A Similarity-based Time Series Source Dataset Selection Method for Transfer Learning
Journal of Physics: Conference Series(2023)
摘要
Abstract Source datasets are selected with high similarity to target datasets to improve the effect of transfer learning. The low similarity between the source and target datasets may lead to negative transfer. This paper proposes a similarity-based time series source dataset selection method for transfer learning. First, to reduce the complexity of the operation, we use Dynamic time-warped barycentric averaging to obtain the prototype signals of each dataset and convert the dataset similarity calculation into the similarity calculation between the prototype signals. The dynamic time warping (DTW) algorithm, commonly used to calculate time-series signal similarity, does not consider the effect of signal complexity and the amount of data the dataset contains on the signal similarity calculation. This article makes improvements to the above issues. Experiments are carried out on 20-time series datasets archived by the University of California Riverside. Experimental results show that the average transfer effect of source datasets selected by the proposed method is better than the commonly used dataset similarity measurement method based on DTW.
更多查看译文
关键词
transfer learning,selection,similarity-based
AI 理解论文
溯源树
样例
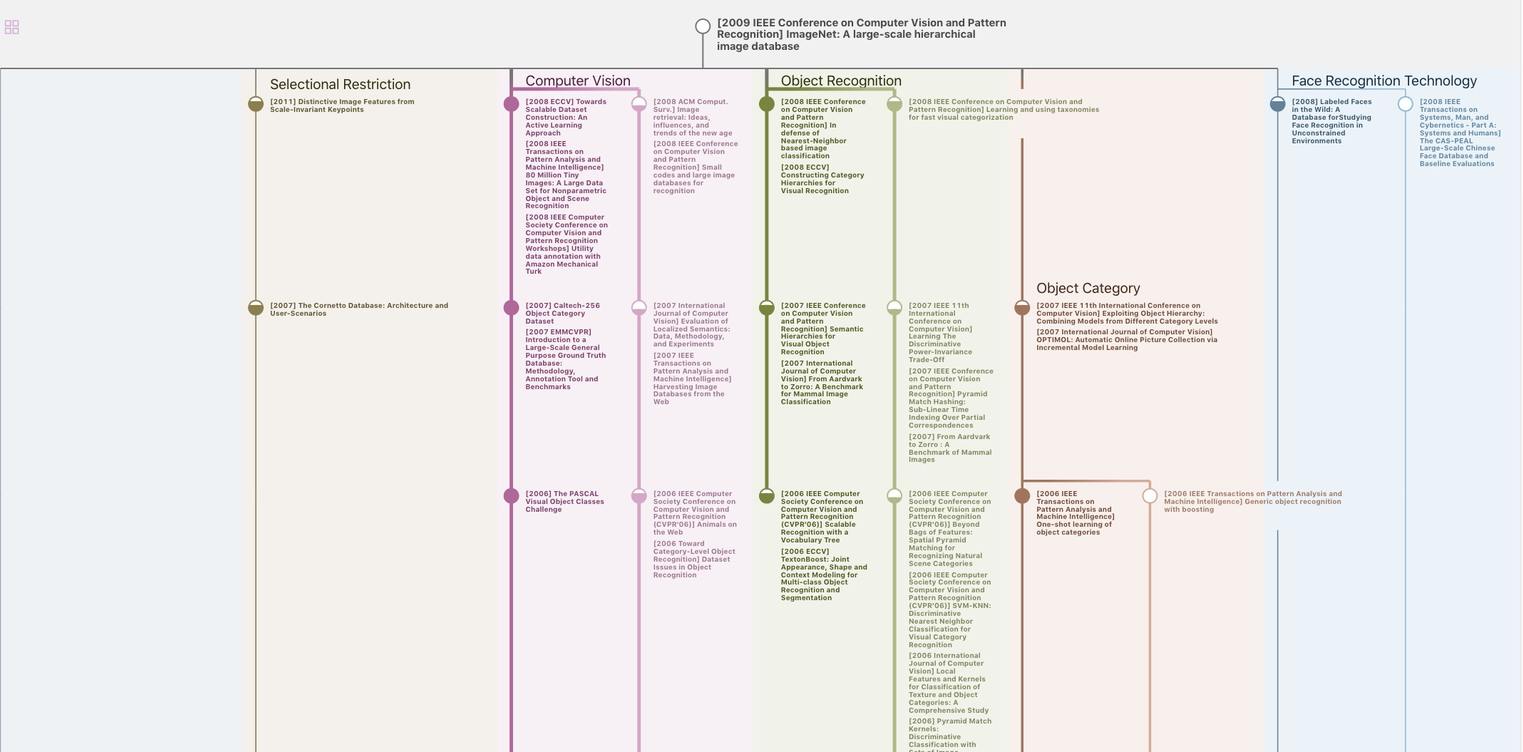
生成溯源树,研究论文发展脉络
Chat Paper
正在生成论文摘要