Attention-Based Knowledge Distillation in Scene Recognition: The Impact of a DCT-Driven Loss
IEEE Transactions on Circuits and Systems for Video Technology(2023)
摘要
Knowledge Distillation (KD) is a strategy for the definition of a set of transferability gangways to improve the efficiency of Convolutional Neural Networks. Feature-based Knowledge Distillation is a subfield of KD that relies on intermediate network representations, either unaltered or depth-reduced via maximum activation maps, as the source knowledge. In this paper, we propose and analyze the use of a 2D frequency transform of the activation maps before transferring them. We pose that—by using global image cues rather than pixel estimates, this strategy enhances knowledge transferability in tasks such as scene recognition, defined by strong spatial and contextual relationships between multiple and varied concepts. To validate the proposed method, an extensive evaluation of the state of the art in scene recognition is presented. Experimental results provide strong evidence that the proposed strategy enables the student network to better focus on the relevant image areas learnt by the teacher network, hence leading to better descriptive features and higher transferred performance than every other state-of-the-art alternative. We publicly release the training and evaluation framework used in this paper at
https://www-vpu.eps.uam.es/publications/DCTBasedKDForSceneRecognition
.
更多查看译文
关键词
Knowledge distillation, multi-attention, 2D frequency transform, scene recognition, deep learning, convolutional neural networks
AI 理解论文
溯源树
样例
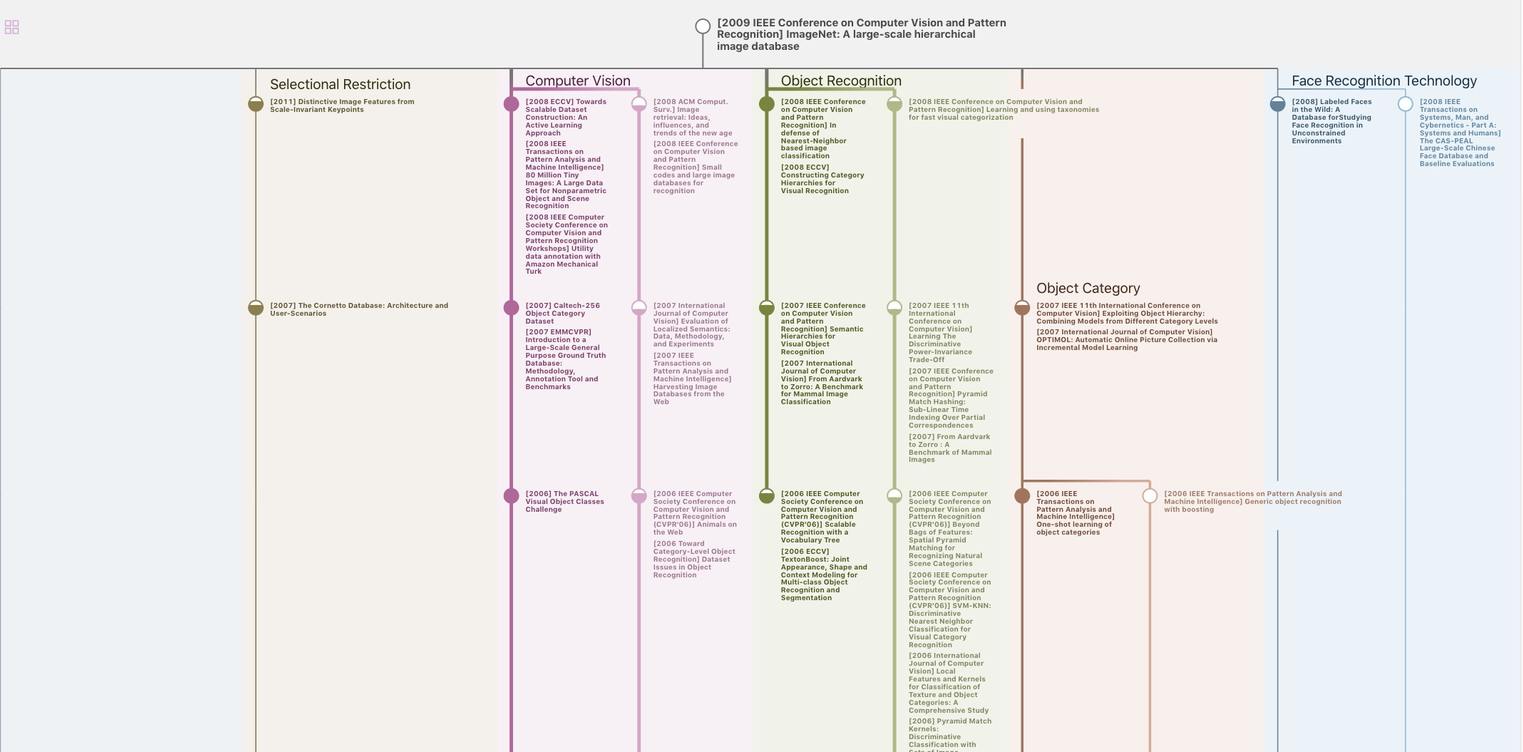
生成溯源树,研究论文发展脉络
Chat Paper
正在生成论文摘要