Research on P2P Default Risk Prediction Based on Logistic Regression
2022 4th International Conference on Intelligent Information Processing (IIP)(2022)
摘要
With the rapid development of the Internet, a variety of traditional industries use information technology and Internet platform closely derived from a variety of emerging industries. In the financial field, traditional banks cannot meet the needs of small and medium-sized enterprises with rapid growth. Thanks to the mature development of Internet platforms, various P2P(Peer to Peer Lending) platforms have emerged as the times require. They provide a simple reception approval process and provide investors and borrowers with convenient lending channels. However, with the rapid development of the P2P industry, P2P has frequent security problems. The main reason for this phenomenon is that the personal credit evaluation of the borrower is inaccurate, which leads to a chain reaction. Therefore, the effective estimation of the credit data of the borrower is the key to solving the credit risk. With the rapid development of machine learning, various risk prevention and control models are more and more accurate in predicting personal credit. However, default data accounts for only a small part of the massive credit data, so it is particularly important to process the data set, especially to balance the sample data. Traditionally, SMOTE(Synthetic Minority Oversampling Technique) algorithm is generally used for oversampling of data. However, when there are noise samples in the auxiliary samples of the samples synthesized by this algorithm, the new samples may become the noise sample interference model. In this paper, the Borderline-SMOTE algorithm is used to oversample the data features. The results show that the model trained by the processed data set is better than the control model, both in accuracy and recall rate.
更多查看译文
关键词
P2P,Credit Prediction,LR-Borderline-SMOTE,Gradient Descent
AI 理解论文
溯源树
样例
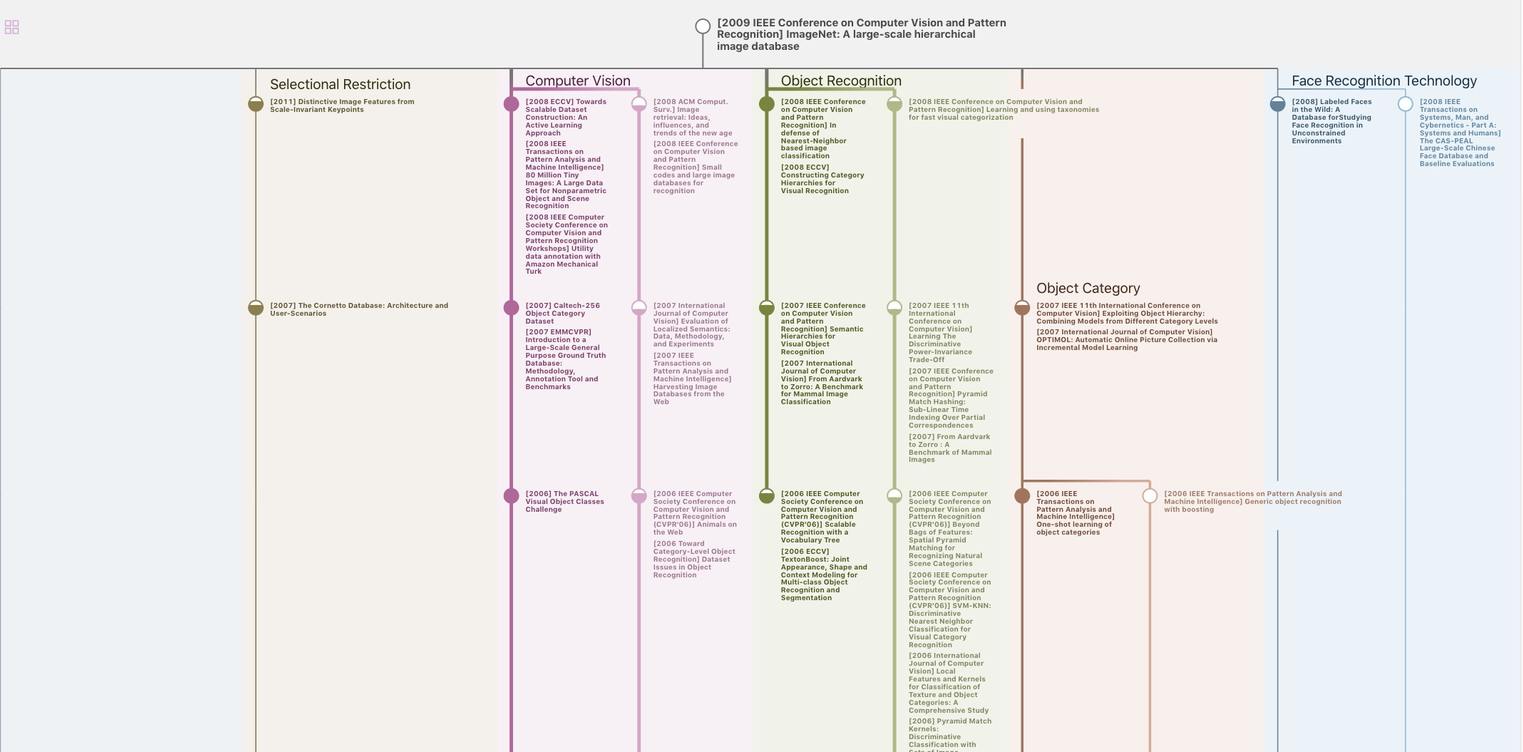
生成溯源树,研究论文发展脉络
Chat Paper
正在生成论文摘要