Online Smooth Backfitting for Generalized Additive Models
Journal of the American Statistical Association(2023)
摘要
We propose an online smoothing backfitting method for generalized additive models coupled with local linear estimation. The idea can be extended to general nonlinear optimization problems. The strategy is to use an appropriate-order expansion to approximate the nonlinear equations and store the coefficients as sufficient statistics which can be updated in an online manner by the dynamic candidate bandwidth method. We investigate the statistical and algorithmic convergences of the proposed method. By defining the relative statistical efficiency and computational cost, we further establish a framework to characterize the tradeoff between estimation performance and computation performance. Simulations and real data examples are provided to illustrate the proposed method and algorithm. for this article are available online.
更多查看译文
关键词
online smooth backfitting,generalized additive models
AI 理解论文
溯源树
样例
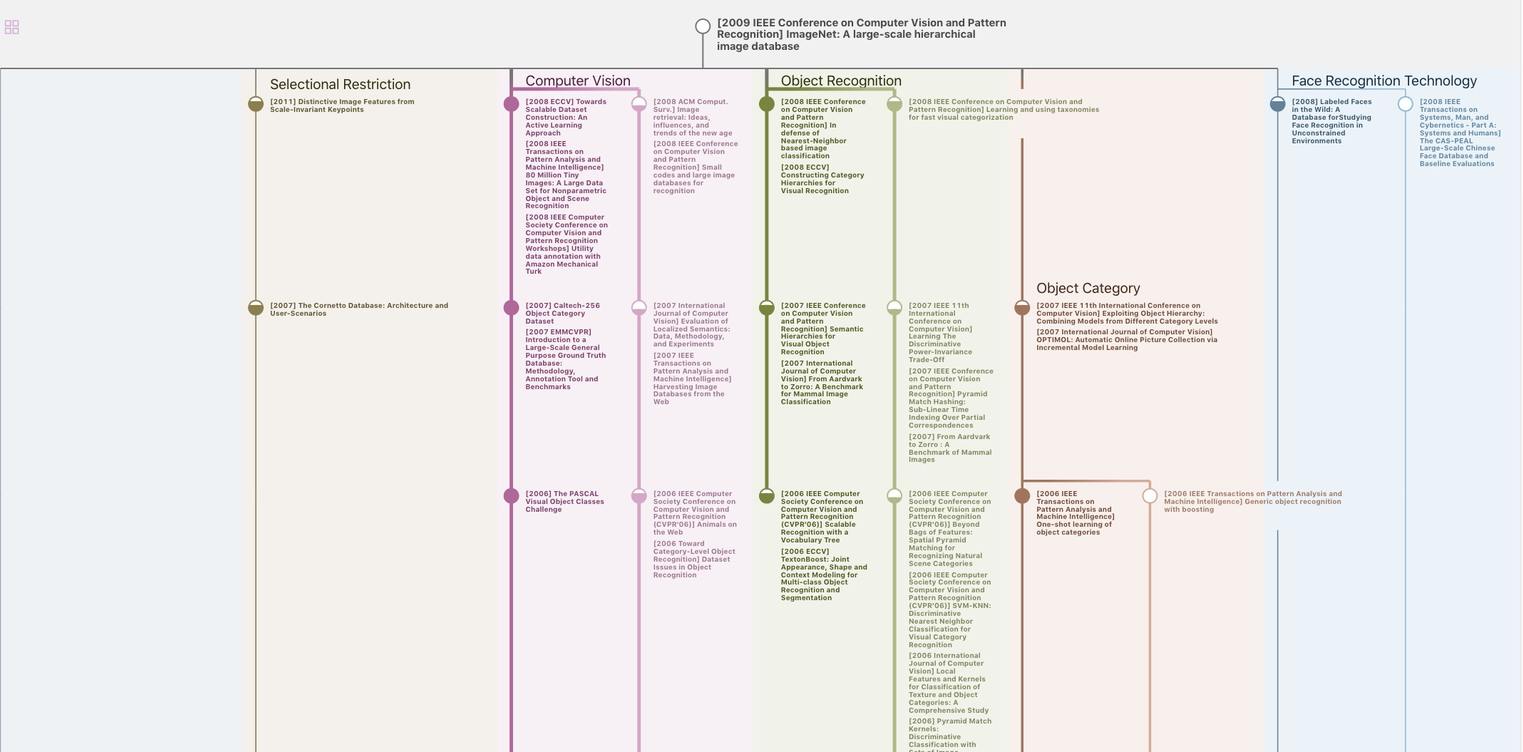
生成溯源树,研究论文发展脉络
Chat Paper
正在生成论文摘要