ORT: Occlusion-robust for multi-object tracking
Fundamental Research(2023)
摘要
Although the joint-detection-and-tracking paradigm has promoted the development of multi-object tracking (MOT) significantly, the long-term occlusion problem is still unsolved. After a period of trajectory inactivation due to occlusion, it is difficult to achieve trajectory reconnection with appearance features because they are no longer reliable. Although using motion cues does not suffer from occlusion, the commonly used Kalman Filter is also ineffective in its long-term inertia prediction in cases of no observation updates or wrong updates. Besides, occlusion is prone to cause multiple track-detection pairs to have close similarity scores during the data association phase. The direct use of the Hungarian algorithm to give the global optimal solution may generate the identity switching problem. In this paper, we propose the Long-term Spatio-Temporal Prediction (LSTP) module and the Ordered Association (OA) module to alleviate the occlusion problem in terms of motion prediction and data association, respectively. The LSTP module estimates the states of all tracked objects over time using a combination of spatial and temporal Transformers. The spatial Transformer models crowd interaction and learns the influence of neighbors, while the temporal Transformer models the temporal continuity of historical trajectories. Besides, the LSTP module also predicts the visibilities of the motion prediction boxes, which denote the occlusion attributes of trajectories. Based on the occlusion attribute and active state, the association priority is defined in the OA module to associate trajectories in order, which helps to alleviate the identity switching problem. Comprehensive experiments on the MOT17 and MOT20 benchmarks indicate the superiority of the proposed MOT framework, namely Occlusion-Robust Tracker (ORT). Without using any appearance information, our ORT can achieve competitive performance beyond other state-of-the-art trackers in terms of trajectory accuracy and purity.
更多查看译文
关键词
Multi-object tracking,Motion-based prediction,Trajectory reconnection,Data association
AI 理解论文
溯源树
样例
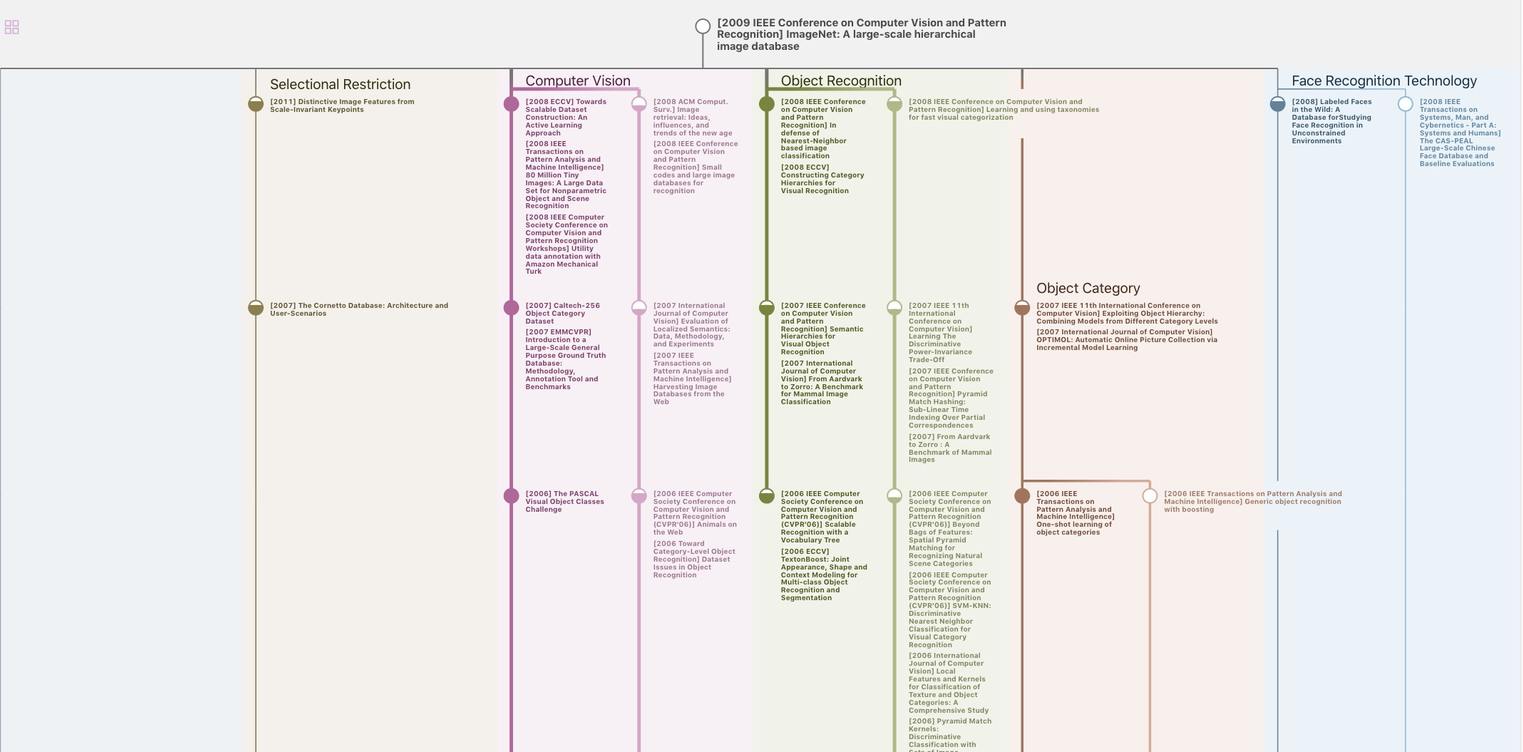
生成溯源树,研究论文发展脉络
Chat Paper
正在生成论文摘要