
EPJ Web of Conferences(2023)
摘要
We introduce a novel technique that utilizes a physics-driven deep learning method to reconstruct the dense matter equation of state from neutron star observables, particularly the masses and radii. The proposed framework involves two neural networks: one to optimize the EoS using Automatic Differentiation in the unsupervised learning scheme; and a pre-trained network to solve the Tolman–Oppenheimer–Volkoff (TOV) equations. The gradient-based optimization process incorporates a Bayesian picture into the proposed framework. The reconstructed EoS is proven to be consistent with the results from conventional methods. Furthermore, the resulting tidal deformation is in agreement with the limits obtained from the gravitational wave event, GW170817.
更多查看译文
关键词
dense matter equation,neural network reconstruction,neutron,neural network,physics-based
AI 理解论文
溯源树
样例
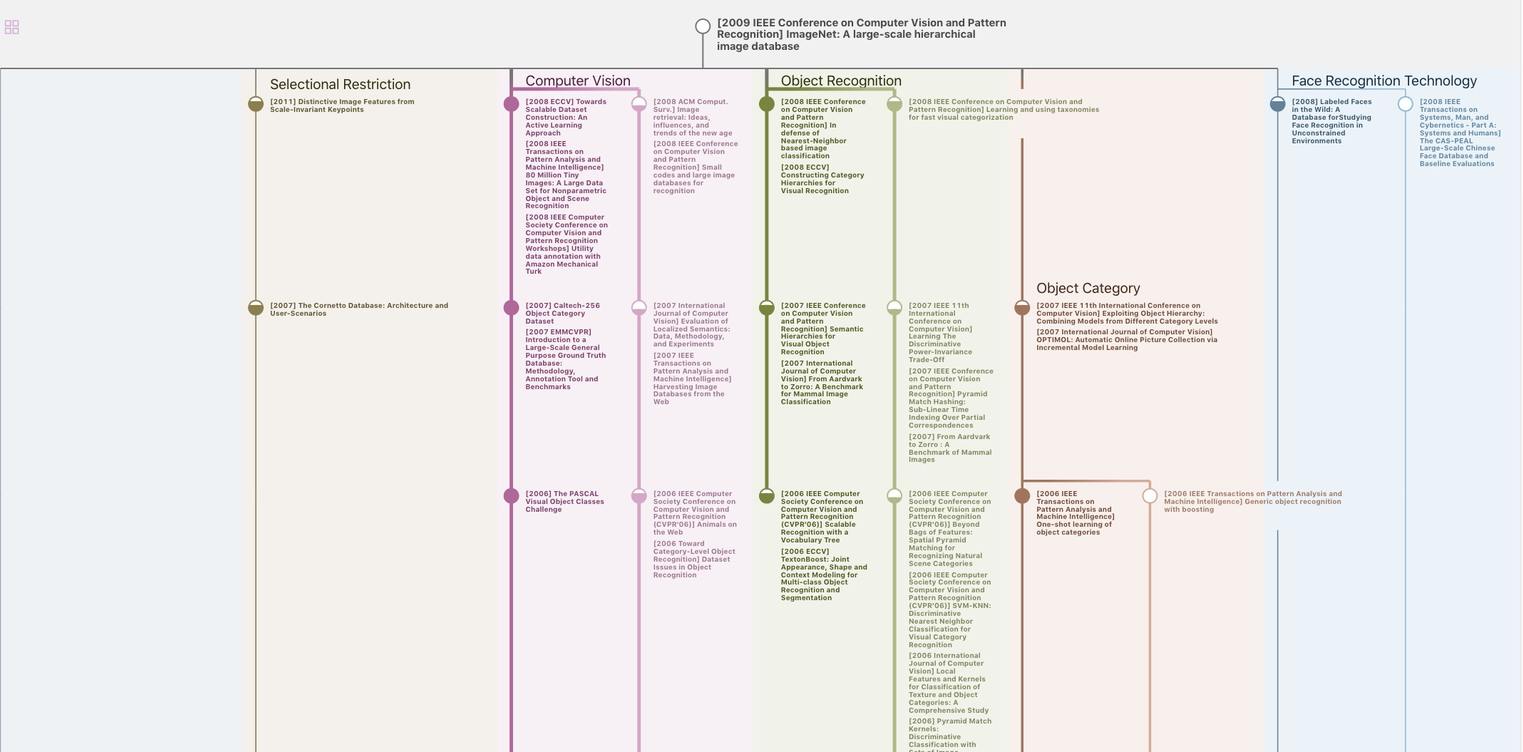
生成溯源树,研究论文发展脉络
Chat Paper
正在生成论文摘要