WPFSAD: Wind Power Forecasting System Integrating Dual-Stage Attention and Deep Learning
IEEE TRANSACTIONS ON INDUSTRIAL INFORMATICS(2023)
摘要
Wind power forecasting with high accuracy and stability concretely contributes to efficient scheduling and risk management of wind power systems. However, current studies remain limited due to these two shortcomings: 1) overemphasizing model combination strategy and data preprocessing, thus ignoring the improvement of the model ' s internal computing mechanism; and 2) much attention was paid to the single-stage attention mechanism, but little is known about dual-stage attention (DSA) mechanism. In this article, we propose a wind power forecasting system (WPFSAD), especially attaching importance to the improvement of forecasting precision and stability using a DSA-based mechanism. Specifically, the features of the input and hidden layers are adaptively and dynamically extracted in-depth. Wind power datasets from three wind farms are used to carry out simulation experiments to demonstrate the effectiveness of the WPFSAD. The study findings confirm the total supremacy of the WPFSAD in forecasting precision and stability, convergence, and robustness over its benchmarks.
更多查看译文
关键词
Forecasting,Wind power generation,Feature extraction,Predictive models,Adaptation models,Training,Wind energy,Attention mechanism,convergence and sensitivity analyses,deep learning,feature decomposition,wind power forecasting
AI 理解论文
溯源树
样例
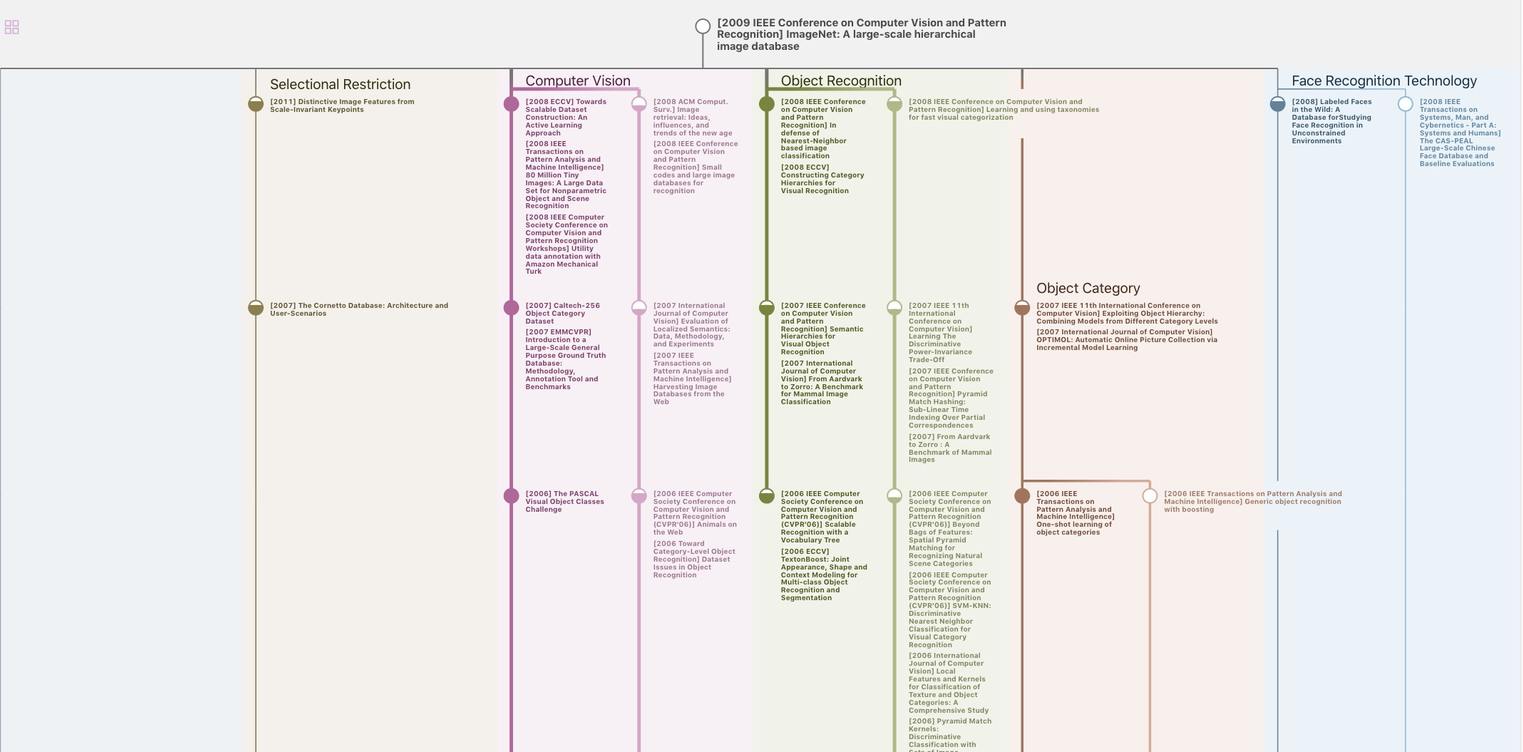
生成溯源树,研究论文发展脉络
Chat Paper
正在生成论文摘要