Estimating two key dimensions of cultural transmission from archaeological data
SSRN Electronic Journal(2023)
摘要
Cultural-evolutionary modeling of archaeological data faces numerous challenges, perhaps the most significant being the mismatch between models of microscale activities and the macroevolutionary scale of the archaeological record. This is especially the case with identifying different kinds of social learning reflected in the record. Here we present a computational approach to social learning using a new model that compares frequencies of stylistic traits through time to an evolutionary model of social learning. Two dimensions of cultural evolu-tion-popularity bias and information transparency-help unify a range of hitherto competing models of social learning. This model has never successfully been calibrated to real-world data, with the sparseness of archaeo-logical data presenting an even further challenge. By calibrating the model to archaeological data, we confirm that it can be used successfully to characterize cultural transmission in the past. Our case study consists of decorative motifs on pottery from Early Neolithic Europe, ca. 5400-5000 BCE. The comparison of data to model is highly computational, involving seven different metrics and hundreds of simulations and re-samplings. Inferences are made using approximate Bayesian computation and a random-forest algorithm to estimate the best solution using a combination of all metrics. The computational modeling confirms that cultural transmission of the Neolithic pottery motifs was a process of unbiased social learning and opens the way for the exploration of a wide range of frequency data.
更多查看译文
关键词
Bayesian estimation,Cultural transmission,Information transparency,LBK,Neutral model,Popularity bias,Social learning,Utility
AI 理解论文
溯源树
样例
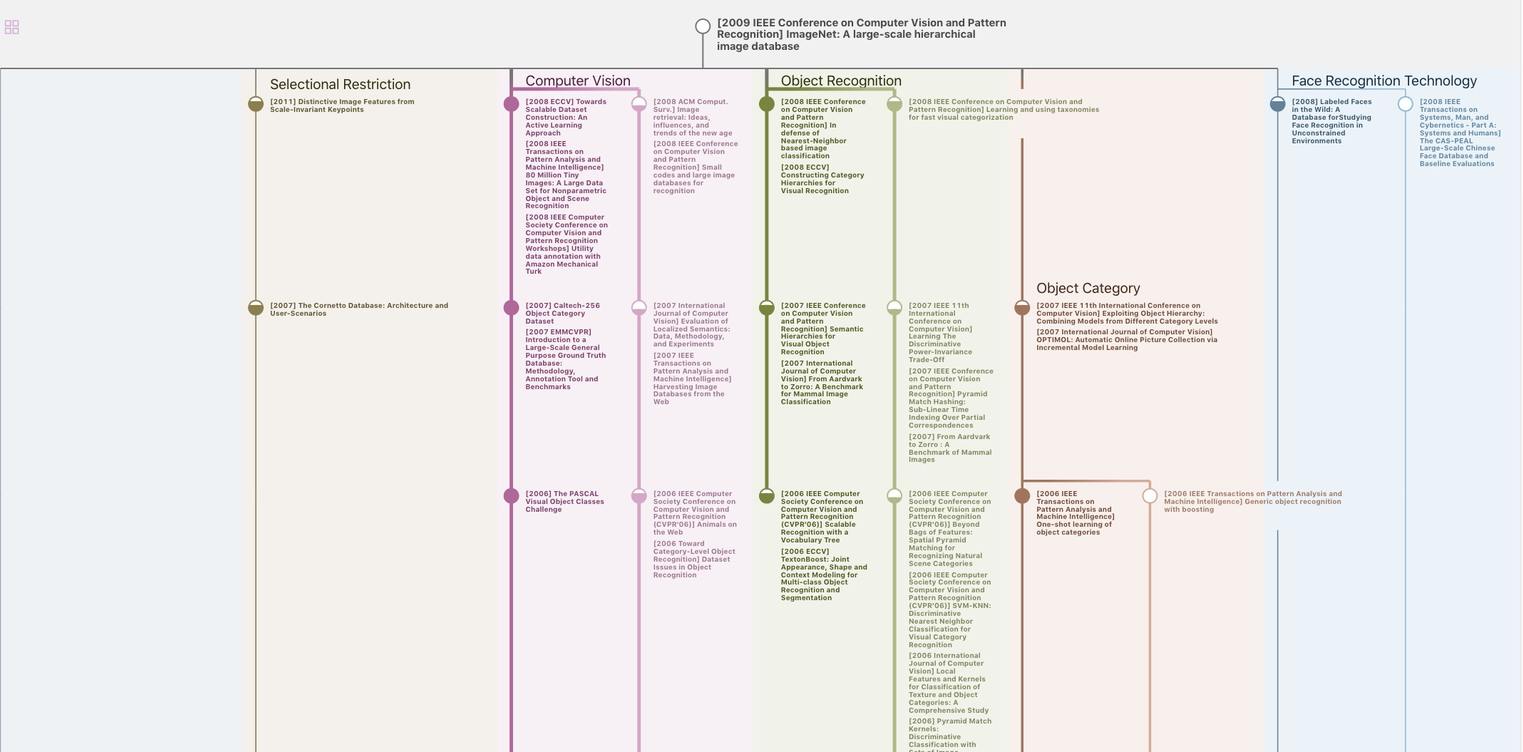
生成溯源树,研究论文发展脉络
Chat Paper
正在生成论文摘要