A Transformer Model for Ionospheric TEC Prediction Using GNSS Observations
crossref(2023)
摘要
<p>Precise modeling of the ionospheric Total Electron Content (TEC) is critical for reliable and accurate GNSS applications. TEC is the integral of the location-dependent electron density along the signal path and is a crucial parameter that is often used to describe ionospheric variability, as it is strongly affected by solar activity. TEC is highly depended on local time (temporal variability), latitude, longitude (spatial variability), solar and geomagnetic conditions. The propagation of the signals from GNSS (Global Navigation Satellite System) satellites throughout the ionosphere is strongly influenced by temporal changes and ionospheric regular or irregular variations. Here, we leverage transformer as an effective and scalable structure with self-attention mechanisms, for modeling long-range temporal dependencies for ionospheric TEC modelling based on GNSS data. </p> <p>The proposed transformer model is capable of learning long-range temporal dependencies. In seq2seq models, learning temporal dependencies is a demanding task, and often the model forgets the first part, once it completes processing the whole sequence input. Our model utilizes attention mechanisms and identifies complex dependencies between input sequence elements throughout the whole sequence.</p> <p>Our model handles imbalanced datasets. Our work demonstrates that combining the unsupervised pre-training process with downstream task fine-tuning, offers a practical solution for ionospheric TEC modelling. This is a comparative advantage against the existing state-of-the-art works which, in most cases, fail to sufficiently model intense ionospheric variability conditions.</p>
更多查看译文
AI 理解论文
溯源树
样例
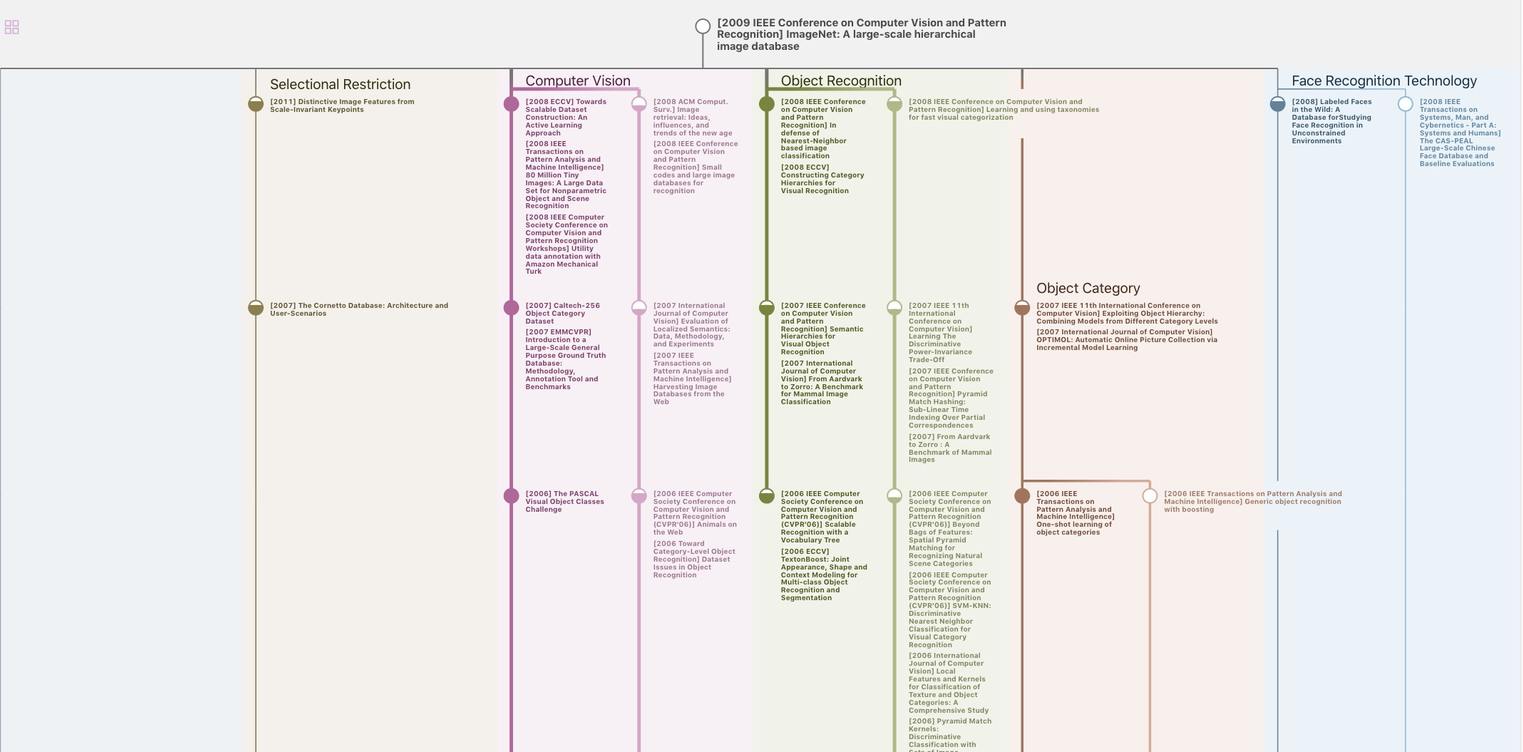
生成溯源树,研究论文发展脉络
Chat Paper
正在生成论文摘要