Dual Fusion-Propagation Graph Neural Network for Multi-View Clustering
IEEE TRANSACTIONS ON MULTIMEDIA(2023)
摘要
Deep multi-view representation learning focuses on training a unified low-dimensional representation for data with multiple sources or modalities. With the rapidly growing attention of graph neural networks, more and more researchers have introduced various graph models into multi-view learning. Although considerable achievements have been made, most existing methods usually propagate information in a single view and fuse multiple information only from the perspective of attributes or relationships. To solve the aforementioned problems, we propose an efficient model termed Dual Fusion-Propagation Graph Neural Network (DFP-GNN) and apply it to deep multi-view clustering tasks. The proposed method is designed with three submodules and has the following merits: a) The proposed view-specific and cross-view propagation modules can capture the consistency and complementarity information among multiple views; b) The designed fusion module performs multi-view information fusion with the attributes of nodes and the relationships among them simultaneously. Experiments on popular databases show that DFP-GNN achieves significant results compared with several state-of-the-art algorithms.
更多查看译文
关键词
Graph neural networks,Task analysis,Deep learning,Convolution,Clustering algorithms,Representation learning,Message passing,graph neural network,unsuper- vised learning,multi-view clustering
AI 理解论文
溯源树
样例
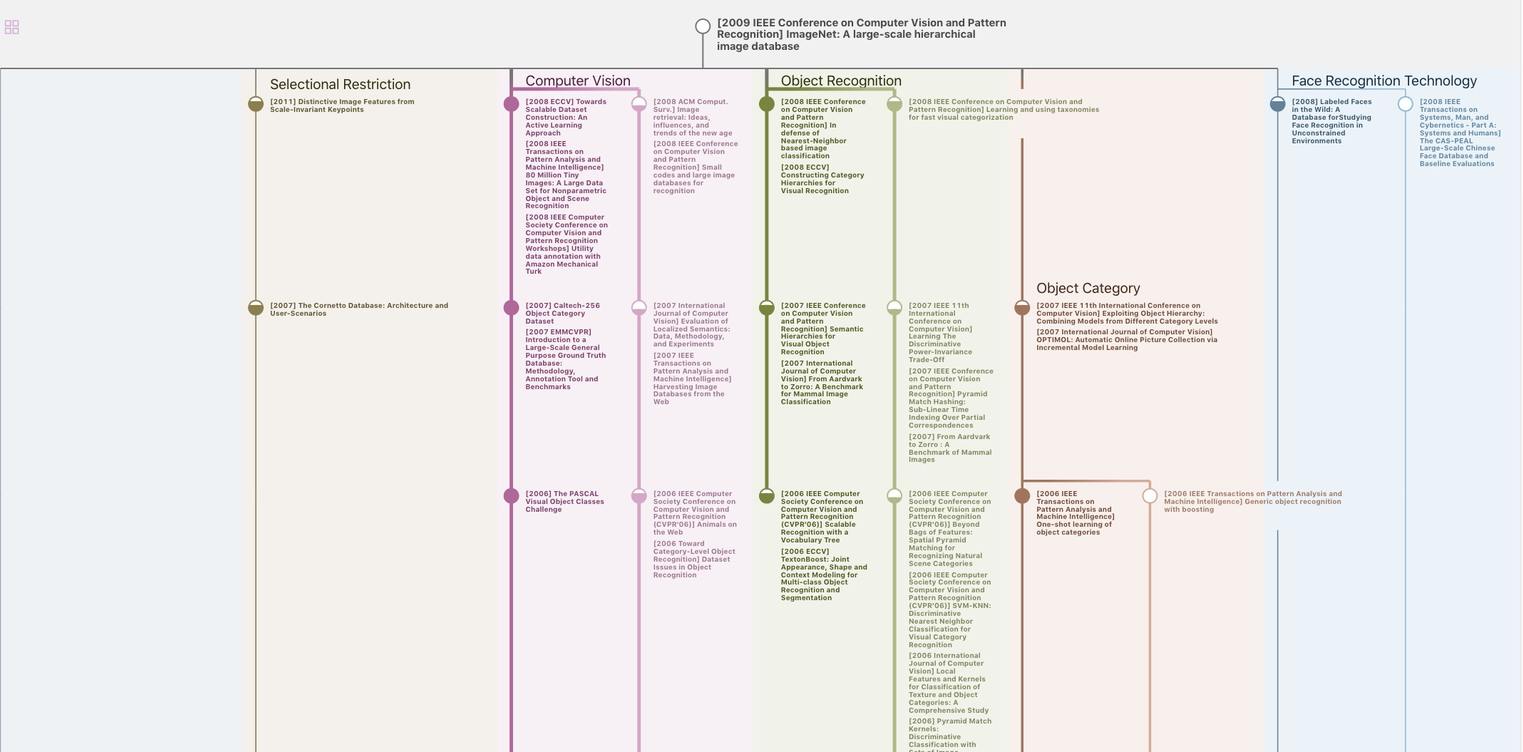
生成溯源树,研究论文发展脉络
Chat Paper
正在生成论文摘要