A VAE-based Model for Incomplete Time Series Modeling using a Time-aware Encoder and Neural ODEs
Research Square (Research Square)(2022)
摘要
Abstract Internet of Things (IoT) realizes the ubiquitous connection of all things, sensors of which produces countless data with time tags. Quality of time series data become increasing important when effectively mined to inform decision making towards solving practical problems. However, time series are often incomplete as a result of missing values or being sampled at irregular frequencies. Yet, traditional algorithms fail to directly model such incomplete time series data. The preprocess of missing data imputing destroys time step information, and the deviation data will additionally bring error accumulation to the subsequent model. To this end, this paper presents an improved variational autoencoder (VAE) model coupled with a time-aware LSTM encoder and neural ordinary differential equations (ODEs) for incomplete time data modeling, which is named the Time-Recurrent Variational AutoEncoder ODE (TRVAEODE) model. In this model, continuous-time latent dynamics are generated by Neural ODEs based on the initial state learned by the time-aware LSTM encoder from partial observed data, further enabling conduct data imputation and prediction with higher accuracy. Furthermore, the proposed TRVAEODE model is evaluated on incomplete real-world and synthetic time-series datasets by conducting data interpolation and extrapolation tasks as well as classification task. Extensive experiments show the TRVAEODE model outperforms the baseline methods in terms of Mean Square Error (MSE) in tasks of imputation and prediction as well as accuracy in downstream classification task.
更多查看译文
关键词
incomplete time series modeling,vae-based,time-aware
AI 理解论文
溯源树
样例
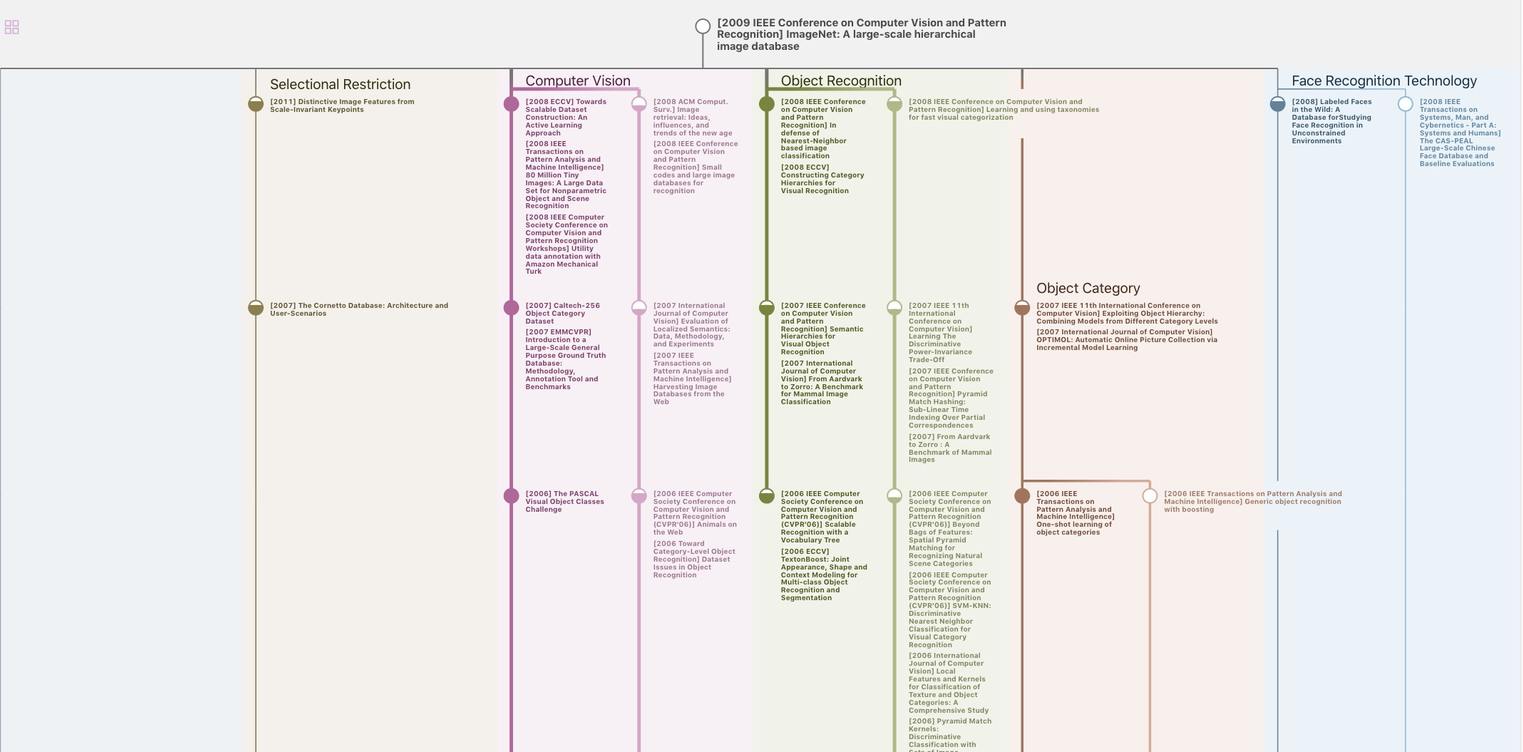
生成溯源树,研究论文发展脉络
Chat Paper
正在生成论文摘要