Image Super-Resolution With Content-Aware Feature Processing.
IEEE Transactions on Artificial Intelligence(2024)
摘要
Image super-resolution (SR) is currently a very active research topic with applications spanning from computer vision to videos and graphic industries. The top performers in SR field usually employ deep or wide convolutional neural networks (CNNs) to restore the lost textures from low-resolution images. However, most of these methods adopt pixel shuffle and deconvolution as their upsampling techniques and often generate conspicuous artifacts in the reconstructed image. In addition, the ongoing trend of directly portraying the degraded low-resolution image to a high-resolution image via complex deep CNNs improves the reconstruction performance, but at the cost of high computational complexity. In this article, we propose a multilevel bicubic upsampler network for reconstructing high-quality SR image with a restricted number of parameters. A novel content-aware feature difference (CAFD) block is presented to reform the network by focusing on contextual information. The proposed CAFD block consists of four multilevel attention blocks for a better extraction of low-level features at different scales. Furthermore, we design an innovative upsampling layer that consistently outperforms the traditional upsampling methods. These components collaboratively endow our proposed network with a great performance boost, helping it achieve state-of-the-art accuracy on five synthetic benchmark datasets, both qualitatively and quantitatively. In addition, a detailed ablation study has been accomplished to scrutinize the improvements obtained by different modules in the proposed method.
更多查看译文
关键词
Bicubic upsampling,content-aware feature difference (CAFD) block,convolutional neural networks (CNNs),multilevel attention (MLA) block,super-resolution (SR)
AI 理解论文
溯源树
样例
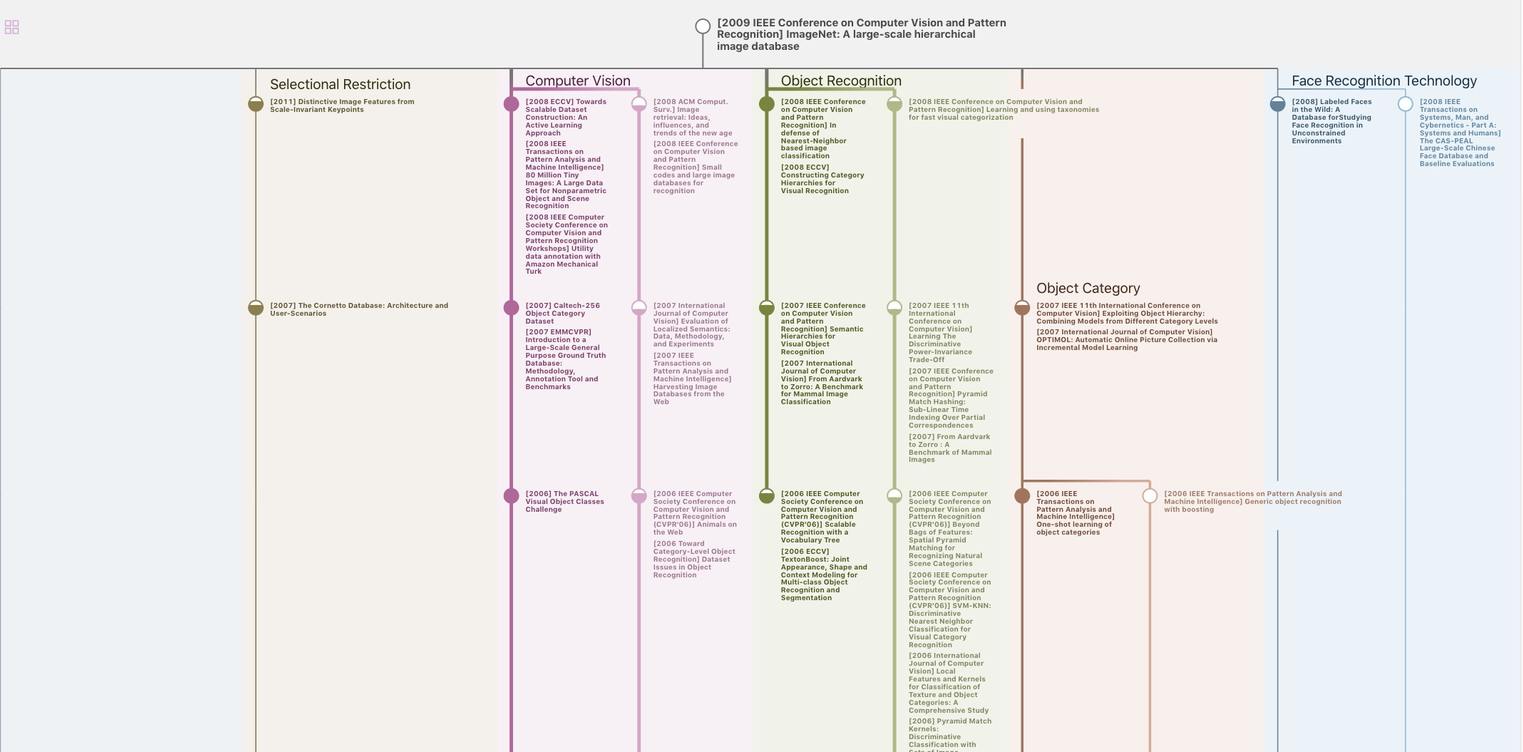
生成溯源树,研究论文发展脉络
Chat Paper
正在生成论文摘要