Benchmark of the high-resolution Nemo-SI3-XIOS configuration SEDNA on an ARM-based HPC system, Fugaku.
crossref(2023)
摘要
<p>We have developed the ocean-sea ice Arctic regional configuration <strong>SEDNA</strong> (Sea ice - EDdy resolving ocean paN-Arctic configuration) at ultra-high resolution (800 m in the horizontal and 150 vertical levels) based on the NEMO-SI3 numerical platform, in order to investigate how the dynamics of mesoscale turbulent eddies in the Arctic Ocean interplay with sea ice. </p><p>The configuration was initially developed on the <strong>AMD-</strong>based HPC system <strong>Joliot-Curie ROME </strong>based at CEA in France<strong>, </strong>which has achieved a Linpack performance of 12 PFlop/s ranked number 33 of June 2020 TOP500 list (https://top500.org/system/179700/).</p><p>Thanks to a European PRACE allocation of nearly 40 million CPU hours, we were able to run a 8 year-long simulation. Although promising to understand part of the small-scale dynamics at play, this length of simulation will likely be a limiting factor in the investigation of the eddy dynamics which is known to equilibrate over several decades.</p><p>To overcome this limitation, here we investigate the feasibility of running SEDNA over several decades but at a realistic time cost. To that aim, a benchmark has been performed on the <strong>ARM</strong> based HPC system <strong>Fugaku </strong>based at RIKEN in Japan, which has archived a Linpack performance of 442 PFlop/s ranked number 1 of November 2020 TOP500 list (https://www.top500.org/system/179807/). Such a benchmark has required adaptation of the compiling and placements of NEMO and XIOS MPI processes in order to fit on the Fugaku architecture compared to standard X86_64 based HPC systems like Joliot-Curie ROME. In this presentation we will share the tips and lessons learned from our benchmarks and will report the benchmark results.  Our insights on model MPI placements for efficient post processing of huge models will be discussed as well.</p>
更多查看译文
AI 理解论文
溯源树
样例
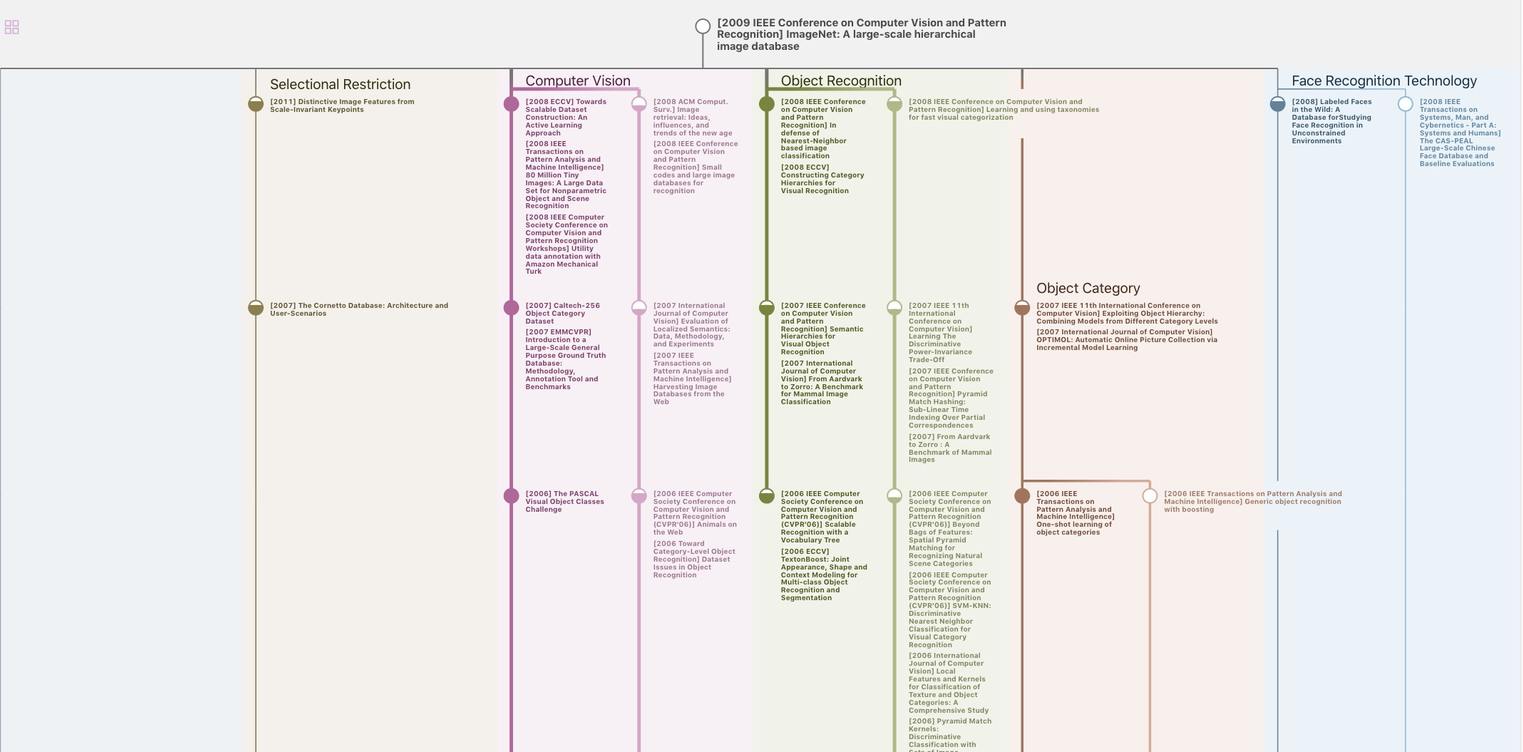
生成溯源树,研究论文发展脉络
Chat Paper
正在生成论文摘要