Low-Dimensional Space Modeling-Based Differential Evolution for Large-Scale Global Optimization Problems
IEEE Transactions on Evolutionary Computation(2023)
摘要
Large-scale global optimization (LSGO) has been an active research field. Part of this interest is supported by its application to cutting-edge research, such as Deep Learning, Big Data, and complex real-world problems, such as image encryption, real-time traffic management, and more. However, the high dimensionality makes solving LSGO a significant challenge. Some recent research deal with the high dimensionality by mapping the optimization process to a reduced alternative space. Nonetheless, these works suffer from the changes in the search space topology and the loss of information caused by the dimensionality reduction. This article proposes a hybrid metaheuristic, so-called low-dimensional space modeling-based differential evolution (LSMDE), that uses the singular value decomposition to build a low-dimensional search space from the features of candidate solutions generated by a new SHADE-based algorithm (GM-SHADE). GM-SHADE combines a Gaussian mixture model (GMM) and two specialized local algorithms: 1) MTS-LS1 and 2) L-BFGS-B, to promote a better exploration of the reduced search space. GMM mitigates the loss of information in mapping high-dimensional individuals to low-dimensional individuals. Furthermore, the proposal does not require prior knowledge of the search space topology, which makes it more flexible and adaptable to different LSGO problems. The results indicate that LSMDE is the most efficient method to deal with partially separable functions compared to other state-of-the-art algorithms and has the best overall performance in two of the three proposed experiments. Experimental results also show that the new approach achieves competitive results for nonseparable and overlapping functions on the most recent test suite for LSGO problems.
更多查看译文
关键词
Differential evolution (DE),dimensionality reduction,Gaussian mixture model (GMM),singular value decomposition (SVD)
AI 理解论文
溯源树
样例
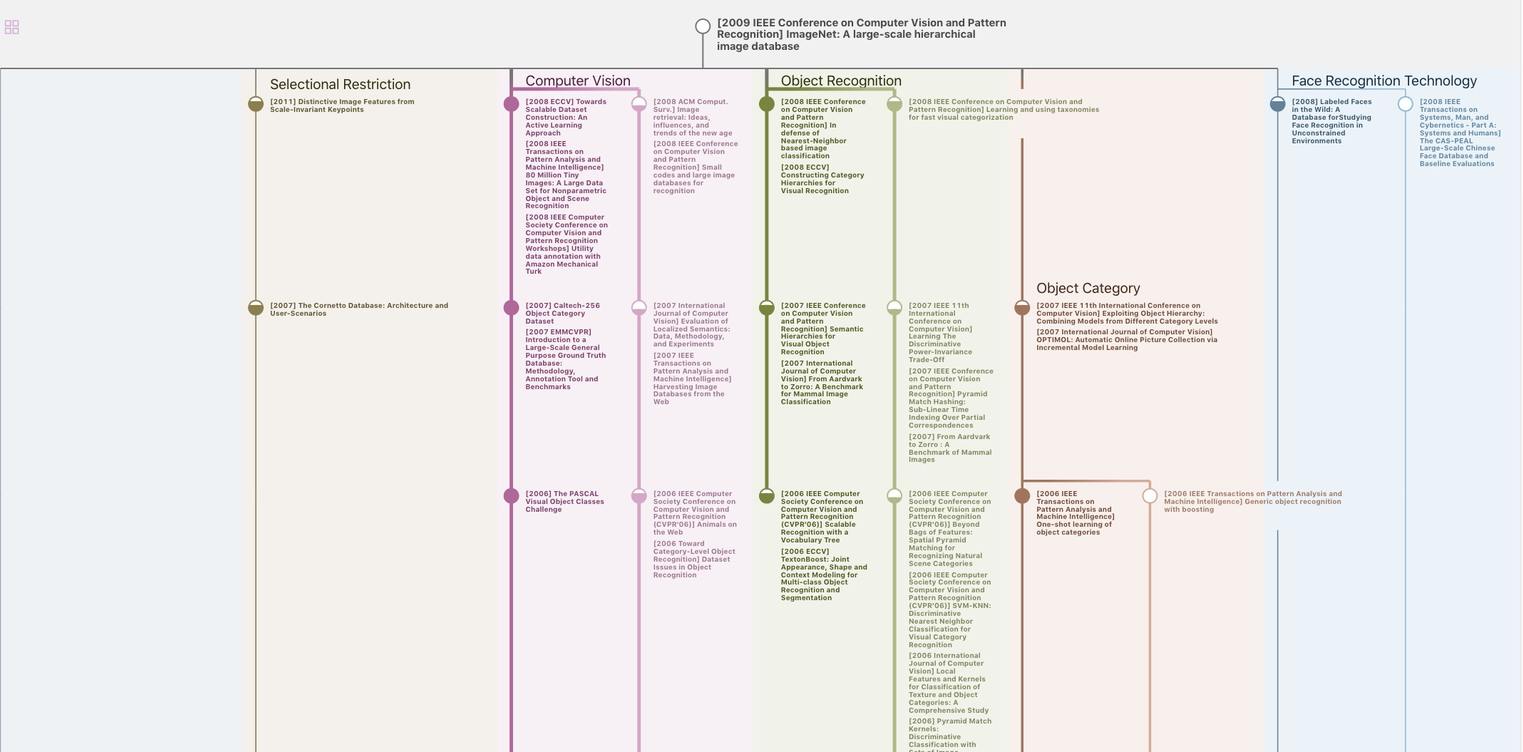
生成溯源树,研究论文发展脉络
Chat Paper
正在生成论文摘要