Matching with Multilevel Data
Handbook of Matching and Weighting Adjustments for Causal Inference(2023)
摘要
A common feature of many data sets in the biomedical and social sciences is what is known as multilevel data structure. In a multilevel data structure, units are nested in one or more clusters and measurements are taken at both the unit and cluster level. Educational settings are perhaps the most well-known multilevel structure, in which we observe student level measures such as gender, but also school level covariates such as the proportion of female students and the size of the school [1]. Other multilevel data structures include patients within hospitals, households within villages, and firms within industries. Often, we are interested in the effects of a treatment administered at the group level on individual level outcomes. For example, a study may focus on the effect of a school level treatment on student level outcomes. A conventional approach to estimating treatment effects with multilevel data relies on hierarchical regression models. In this chapter, we review how matching methods can be applied to data with a multilevel structure. As we outline, the application of matching to multilevel data depends critically on the treatment assignment mechanism. Different treatment assignment mechanisms lead to very different applications of matching with multilevel data. In what follows we describe two primary classes of multilevel observational studies, those with treatment assignment at the individual level, and those with treatment at the cluster level, the latter class designated as clustered observational studies (COSs). We briefly review matching in settings with individualistic treatment assignment, which is closely related to existing matching methods for single-level data. The balance of the chapter is devoted to a specific form of matching tailored to clustered treatment assignment, where two matches are typically performed within the same dataset, one at the cluster level and one at the individual. We also discuss implications for inference, sensitivity analysis, and open problems in this general area.
更多查看译文
关键词
multilevel data
AI 理解论文
溯源树
样例
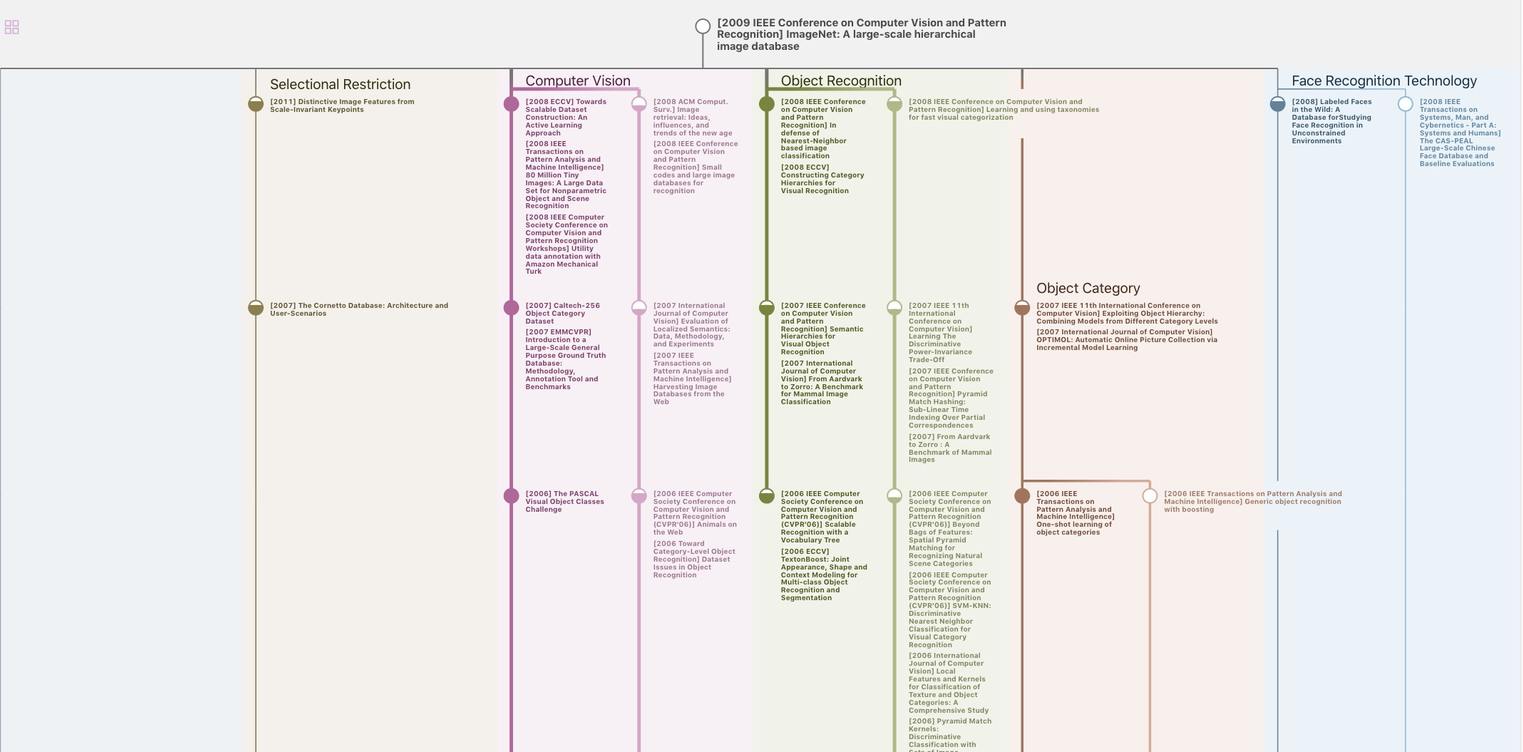
生成溯源树,研究论文发展脉络
Chat Paper
正在生成论文摘要