Pagml: Precise Alignment Guided Metric Learning for Sketch-Based 3d Shape Retrieval
SSRN Electronic Journal(2023)
摘要
Sketch-based 3D shape retrieval has always been a hot research topic in the computer vision community. The main challenge is to alleviate the cross-modality discrepancies such that the retrieval accuracies can be improved. In this paper, we propose a novel Precise Alignment Guided Metric Learning (PAGML) method based on master-auxiliary cross-modality retrieval framework. An auxiliary learning network is developed to indirectly guide the master learning model to extract features of rich semantic information, so as to achieve a semantic alignment between the cross-modality data. Furthermore, affected by the intra-class variability and inter-class imbalance issue, the learned class distributions may exhibit unevenness in the common embedding space and cause poor retrieval performance. A loss function dedicated for cross-modality retrieval is designed to achieve a rigid alignment between sketches and 3D shapes of the same category by pulling their rich semantic represen-tations to the rigid center of the category. As a result, a more precise alignment between the cross-modality embedding features of the same category is approached gradually, which further alleviates the cross-modality discrepancies, inter-class variability, and inter-class imbalance, thus improving the cross-modality retrieval ac-curacies. Extensive experiments on two public benchmark datasets demonstrate that the proposed PAGML sur -passes the state-of-the-art methods in retrieval accuracy and has excellent generalization abilities to unseen classes.
更多查看译文
关键词
Sketch-based 3D shape retrieval,Cross-modality retrieval,Master-auxiliary network,Triplet rigid center loss,Precise alignment
AI 理解论文
溯源树
样例
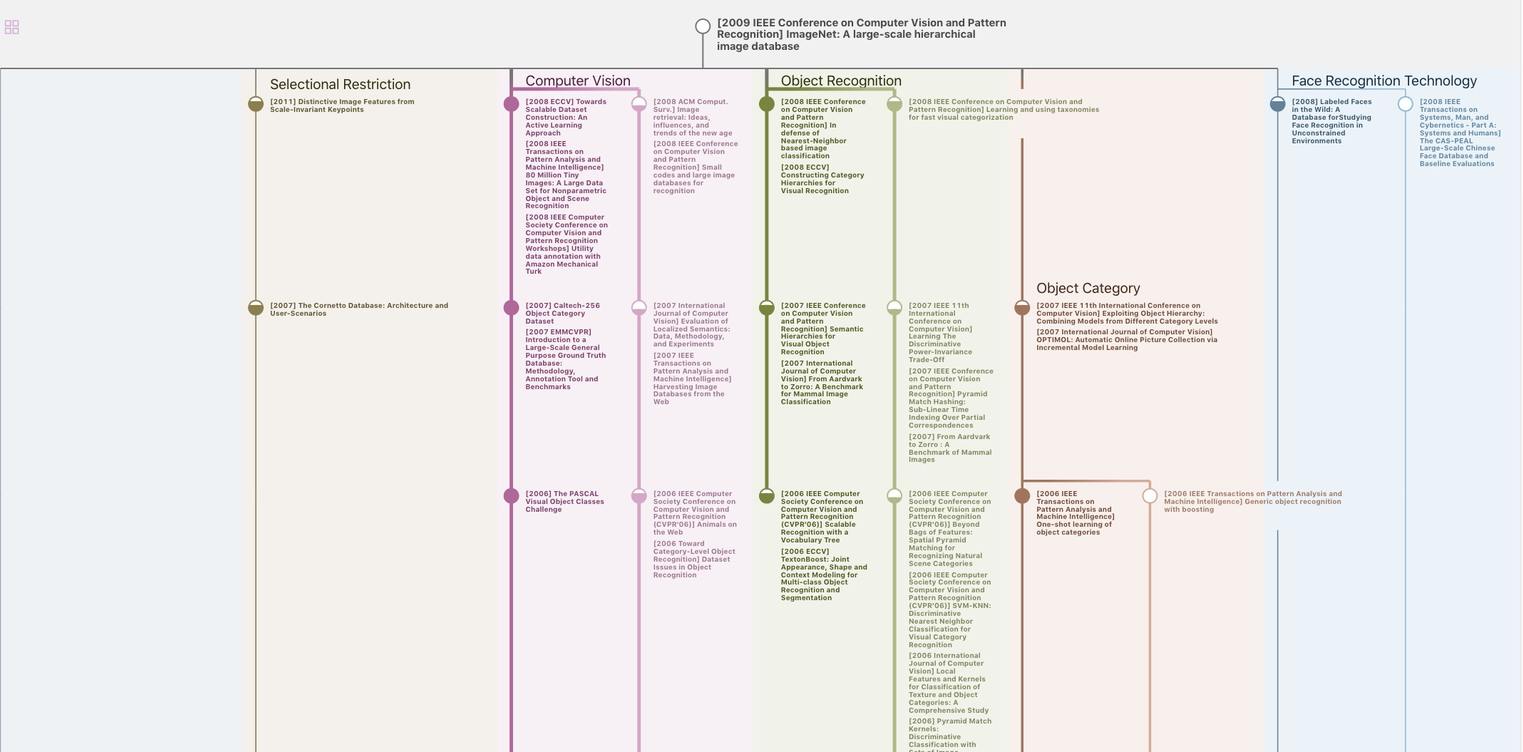
生成溯源树,研究论文发展脉络
Chat Paper
正在生成论文摘要