The Suitability of Different Training Images for Producing Low Connectivity, High Net:Gross Pixel-Based MPS Models
Springer Proceedings in Earth and Environmental SciencesGeostatistics Toronto 2021(2023)
摘要
AbstractPixel-based multiple-point statistical (MPS) modelling is an appealing geostatistical modelling technique as it easily honours well data and allows use of geologically-derived training images to reproduce the desired heterogeneity. A variety of different training image types are often proposed for use in MPS modelling, including object-based, surface-based and process-based models. The purpose of the training image is to provide a description of the geological heterogeneities including sand geometries, stacking patterns, facies distributions, depositional architecture and connectivity. It is, however, well known that pixel-based MPS modelling has difficulty reproducing facies connectivity, and this study investigates the performance of a widely-available industrial SNESIM algorithm at reproducing the connectivity in a geometrically-representative, idealized deep-water reservoir sequence, using different gridding strategies and training images. The findings indicate that irrespective of the sand connectivity represented in the training image, the MPS models have a percolation threshold that is the same as the well-established 27% percolation threshold of random object-based models. A more successful approach for generating poorly connected pixel-based MPS models at high net:gross ratios has been identified. In this workflow, a geometrical transformation is applied to the training image prior to modelling, and the inverse transformation is applied to the resultant MPS model. The transformation is controlled by a compression factor which defines how non-random the geological system is, in terms of its connectivity.
更多查看译文
关键词
different training images,low connectivity,pixel-based
AI 理解论文
溯源树
样例
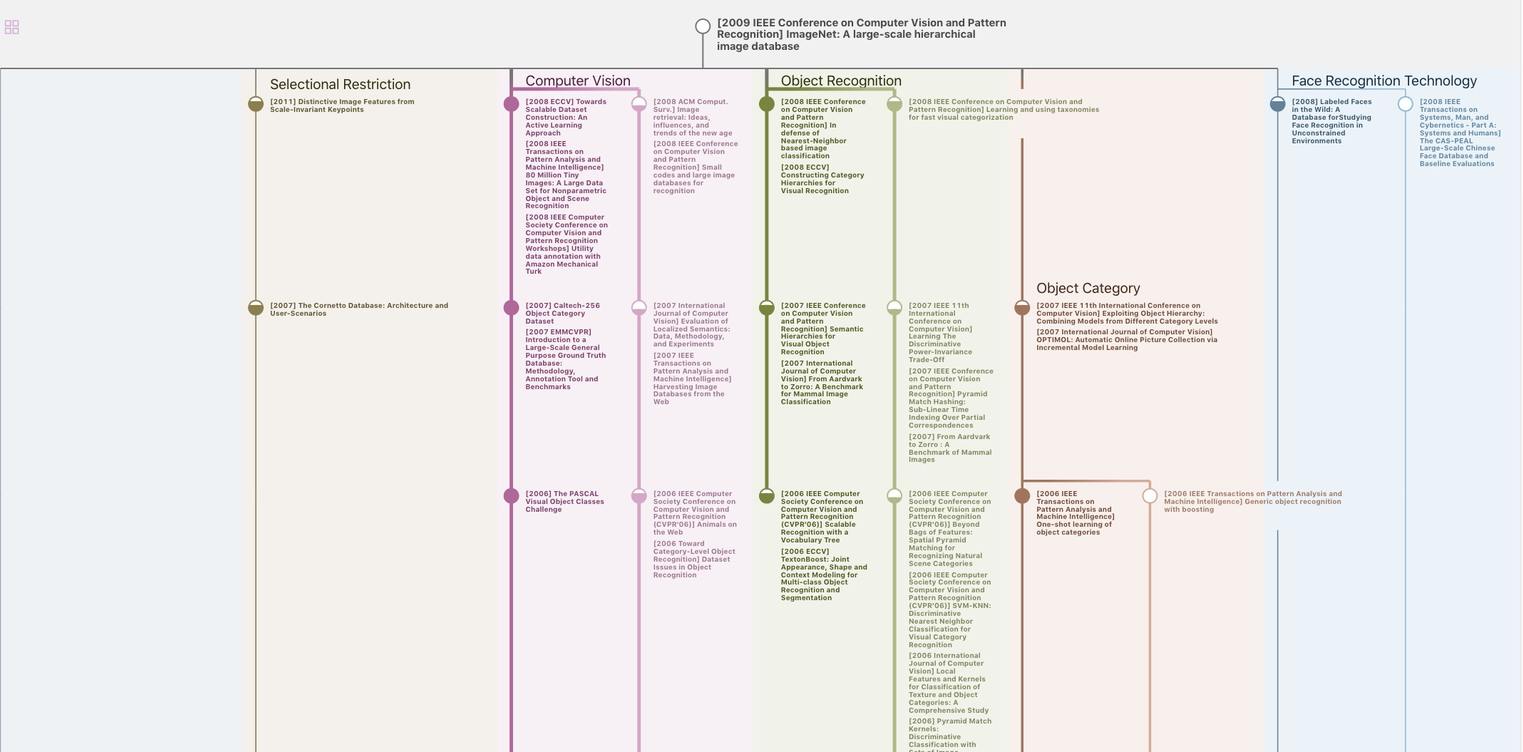
生成溯源树,研究论文发展脉络
Chat Paper
正在生成论文摘要