Tensorial principal component analysis in detecting purchase patterns in loyalty card data (Preprint)
crossref(2022)
摘要
BACKGROUND Loyalty card data automatically collected by retailers provide an excellent source for evaluating health-related purchase behaviour of customers. The data comprise information on every grocery purchase, including expenditures on products and time of purchase for each customer. Such data where customers have an expenditure value for every product for each time can be formulated as three-dimensional tensorial data. OBJECTIVE The aim of the study was to use the modern tensorial principal component analysis (tensorial PCA) method to disclose characteristics of health-related purchase patterns from loyalty card data. A further aim was to identify card holders with distinct purchase patterns. We also considered the interpretation as well as advantages and challenges of tensorial PCA compared with standard PCA. METHODS Loyalty card programme members from the largest retailer in Finland were invited to participate in the study. Our data consist of the purchases of 7251 card holders who consented to the use of their data from the year 2016. The purchases were re-classified into 55 products and aggregated across 52 weeks. The data were then analysed by tensorial PCA, allowing us to effectively reduce the time and product-wise dimensions simultaneously. The augmentation method was utilized for selecting the suitable number of principal components for the analysis. RESULTS By tensorial PCA, we were able to systematically search for typical food purchasing patterns across time and product groups as well as detect atypical behaviour across products and time, and differing purchasing behaviours across groups of card holders. For example, we identified customers who purchaseds large amounts of meat products and separated them further into groups based on time profiles, i.e. customers whose purchases of meat stayed stable, increased, or decreased throughout the year, or varied between seasons of the year. CONCLUSIONS With tensorial PCA, we can effectively examine customers’ purchasing behaviour in more detail than with traditional methods because it can handle time and product dimensions simultaneously. When interpreting the results, both time and product dimensions must be considered. In further analysis, these time and product based patterns can directly be associated with other attributes of customers. This enables us to identify what kinds of people have specific purchasing patterns, which can help in the development of ways in which consumers can be steered towards making healthier food choices.
更多查看译文
AI 理解论文
溯源树
样例
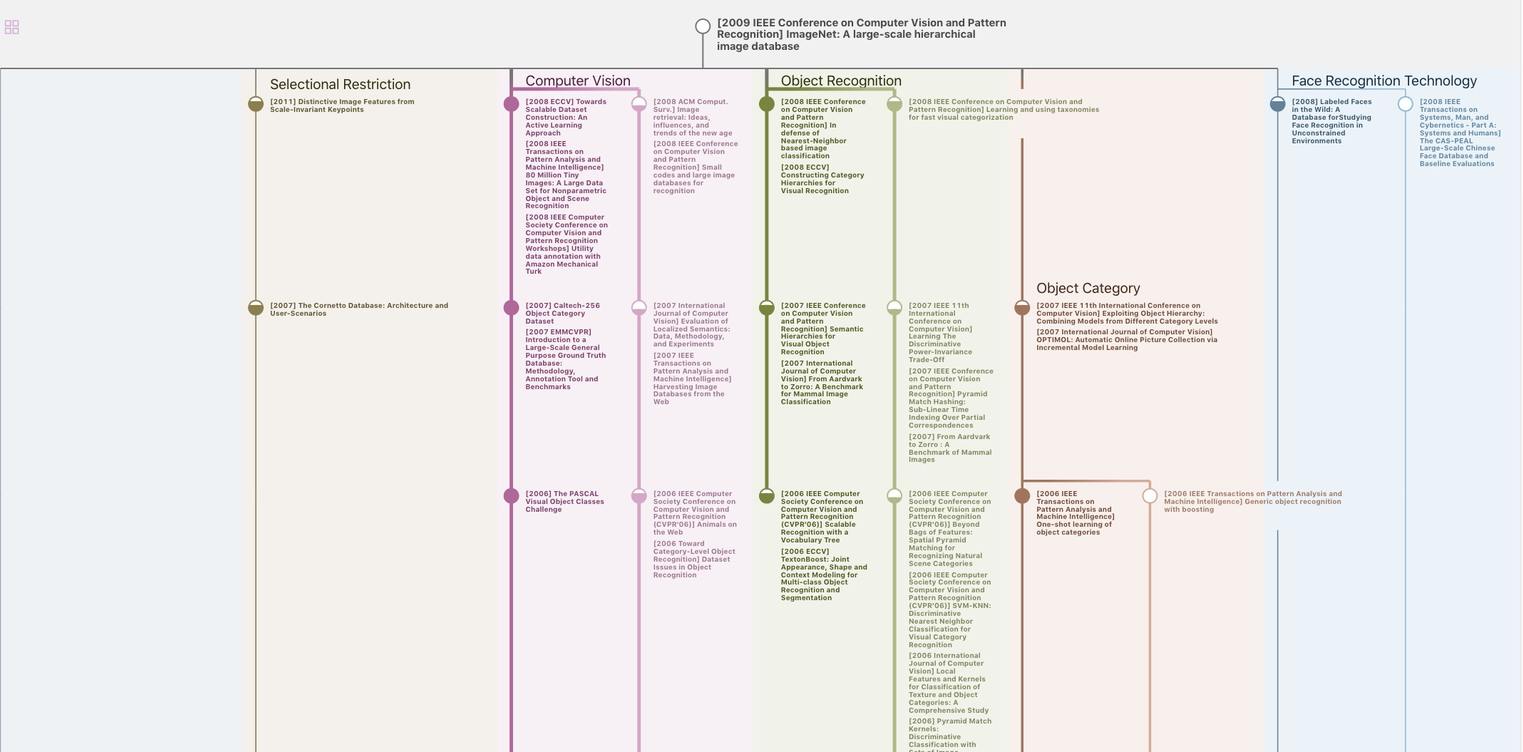
生成溯源树,研究论文发展脉络
Chat Paper
正在生成论文摘要