Patch-Mixing Contrastive Regularization for Few-Label Semi-Supervised Learning.
IEEE Transactions on Artificial Intelligence(2024)
摘要
Recently, consistency regularization has become a fundamental component in semi-supervised learning, which tries to make the network's predictions on unlabeled data to be invariant to perturbations. However, its performance decreases drastically when there are scarce labels, e.g., two labels per category. In this article, we analyze the semantic bias problem in consistency regularization for semi-supervised learning and find that this problem stems from imposing consistency regularization on some semantically biased positive sample pairs derived from indispensable data augmentation. Based on the above analysis, we propose a patch-mixing contrastive regularization approach called
$p$
-Mix for semi-supervised learning with scarce labels. In
$p$
-Mix, the magnitude of semantic bias is estimated by weighting augmented samples in the embedding space. Specifically, the samples are mixed in both sample space and embedding space, respectively, to construct more reliable and task-relevant positive sample pairs. Then, a patch-mixing contrastive objective is designed to indicate the magnitude of semantic bias by utilizing a mixed embedding weighted by virtual soft labels. Extensive experiments were conducted, demonstrating that
$p$
-Mix significantly outperforms current state-of-the-art approaches. Especially,
$p$
-Mix achieves an accuracy of 91.95% on the CIFAR-10 benchmark with only two labels available for each category, which exceeds the second-best method ICL-SSL by 3.22%.
更多查看译文
关键词
Deep semi-supervised learning,few-label,patch-mixing
AI 理解论文
溯源树
样例
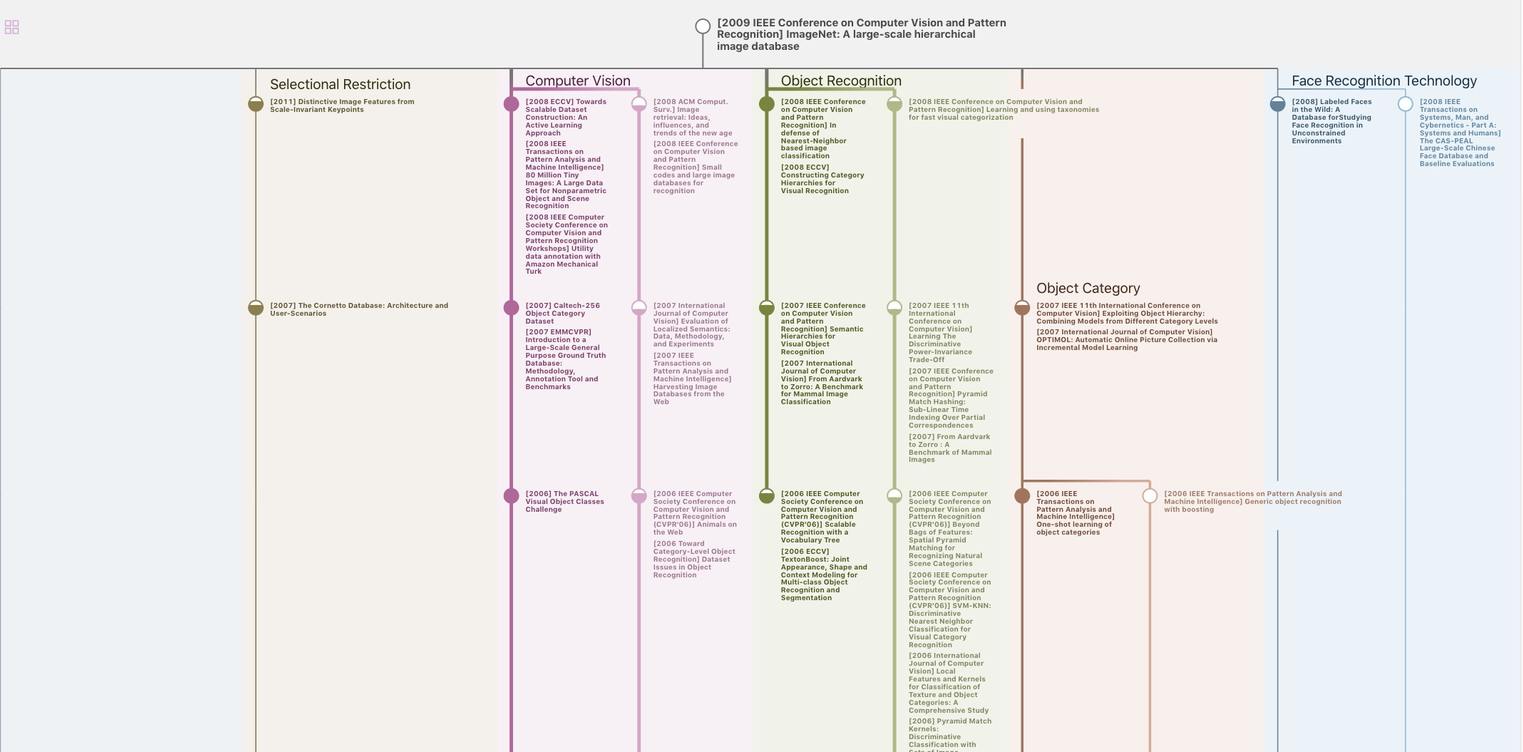
生成溯源树,研究论文发展脉络
Chat Paper
正在生成论文摘要