Multi-task Joint Learning to Enhance Named Entity Recognition
Journal of physics Conference series(2023)
摘要
Abstract Named Entity Recognition (NER) models have achieved good performance in recent years but also have some shortcomings. Existing models regard NER as a sequence labeling task for label prediction, without considering the impact of different stages in the entity recognition process on the final result. NER can be viewed as two separate subtasks: boundary detection task and type prediction task. The two subtasks can transmit information and cooperate in the process of entity recognition, so the synergy of the two subtasks is beneficial to NER. In this paper, we propose a method to split the NER task into multiple subtasks and use the information of each subtask to enhance the NER task. According to the characteristics of different subtasks, we use different feature extraction method models to extract structural information useful for this task effectively. Using the information extracted from each subtask or the final results of the subtasks, the performance of NER was enhanced through the gating network. We conducted extensive experiments on the CONLL2003 dataset, and the experimental results show that our proposed multi-task joint learning enhances the effectiveness of the named entity recognition model.
更多查看译文
AI 理解论文
溯源树
样例
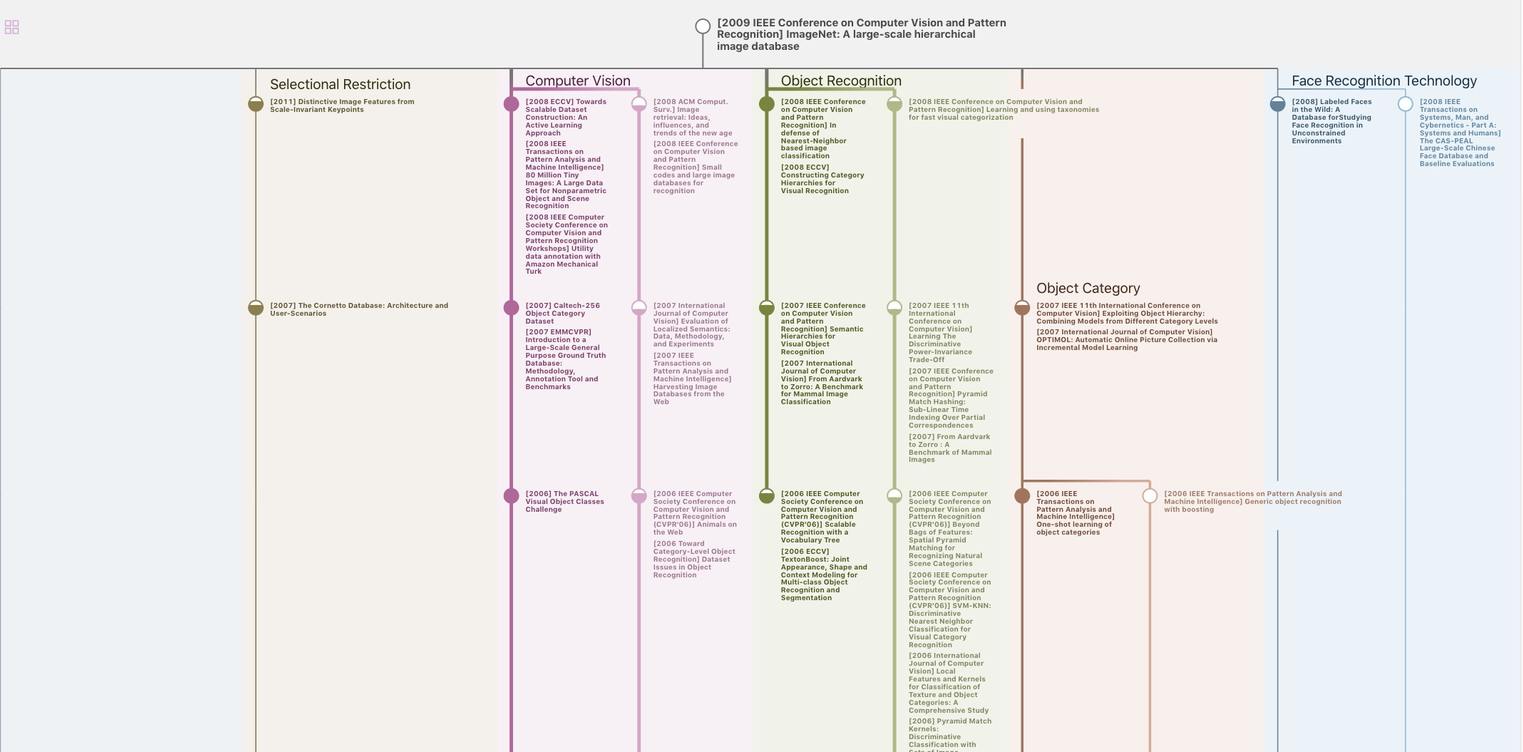
生成溯源树,研究论文发展脉络
Chat Paper
正在生成论文摘要