Deep-learning-based local wavefront attributes and their application to 3D prestack data enhancement
GEOPHYSICS(2023)
摘要
The work presents a novel workflow to accelerate the esti-mation of local wavefront attributes (LWAs) from massive 3D prestack seismic data using deep learning (DL) focusing on data enhancement. A standard estimation method based on a semblance-based brute-force optimization provides good results but is time consuming. A modification of the U-net con-volutional neural network, commonly used in image process-ing applications, is proposed to link the seismic data with the wavefront attributes. Color pixel image input for the neural network is generated through a straightforward seismic data regularization based on supergrouping followed by red, green, and blue encoding. The proposed workflow can be adapted to any 3D prestack seismic volume. Conventional semblance -based attributes estimation is required for the training step but only for approximately 1% of the total data. The prediction step is very efficient and reduces the overall run time signifi-cantly. The verification of the proposed approach is performed on challenging real land and marine data sets. As a result, DL -based estimation of LWAs accelerates computation up to 200 times compared to the standard method. The attributes from the proposed DL-based approach indicate an acceptable match compared with the brute-force semblance-based optimization results. Conventional and proposed estimation methods result in comparable prestack data enhancement results for more re-liable seismic processing in challenging areas.
更多查看译文
关键词
local wavefront attributes,3d,deep-learning-based
AI 理解论文
溯源树
样例
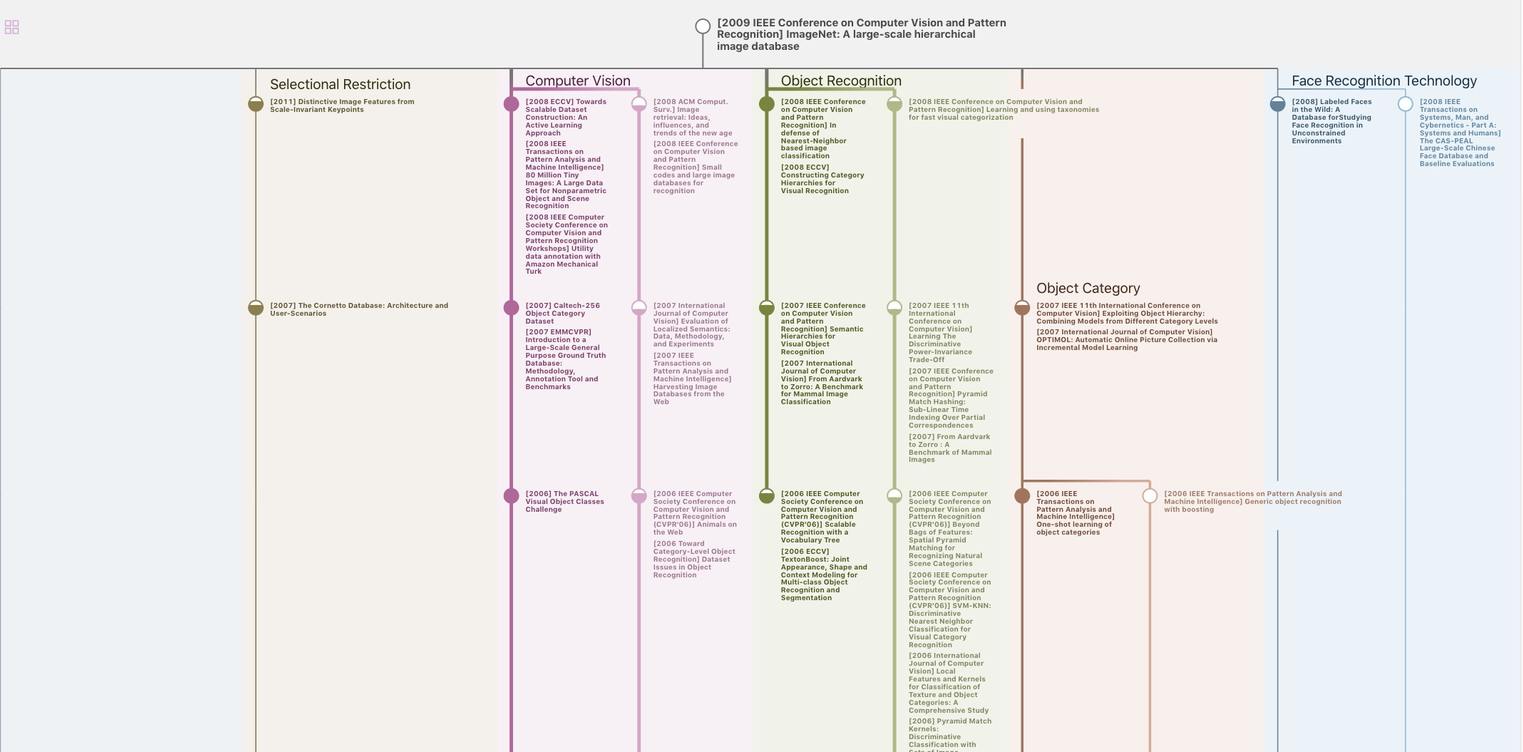
生成溯源树,研究论文发展脉络
Chat Paper
正在生成论文摘要