Extracting Electricity Patterns from High-dimensional Data: A comparison of K-Means and DBSCAN algorithms
IOP Conference Series: Earth and Environmental Science(2022)
摘要
Abstract Environmental sustainability has become a priority for an increasing number of Australian cities in recent years. As a smart distribution platform, Virtual Power Plant (VPP) is a promising approach to achieving urban sustainability. Understanding local conditions, such as user electricity demand profile, is one of the most important aspects to modelling a VPP platform. However, due to data privacy and confidentiality, in many cases, energy demand profile has limitations. This study demonstrates how clustering techniques can support the development of electricity patterns from high-dimensional data, thereby inform VPP modelling. This study analysed historical electricity consumption data from an Australian city by using two clustering algorithms, K-Means and DBSCAN. According to the experiment results, both algorithms have their advantages and disadvantages, and the optimal algorithm choice is highly dependent on the clustering purpose. Both algorithm are not human iterpratable dealing with such high-dimensional dataset. In VPP modelling, the electricity patterns generated by clustering analyses can be used as input data to train Reinforcement Learning agents to learn human behaviours and rationality.
更多查看译文
关键词
electricity patterns,dbscan algorithms,data,high-dimensional,k-means
AI 理解论文
溯源树
样例
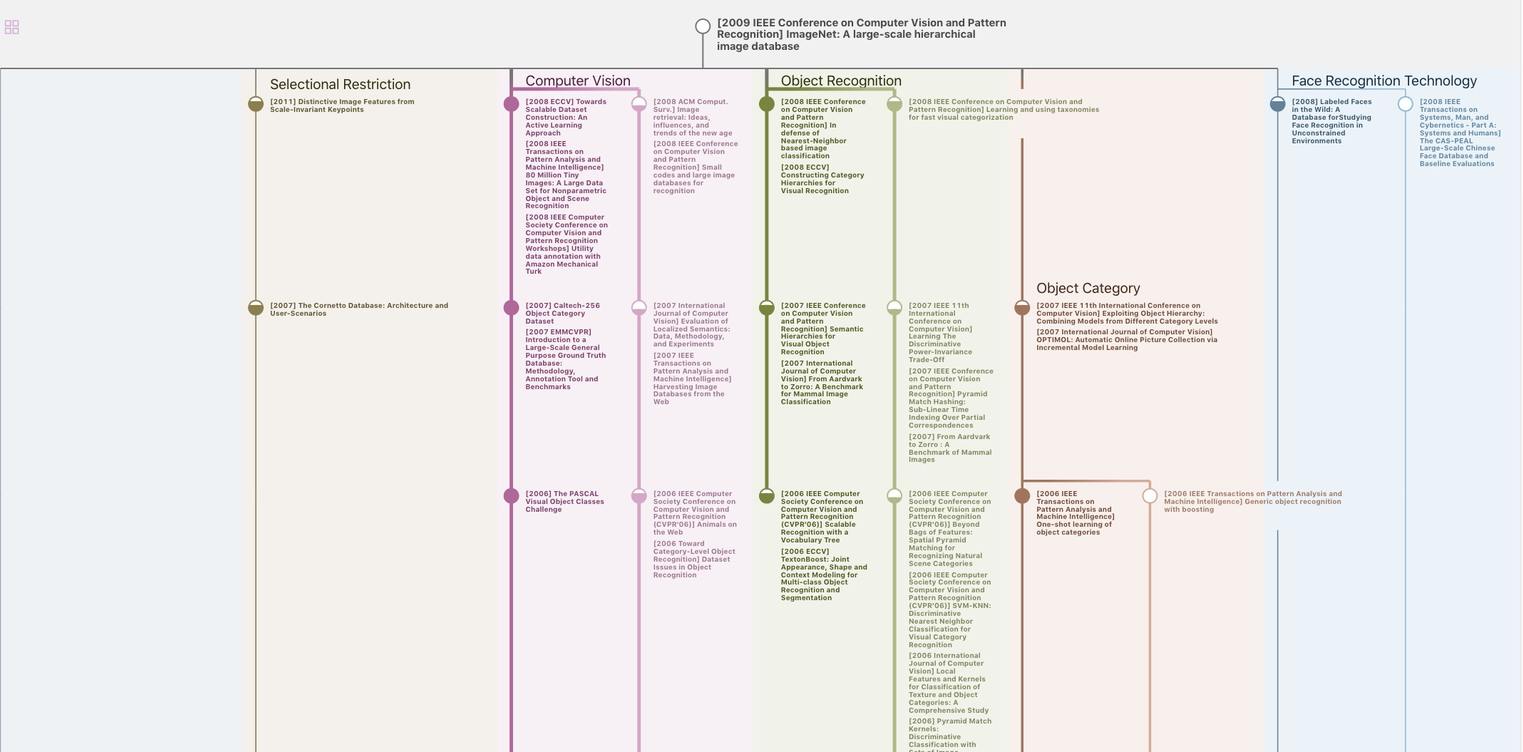
生成溯源树,研究论文发展脉络
Chat Paper
正在生成论文摘要