Enhancing the Transferability of Adversarial Examples Based on Nesterov Momentum for Recommendation Systems
IEEE TRANSACTIONS ON BIG DATA(2023)
摘要
The attacker's malicious behavior of injecting well-designed adversarial examples (i.e., fake users) into recommender systems will severely affect the security of systems. It's difficult to fully obtain details of victim recommendation models (i.e., black-box model) in practical recommendation scenarios, using the transferability of adversarial examples to achieve black-box attacks is still an effective way. At present, adversarial examples generated by existing gradient-based methods are prone to drop into local minima, making it impossible to achieve the expected attack effect and reducing the transferability. In this article, we propose an attack algorithm that enhances the transferability of adversarial examples based on the Nesterov Momentum for Recommendation Systems (ETANRS). With white-box recommendation surrogate models, we utilize Nesterov momentum to generate better adversarial examples, then inject them into black-box victim models to attack. We utilize the accumulated gradients and pre-determine the update direction of the gradients to keep the optimal value from being lost, thus enhancing the transferability of the adversarial examples. Experimental results demonstrate that our method is better than state-of-the-art gradient-based attack algorithms, which affect recommendation performance.
更多查看译文
关键词
Adversarial examples,nesterov momentum,recommendation systems,transferability
AI 理解论文
溯源树
样例
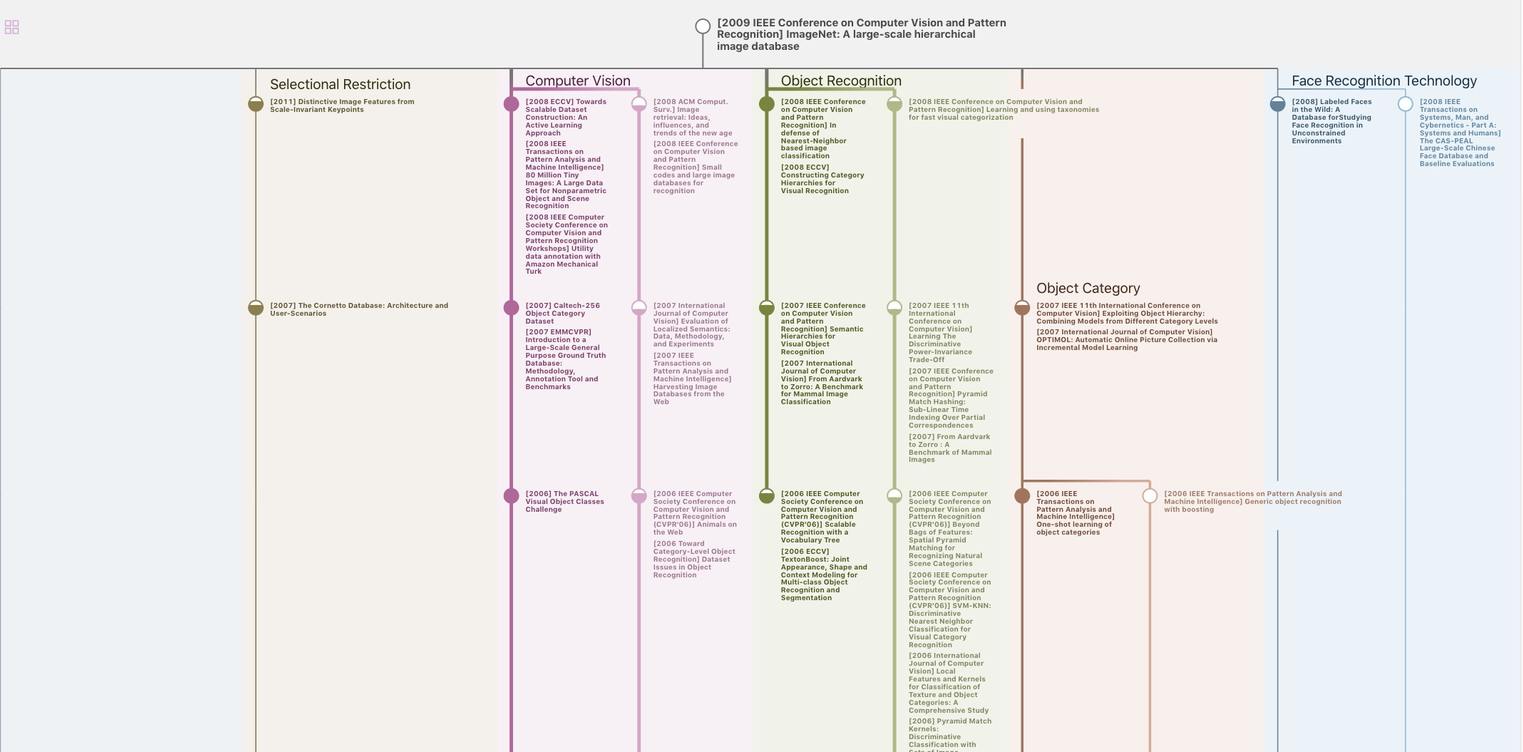
生成溯源树,研究论文发展脉络
Chat Paper
正在生成论文摘要